Google DeepMind’s Robot Reaches New Heights in Sports Robotics
- Breakthrough in Robot Table Tennis: Google DeepMind’s robot achieves amateur human-level performance in competitive table tennis, marking a significant milestone in robotics.
- Hierarchical and Modular Architecture: The robot uses a sophisticated system combining low-level skill policies and a high-level controller to adapt and compete against human opponents.
- Future Research Directions: Addressing latency, enhancing collision detection, and improving strategic capabilities are the next steps to refine the robot’s performance.
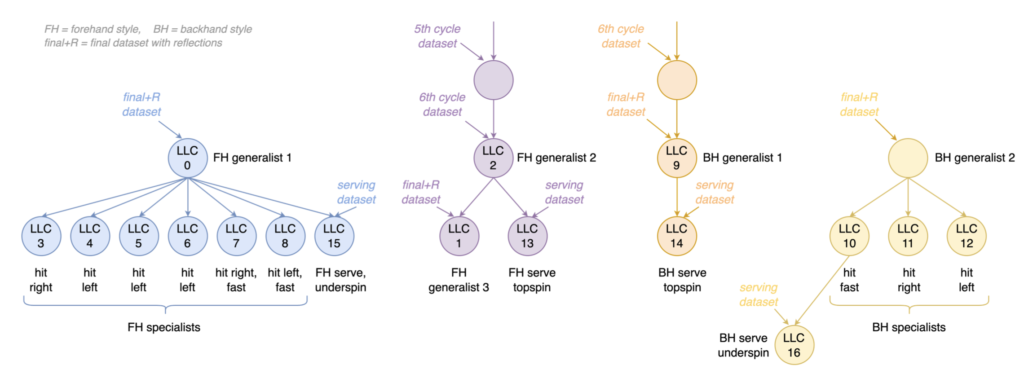
Google DeepMind has made a significant leap in robotics by developing a robot that can compete at an amateur human level in table tennis. This achievement underscores the potential of robots to perform real-world tasks that require speed, precision, and strategic decision-making. Table tennis, a sport known for its physical demands and rapid pace, has served as a benchmark for robotics research since the 1980s, and this new development marks a pivotal advancement.
The success of this robot is built on a hierarchical and modular policy architecture. At the core of the system are multiple low-level skill policies, each specializing in specific aspects of table tennis, such as forehand topspin or backhand targeting. These skills are managed by a high-level controller that selects the optimal move based on game statistics, skill descriptors, and the opponent’s abilities. This structure allows the robot to adapt its strategies dynamically, a crucial factor in competitive play.
In practical terms, the robot’s performance was tested through 29 matches against human players of varying skill levels, winning 45% of the games. While the robot struggled against advanced players, it excelled against beginners and intermediates, demonstrating its solid amateur-level capabilities. This is a significant accomplishment, considering the complexity and rapid decision-making required in table tennis.
Looking ahead, the research team identified several areas for improvement. Enhancing the robot’s reaction time to fast balls through advanced control algorithms and hardware optimizations is a priority. This could involve predictive models to anticipate ball trajectories and faster communication protocols between sensors and actuators. Additionally, improving the robot’s handling of low balls through sophisticated collision detection and avoidance algorithms will be crucial. The strategic capabilities of the robot can also be refined by training on longer rally scenarios and exploring self-play techniques to better capture the game state.
In conclusion, Google DeepMind’s development of a robot that competes at a human level in table tennis is a remarkable achievement in the field of robotics. It showcases the potential for robots to perform complex, real-world tasks and opens up new avenues for research and development. As the team continues to address the current limitations and refine the robot’s capabilities, we can expect even more impressive advancements in the near future.