Revolutionizing Monocular Depth Perception with the Precision of Edges
- Edge-centric Approach: ECFNet pioneers an innovative framework for monocular depth estimation by emphasizing the significance of edge information, demonstrating that edge maps alone can substantially enhance the sharpness and accuracy of depth maps.
- Synthetic Image Validation: Through the generation of synthetic images with ControlNet and stable diffusion models, ECFNet underscores the pivotal role of consistent edge structures in achieving highly similar depth maps, despite variations in textures and materials.
- Comprehensive Evaluation: The model’s efficacy is validated across multiple datasets, employing a range of metrics including SqRel, AbsRel, RMSE, and ORD, along with specialized edge depth quality assessments like ESR and EcSR, showcasing its superior performance in depth estimation tasks.
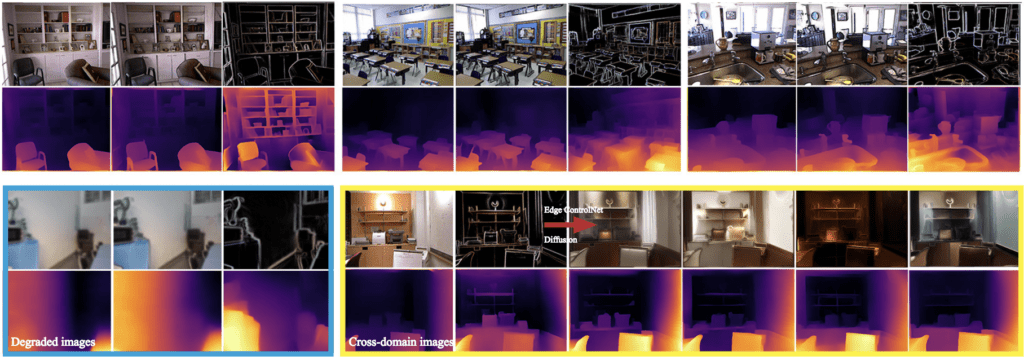
In the realm of computer vision, accurately perceiving the depth of a scene from a single image, known as monocular depth estimation, presents a complex challenge. Traditionally, models have struggled to replicate the depth perception of the human eye, often resulting in blurred or distorted depth maps. However, the introduction of ECFNet (Edge-aware Consistency Fusion Network) marks a significant advancement in this field, leveraging the power of edge information to redefine depth estimation accuracy.
Empowering Depth with Edges
ECFNet’s core innovation lies in its edge-centric approach. By focusing on edge maps, the model taps into the structural essence of scenes, enabling the generation of sharper, more detailed depth maps. This method contrasts with conventional techniques that might overlook the critical role of edges, often leading to depth estimations that lack clarity and precision.
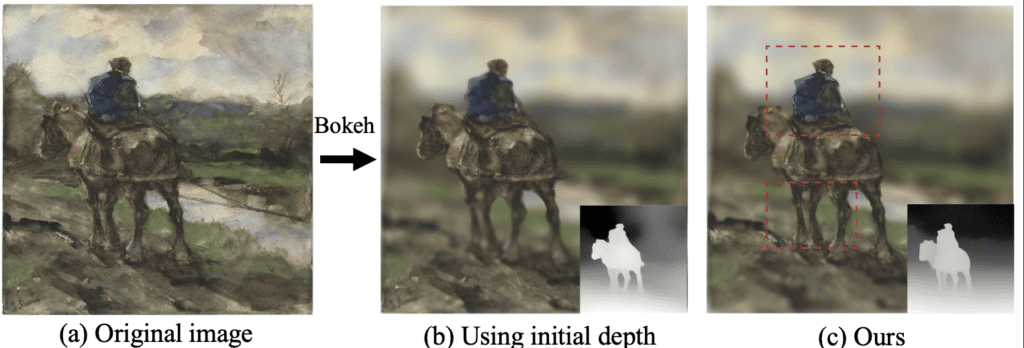
Synthetic Image Experimentation
A pivotal aspect of ECFNet’s development involved the creation of synthetic images, which share identical edge information but vary in textures and materials. This experimentation revealed that despite these variations, the resulting depth maps maintained a high degree of similarity, underscoring the edge structure’s paramount importance in depth estimation. This insight not only validates ECFNet’s foundational principle but also opens up new avenues for understanding depth perception in complex visual contexts.
Rigorous Evaluation and Real-world Applications
ECFNet has undergone extensive testing across several renowned datasets, including DIODE, IBims-1, and NYU-v2, employing a comprehensive set of evaluation metrics. Notably, the model excels in both general depth estimation accuracy and the nuanced assessment of edge depth quality, as indicated by specialized metrics like ESR and EcSR. This thorough evaluation underscores ECFNet’s potential to significantly improve applications reliant on depth perception, from 3D photo rendering to the creation of bokeh effects in photography.
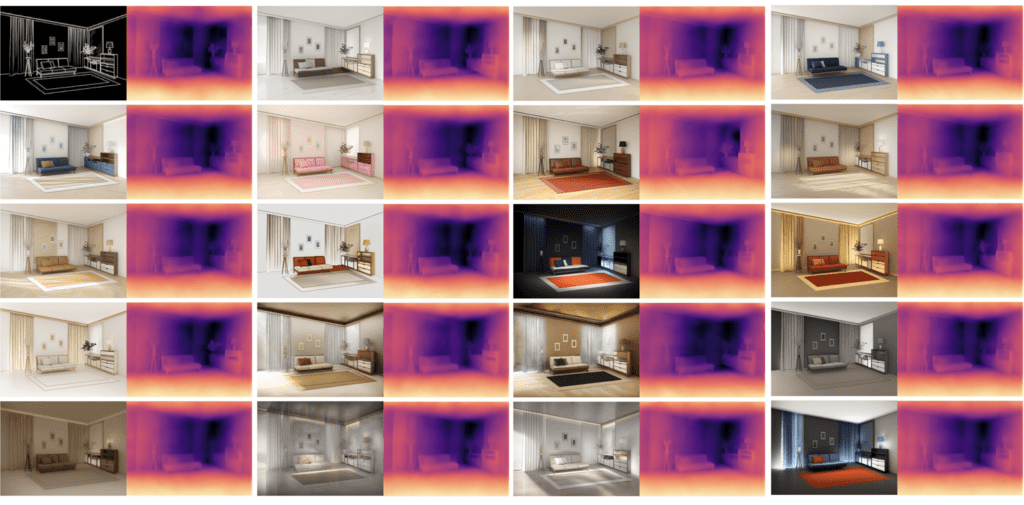
Despite its remarkable achievements, ECFNet’s journey is not without challenges. The field of depth estimation, particularly for artistic images, remains fraught with complexity due to the diverse nature of artistic expressions and the inherent scale ambiguity in depth perception. However, ECFNet’s innovative framework and promising results pave the way for future advancements, potentially transforming how machines understand and interact with the three-dimensional world around us.
ECFNet stands as a beacon of innovation in monocular depth estimation, demonstrating the untapped potential of edge information in enhancing depth perception. As the model continues to evolve, it holds the promise of unlocking new possibilities in computer vision, making our digital interactions with the world more intuitive and lifelike.