Enhancing Text-to-Audio Translations via Direct Preference Optimization
- troduction of Preference Optimization: Tango 2 utilizes a novel approach in the realm of text-to-audio generation by employing direct preference optimization (DPO), which refines audio output based on user-preferred examples.
- Creation of a Specialized Dataset: The development includes the synthesis of a preference dataset named Audio-alpaca, featuring audio outputs that are directly compared to ensure alignment with the text prompts’ intended content and sequence.
- Significant Performance Improvements: Preliminary results show that Tango 2 surpasses its predecessor and other leading models in both objective measurements and human evaluations, indicating a notable advancement in audio fidelity and relevance.
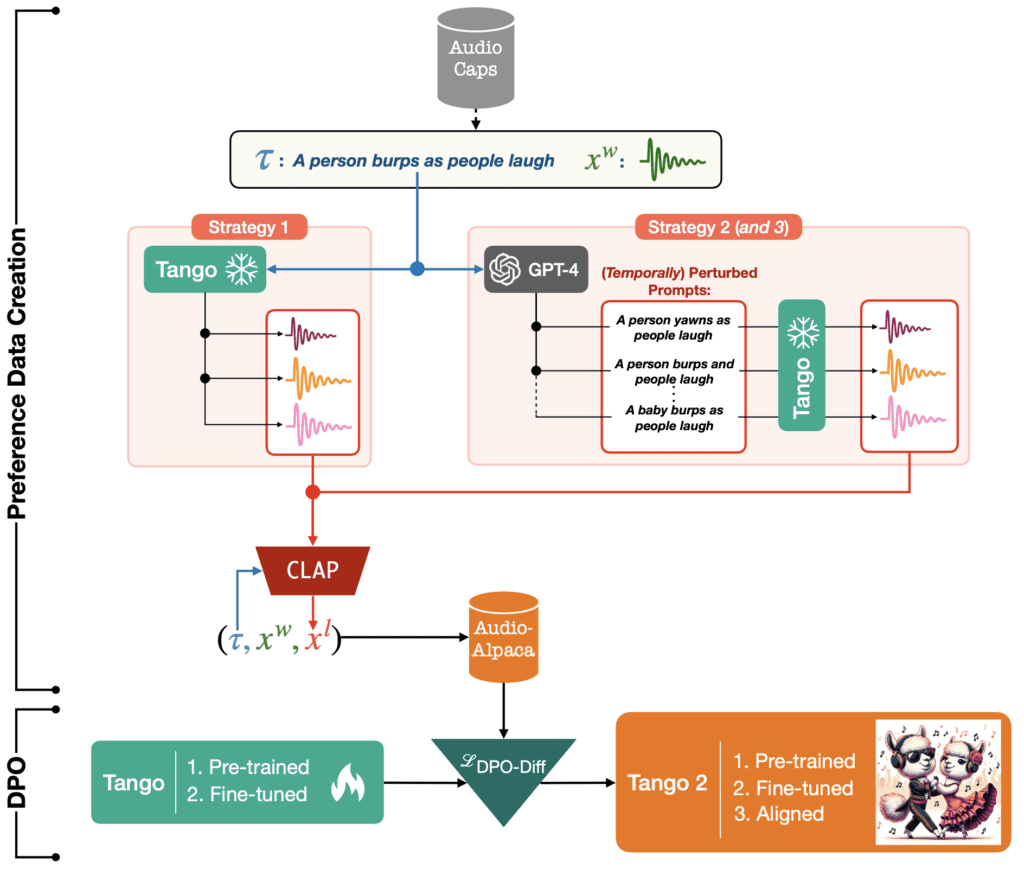
The latest development from the generative AI sector comes with the introduction of Tango 2, a pioneering text-to-audio model that significantly enhances the process of generating audio content from textual descriptions. This model represents a critical evolution in multimedia content creation, particularly benefiting industries like music and film where rapid prototyping of soundscapes is essential.
Core Innovations and Methodology
Tango 2’s core innovation lies in its use of direct preference optimization (DPO), a technique designed to fine-tune audio outputs based on a set of preferred examples versus less desirable ones. This method allows Tango 2 to learn more effectively from its mistakes by understanding not just what makes a good audio clip, but also what makes a bad one.
The model operates on a newly created dataset called Audio-alpaca, which consists of audio descriptions paired with corresponding audio files that are labeled as ‘winner’ or ‘loser’ based on their fidelity to the text prompt. These labels are determined through an adversarial filtering process, where audio outputs are generated and then scored; those with lower scores are used as examples of what to avoid.
Technical Breakthroughs and User Impact
By focusing on the alignment of audio content with the narrative and temporal sequence described in text prompts, Tango 2 addresses a common shortfall in previous models which often produced outputs that were mismatched or out of order. The preference-driven approach not only enhances the model’s accuracy but also its applicability in real-world scenarios where the context and sequence of sounds are crucial.
Future Prospects and Challenges
While Tango 2 has shown promising results, its development also opens discussions on the scalability of such models and the ethical implications of generating media content through AI. Future enhancements could include expanding the model’s capacity to handle more complex narratives and exploring its potential in other areas such as virtual reality and interactive gaming.
With Tango 2, the landscape of generative AI takes a significant leap forward. This model’s ability to fine-tune audio generation through direct preference optimization offers a glimpse into the future of AI-driven content creation, where accuracy and user preference drive the development of more dynamic and contextually aware AI systems.