A Breakthrough in Point Cloud Diffusion Models Enables Scalable and High-Fidelity 3D Generation
- Resolution-Invariant Architecture: PointInfinity introduces a transformative approach with its fixed-size, resolution-invariant latent representation, allowing for the efficient generation of high-resolution point clouds from low-resolution training data.
- High-Resolution Generation: The model sets a new benchmark in 3D modeling by producing point clouds of up to 131k points, significantly surpassing previous models in both scale and quality.
- Quality Improvement with Scale: Unique to PointInfinity, the quality of the generated surfaces improves as the resolution increases, a phenomenon thoroughly analyzed and linked to the model’s innovative two-stream design.
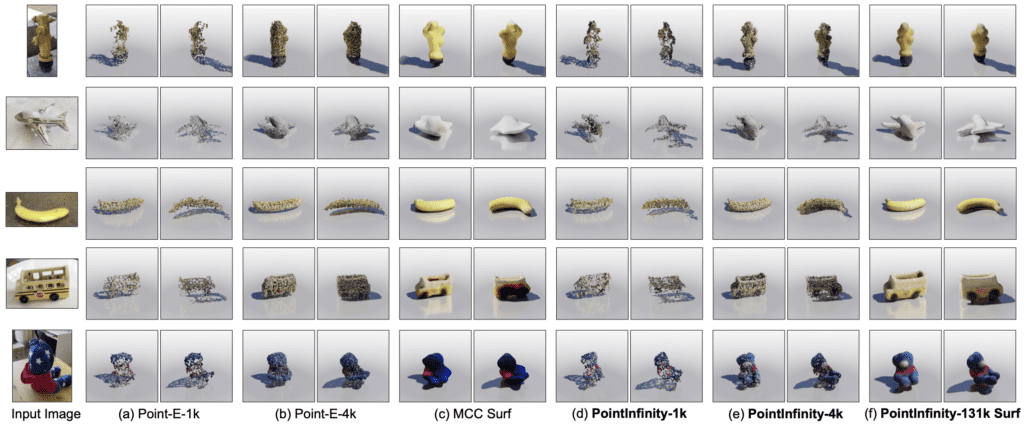
The advent of PointInfinity marks a significant leap forward in the field of 3D modeling, introducing a novel family of point cloud diffusion models that defy traditional resolution constraints. At the heart of PointInfinity lies a transformer-based architecture that maintains a fixed-size, resolution-invariant latent representation, a feature that dramatically enhances the model’s efficiency and scalability.
This groundbreaking approach enables PointInfinity to be trained on low-resolution point clouds without compromising its ability to generate high-resolution outputs during inference. This capability not only conserves computational resources during the training phase but also opens up new possibilities for creating detailed and intricate 3D models that were previously unattainable.
PointInfinity’s prowess is showcased in its ability to generate point clouds with up to 131,000 points, offering a 31-fold increase in resolution compared to its predecessors, such as Point-E. This remarkable feat is achieved through a two-stream design that separates the modeling of the underlying shape from the variable-sized point cloud representation. This decoupling is pivotal in allowing the model to scale its output resolution while maintaining, and even enhancing, the fidelity of the generated surfaces.
The model’s capacity to improve surface quality with increased resolution is not only intriguing but also indicative of a deeper underlying mechanism. This phenomenon is thoughtfully analyzed in the context of PointInfinity, drawing parallels to the concept of classifier-free guidance commonly observed in diffusion models. This analysis sheds light on how PointInfinity can effectively balance fidelity and variability during inference, offering insights that could propel future research in scalable 3D point cloud generation.
PointInfinity stands as a beacon for future developments in 3D modeling, offering a scalable and efficient solution for generating high-fidelity point clouds. Its introduction challenges the status quo and sets a new standard for what is achievable in the realm of 3D content creation, promising to fuel advancements across various applications, from virtual reality to architectural design. As PointInfinity continues to evolve, its impact on the field is poised to be profound, paving the way for a new era of 3D modeling that is bound only by the limits of imagination.