Enhancing Zero-shot Personalized Image Generation with Masked Cross-Attention
- Innovative Masked Cross-Attention Mechanism: InstantFamily introduces a novel masked cross-attention mechanism that integrates with a multimodal embedding stack, allowing for precise control and integration of multiple identities (IDs) within a single generated image.
- Superior ID Preservation: The method leverages features from a pre-trained face recognition model combined with textual conditions to preserve the identity and characteristics of multiple subjects in zero-shot scenarios, setting a new benchmark in multi-ID image generation.
- Scalability and Performance: Demonstrates exceptional scalability and robust performance, surpassing existing models in both single-ID and multi-ID preservation, even with a greater number of IDs than it was originally trained to handle.
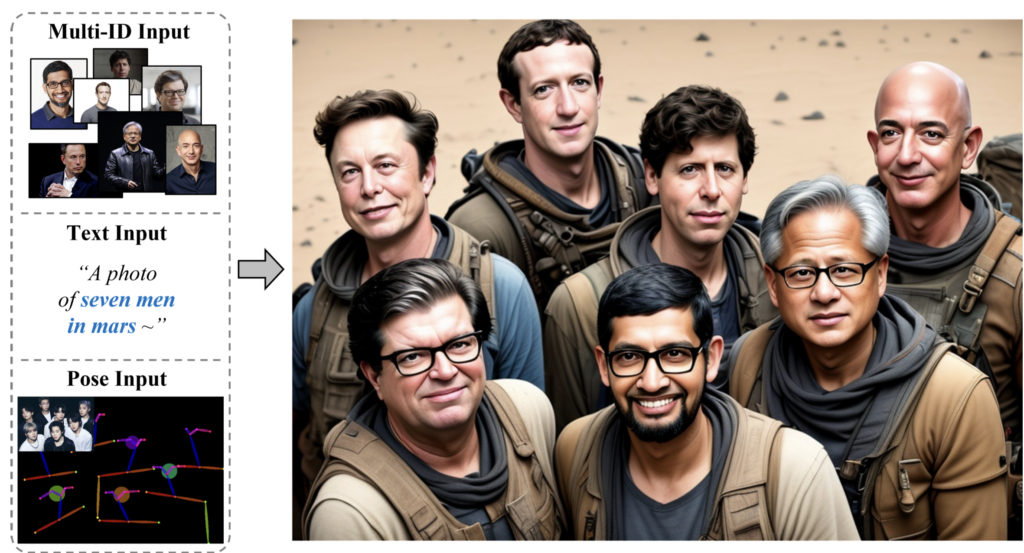
InstantFamily marks a significant milestone in the realm of personalized image generation. Traditional methods often struggle with creating cohesive images that integrate multiple identities or concepts effectively. InstantFamily addresses this by employing a masked cross-attention mechanism that allows for the detailed and accurate layering of multiple IDs in a single image, ensuring each is distinct yet harmoniously integrated.
The core of InstantFamily’s effectiveness lies in its use of a multimodal embedding stack that integrates global and local features extracted from a pre-trained face recognition model. This setup is further enriched with textual conditions that guide the image generation process, ensuring that each identity is represented as intended by the input prompts.
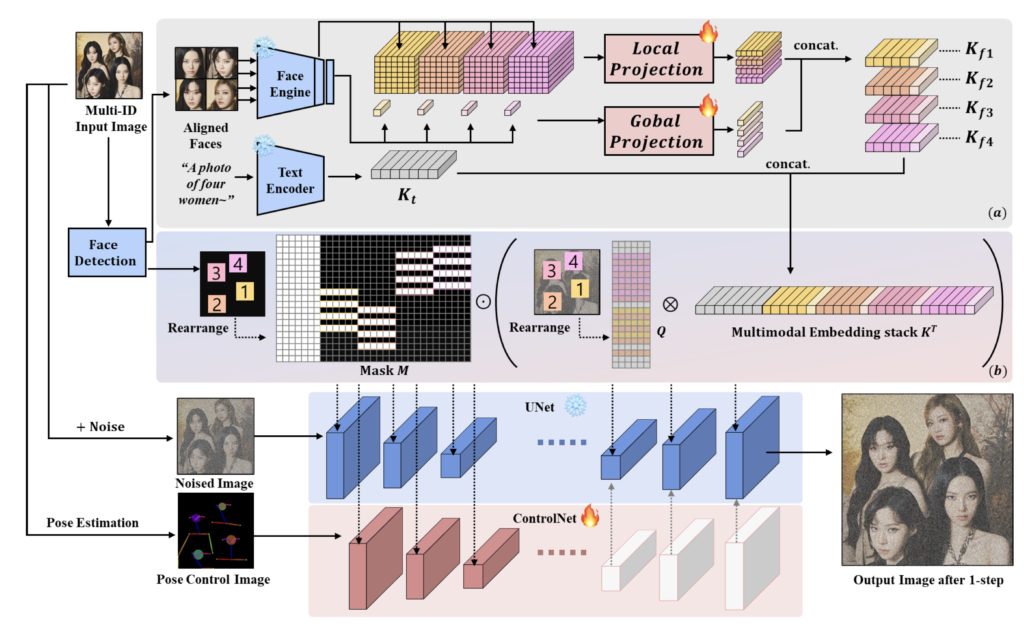
The technical foundation of InstantFamily involves the integration of advanced components like LoRA adapters and DreamBooth, which allow for high customization without the need for extensive retraining of the base model, thereby avoiding potential distribution shifts often seen with other methods. The training was conducted on high-performance NVIDIA A100 GPUs, showcasing the model’s ability to handle complex and resource-intensive tasks efficiently.
InstantFamily not only sets new standards in ID preservation but also introduces a novel metric for evaluating the preservation of multiple IDs in generated images. This metric has confirmed that InstantFamily achieves state-of-the-art performance in this domain.
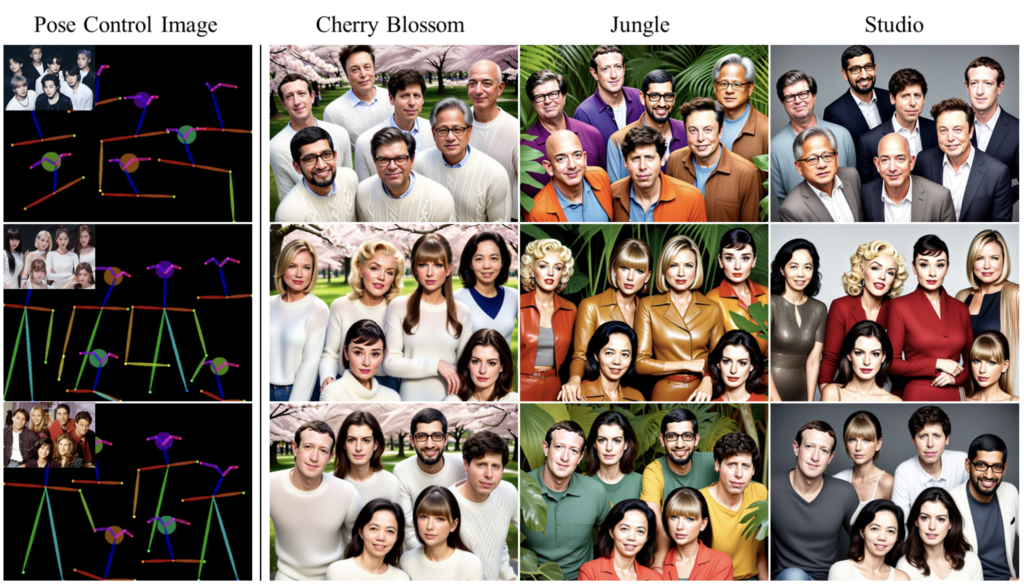
Moreover, InstantFamily’s ability to scale and handle more IDs than it was initially trained for opens new possibilities for generating multimedia content that can accommodate complex, multi-character scenarios without losing individual identity fidelity. This makes it particularly valuable for applications in digital marketing, entertainment, and personalized content creation, where bespoke image generation can significantly enhance user engagement and content relevance.
As AI continues to evolve, tools like InstantFamily are poised to revolutionize how we create and interact with digital content, making personalized and contextually relevant media more accessible and effective. The ongoing development of this technology is expected to lead to even more sophisticated applications, potentially transforming the landscape of digital media production.
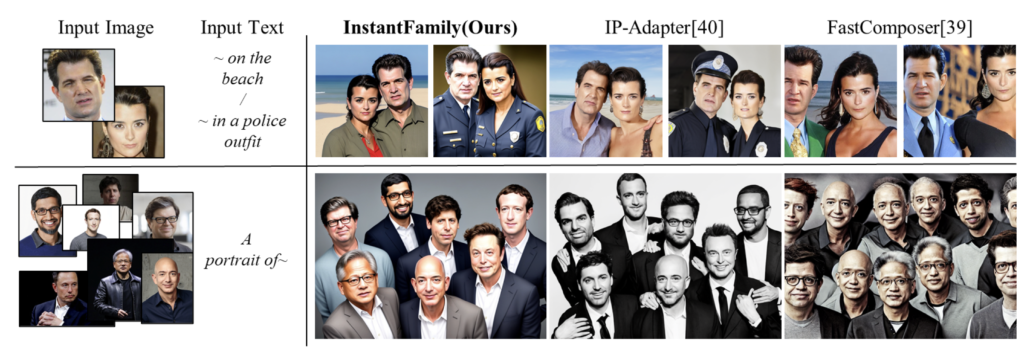