InternVL 1.5 Challenges Proprietary Giants with Enhanced Multimodal Capabilities
- Enhanced Vision Encoder: InternVL 1.5 incorporates a robust vision foundation model, InternViT-6B, improved through continuous learning to enhance visual understanding, applicable across various language models.
- Dynamic High-Resolution Processing: The model supports high-resolution inputs by dynamically adjusting image tiles based on the aspect ratio, enabling processing of images up to 4K resolution.
- Bilingual Dataset Utilization: A meticulously curated high-quality bilingual dataset significantly boosts the model’s performance, especially in OCR and Chinese language tasks.
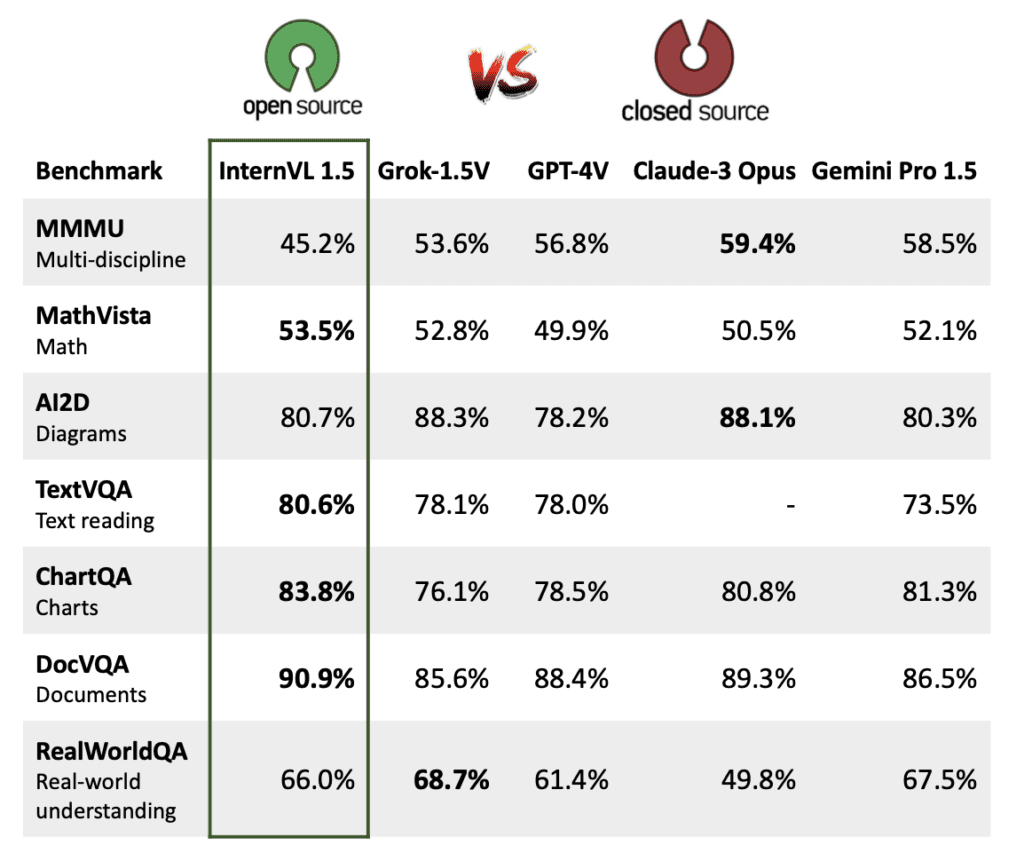
As multimodal models become increasingly crucial in tech, the open-source community is making significant strides to close the gap with commercial proprietary systems. InternVL 1.5, developed as part of this effort, represents a pivotal advancement in open-source multimodal large language models (MLLMs). This model not only matches but in some cases surpasses the capabilities of its commercial counterparts. Here’s a closer look at the innovations and their implications:
Strong Vision Encoder
InternVL 1.5’s vision capabilities are powered by InternViT-6B, a large-scale vision foundation model enhanced through a continuous learning strategy. This approach not only improves the model’s understanding of complex visual inputs but also ensures its adaptability across different linguistic frameworks, enhancing its utility in diverse applications.
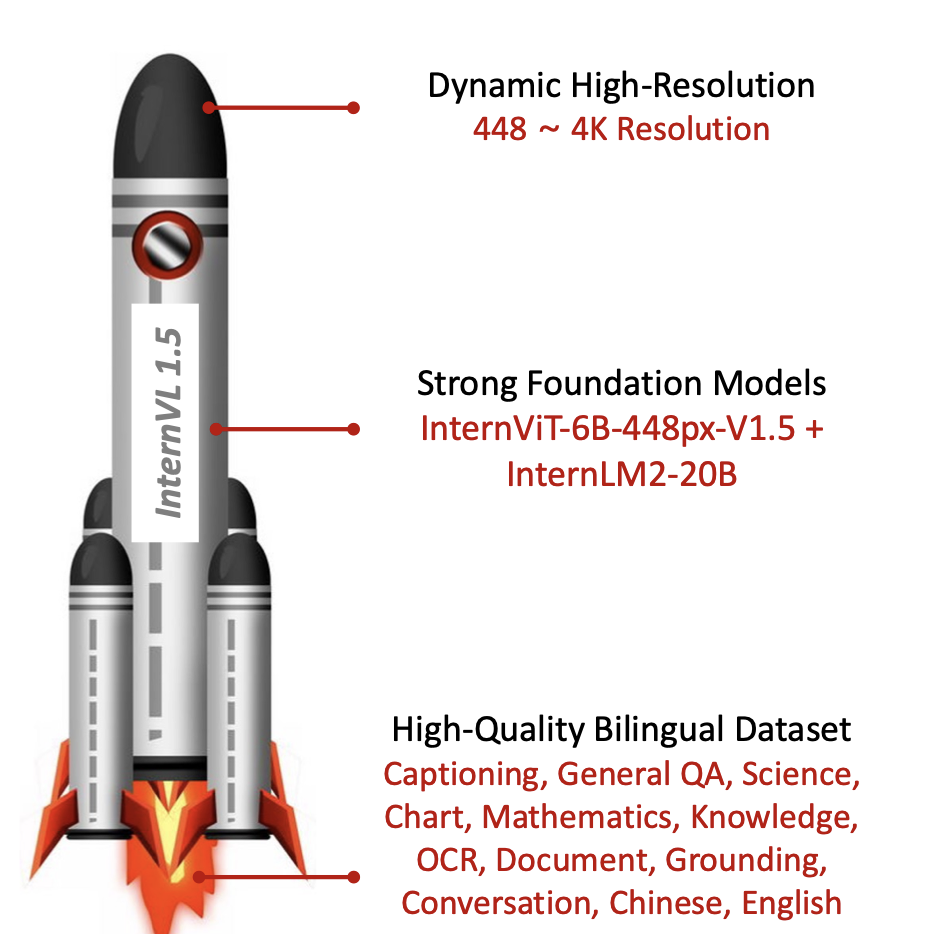
Dynamic High-Resolution Support
Addressing the need for high-quality image processing, InternVL 1.5 introduces a dynamic tiling mechanism that adjusts the segmentation of images based on their resolution and aspect ratio. This feature is crucial for maintaining image integrity, particularly when handling high-resolution inputs up to 4K, making the model ideal for detailed visual tasks.
High-Quality Bilingual Dataset
One of the standout features of InternVL 1.5 is its use of a high-quality bilingual dataset, which includes annotations in both English and Chinese. This dataset significantly enhances the model’s performance in OCR tasks and improves its understanding of scenes involving Chinese text, thereby broadening its applicability in global contexts.
Comparative Performance
InternVL 1.5 has been rigorously tested against both open-source and proprietary models across various benchmarks. It shows competitive or superior performance in many of these, particularly excelling in OCR and tasks requiring nuanced understanding of Chinese contexts. Such achievements underscore the potential of open-source initiatives in pushing the boundaries of what multimodal models can achieve.
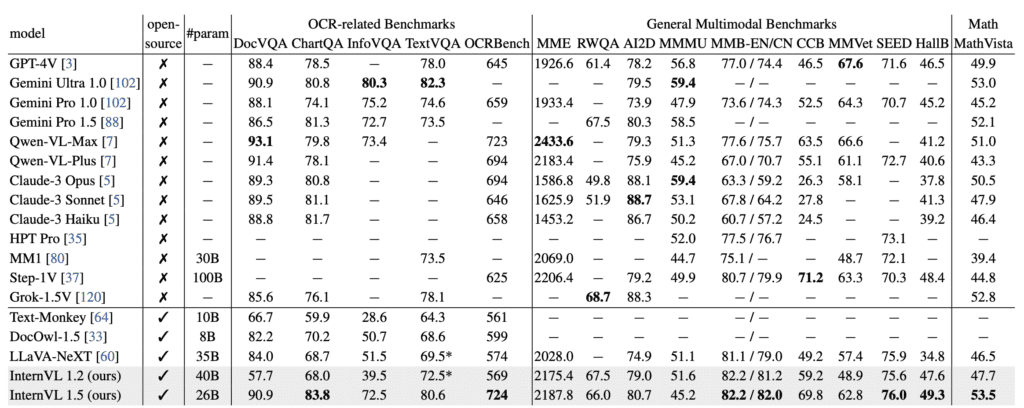
Future Directions and Challenges
While InternVL 1.5 marks a significant achievement, the landscape of multimodal understanding continues to evolve. The model’s developers aim to further enhance its capabilities and encourage collaboration within the global research community. Such efforts are expected to drive further innovations in open-source multimodal AI, challenging the current dominance of proprietary models and fostering a more inclusive and diverse AI development ecosystem.
InternVL 1.5 represents a major step forward in narrowing the performance gap between open-source and proprietary multimodal AI models. With its advanced visual encoding, support for high-resolution images, and enhanced bilingual capabilities, InternVL 1.5 is poised to become a key player in the field of AI, offering robust, accessible tools that empower researchers and developers around the world.