Blending Fashion and Technology to Tailor Customized Digital Apparel
- Innovative Network Architecture: Magic Clothing utilizes a latent diffusion model-based network to create images of characters wearing specific garments, driven by detailed text prompts.
- Enhanced Image Controllability: The system incorporates a garment extractor and self-attention fusion to ensure garment details remain accurate and faithful to the input prompts.
- Robust Evaluation Metric: The introduction of Matched-Points-LPIPS (MP-LPIPS) as a metric for consistency ensures the synthesized images match the desired garment features accurately.
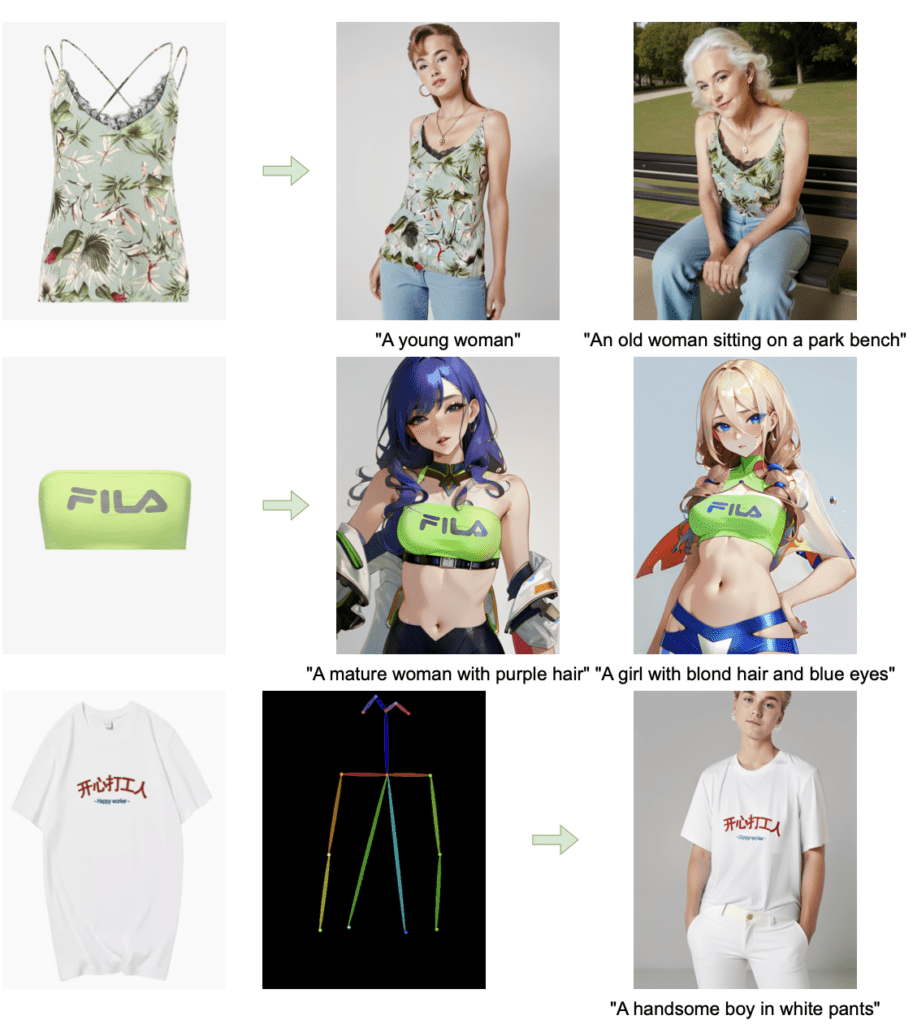
Magic Clothing represents a significant advance in the field of image synthesis, specifically focusing on garment-driven generation, an area previously unexplored. This technology allows for the customization of digital characters donning specific apparel, meticulously adhering to the details specified by users through text prompts.
Core Technology
The foundation of Magic Clothing is its latent diffusion model (LDM)-based network architecture, which integrates several innovative components to enhance the fidelity and diversity of generated images. Central to this system is the garment extractor, a sophisticated tool designed to capture intricate garment details. This extractor is combined with a self-attention fusion mechanism that integrates these details into pre-trained latent diffusion models without altering the original garment’s appearance on the target character.
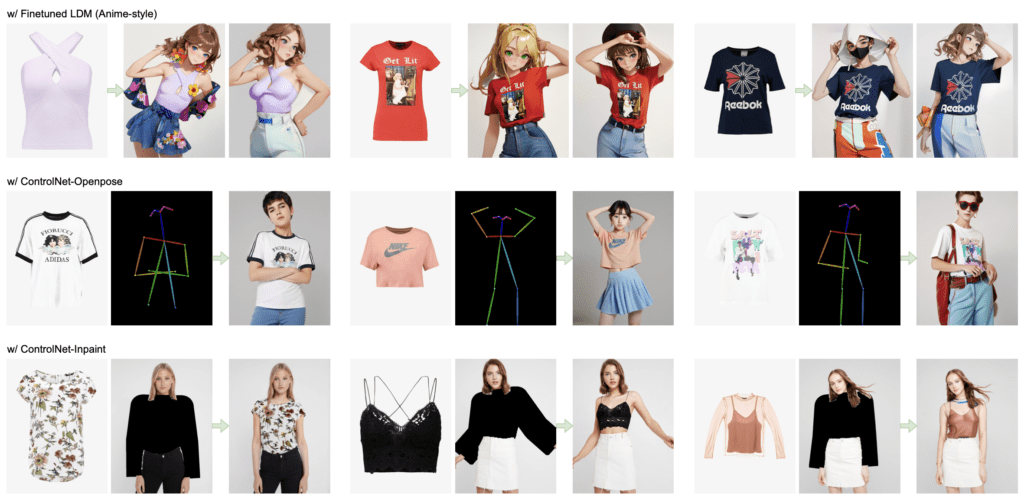
Controllability and Flexibility
To manage the delicate balance between adhering to the garment’s details and the text prompts, Magic Clothing employs joint classifier-free guidance. This approach finely tunes the influence of both garment features and textual instructions over the generated results, enhancing the controllability of the output. Additionally, the garment extractor functions as a plug-in module, making it versatile enough to be incorporated into various fine-tuned LDMs and compatible with other extensions such as ControlNet and IP-Adapter. This flexibility allows for increased diversity in the characters generated and more precise control over their appearance.
Evaluation and Metrics
Ensuring the consistency of the generated images with the source garments is crucial. To this end, the study introduces Matched-Points-LPIPS (MP-LPIPS), a robust metric specifically developed to evaluate how well the target images adhere to the source garment details. This metric has been instrumental in demonstrating the efficacy of Magic Clothing through extensive testing, which shows its capability to surpass existing methods under various conditional controls.
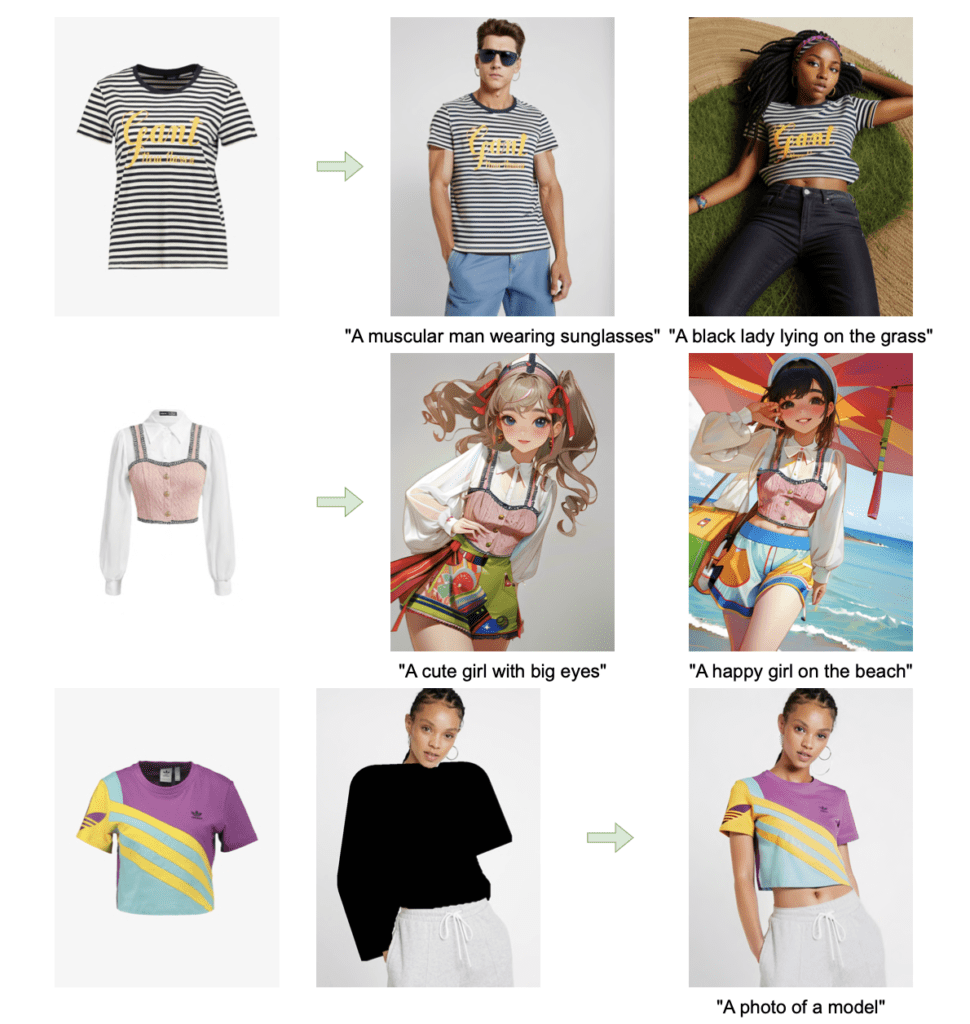
Challenges and Future Directions
Despite its success, Magic Clothing faces challenges, primarily due to the dependency on the underlying diffusion models’ capabilities and the limited diversity of the training samples in the VITON-HD dataset. Future improvements might include integrating more powerful pre-trained models and expanding the dataset to include a broader range of garments, which could help in generating better results for complex apparel like down jackets and coats.
In conclusion, Magic Clothing is a groundbreaking advancement in digital fashion technology, offering unprecedented control and diversity in garment-driven image synthesis. Its development not only showcases the potential of combining AI with fashion design but also sets a new standard for how clothing can be visualized and customized in the digital space.