New Metrics, Nuanced Human Ratings, and Diverse Model Assessments
- Introduction of Gecko2K Benchmark: Google’s new Gecko2K benchmark categorizes prompts into sub-skills, providing a granular assessment of text-to-image (T2I) model capabilities and identifying specific complexities within tasks.
- Extensive Human Ratings Collection: Over 100,000 annotations were gathered across multiple templates and models, offering insights into the impacts of prompt ambiguity and variations in model performance.
- Innovative QA-Based Metric: A new QA-based evaluation metric aligns more closely with human judgments than existing metrics, enhancing the reliability of model comparisons across different conditions.
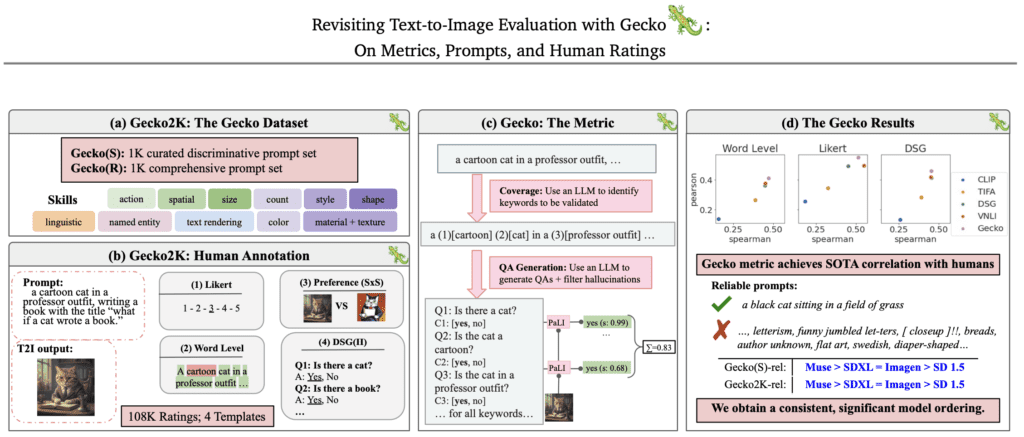
The rapid advancement of text-to-image (T2I) generative models necessitates robust and transparent evaluation methods to ensure they perform effectively and ethically. Google’s latest research initiative, introduces critical advancements in how these models are assessed, addressing longstanding issues with the alignment between generated images and textual prompts.
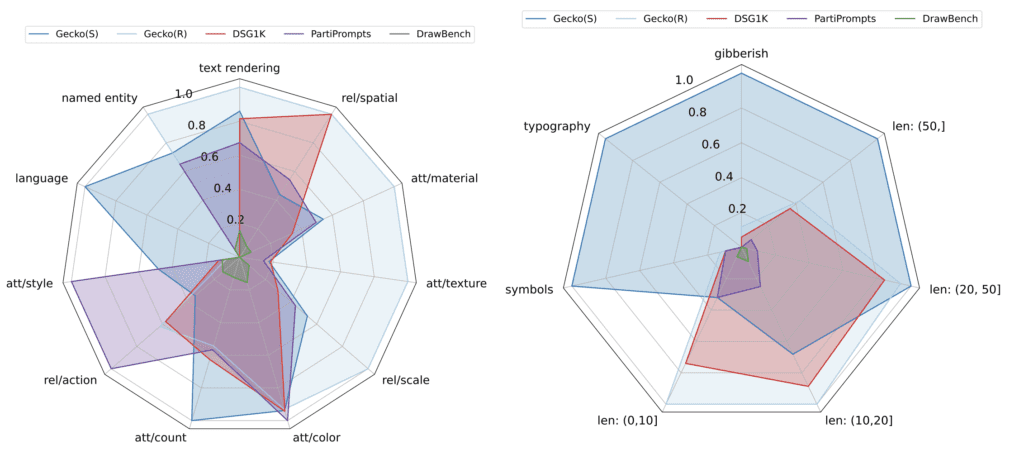
Deep Dive into Innovations
- Gecko2K Benchmark: This new benchmark is a significant leap in evaluating T2I models, breaking down tasks into sub-skills that clarify at what complexity levels these models begin to struggle. This allows developers to pinpoint specific areas for improvement within AI models, making the benchmark a powerful tool for refining AI capabilities.
- Human Annotations: By collecting a vast dataset of human annotations, the research provides a nuanced view of where T2I models succeed and fail. This large-scale analysis across four different templates and models sheds light on the subjective nature of human evaluations and the inherent ambiguities present in textual prompts.
- QA-Based Metric: The introduction of a QA-based auto-eval metric represents a methodological advancement, showing superior correlation with human ratings compared to older metrics. This approach potentially sets a new standard for model evaluation, prioritizing metrics that reflect human perceptions and understandings.
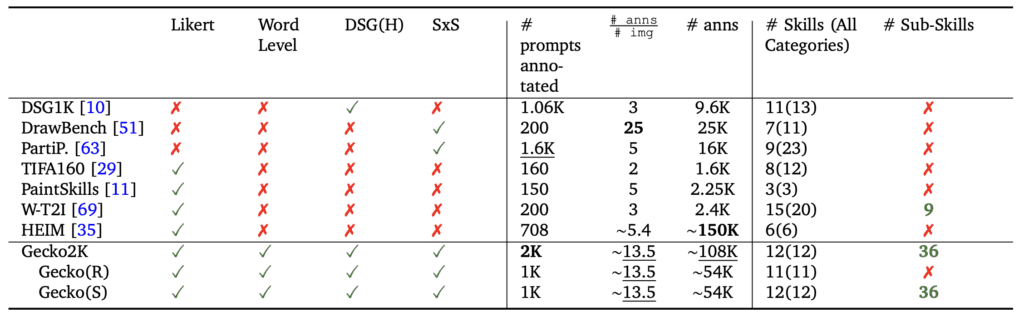
Strategic Implications and Future Work
The study highlights the necessity for ongoing refinement of both the models and the metrics used to evaluate them. The findings suggest that while current models are advancing, there remains a significant gap in their ability to handle complex, multi-faceted tasks as humans do. Future research could explore extending these evaluation frameworks to other forms of generative AI, potentially offering a comprehensive standard that spans various AI disciplines.
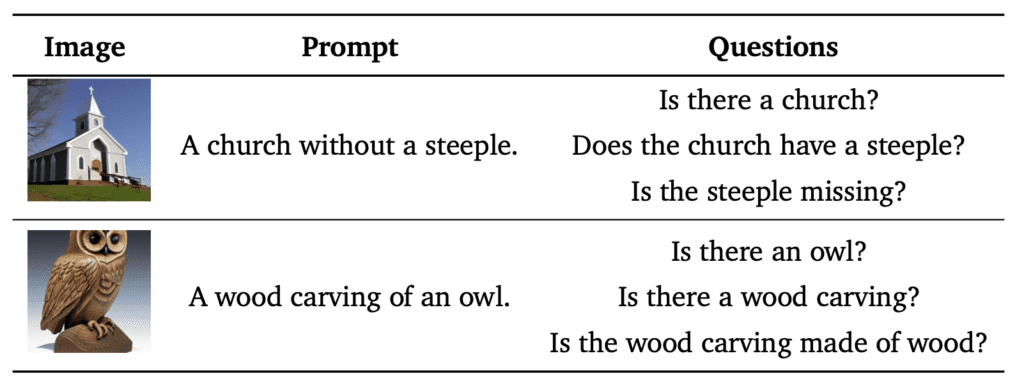
Challenges and Opportunities
The research identifies specific challenges such as the error-prone nature of automatic tagging and the limitations of current tagging mechanisms in capturing all relevant skills and sub-skills. Addressing these challenges requires a more sophisticated approach to data annotation and prompt generation, likely involving a combination of human and AI efforts.
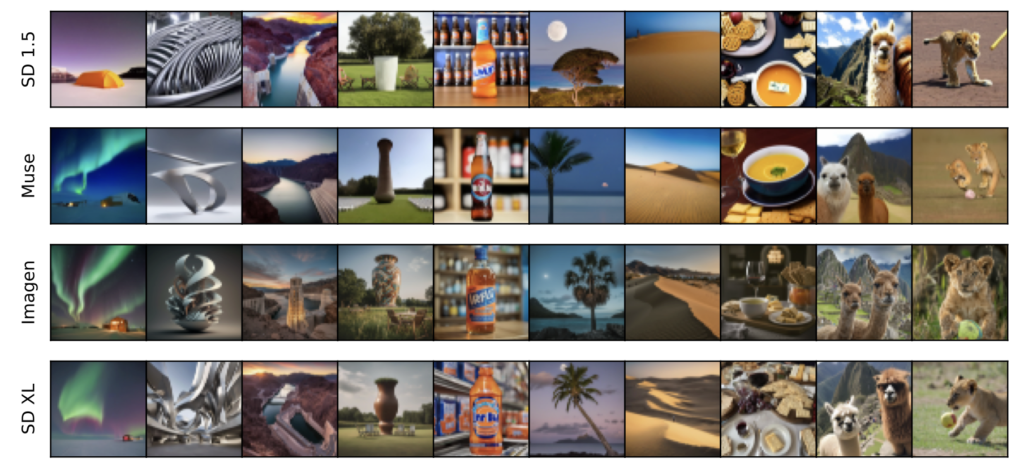
Google’s introduction of the Gecko framework marks a significant advancement in the field of AI evaluation, particularly for text-to-image models. By addressing the nuances of human judgment and the specificity of skill-based assessments, this research paves the way for more accurate, fair, and useful AI systems in creative and analytical applications.