Advancing 3D Scene Generation with Holistic Text-to-Image Models
- HoloDreamer generates highly consistent 3D panoramic scenes from text descriptions.
- The framework combines multiple diffusion models with 3D Gaussian Splatting (3D-GS) for robust scene reconstruction.
- Despite its success, challenges include data scarcity and optimization efficiency in panoramic generation.
In the evolving landscape of virtual reality, gaming, and film, the demand for advanced 3D scene generation is skyrocketing. HoloDreamer, a groundbreaking framework, addresses this demand by transforming text descriptions into intricate and fully enclosed 3D panoramic scenes. This innovation leverages the power of text-to-image diffusion models, offering a significant leap forward in text-driven 3D scene generation.
The HoloDreamer Framework
HoloDreamer stands out by addressing the limitations of previous methods, which often result in globally inconsistent and incomplete scenes. Traditional approaches generate initial local images and iteratively outpaint them, a process prone to inconsistencies. HoloDreamer, however, begins with high-definition panoramic generation, ensuring a holistic initialization of the entire 3D scene.
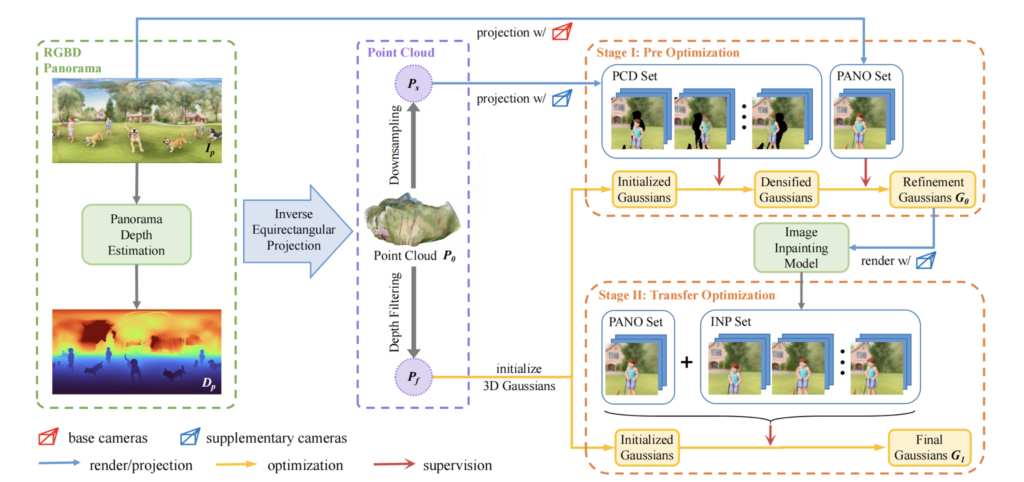
Stylized Equirectangular Panorama Generation:
- Combining Diffusion Models: This pipeline integrates multiple diffusion models to create stylized and detailed equirectangular panoramas from complex text prompts. This initial panoramic image serves as a comprehensive base for the 3D scene.
Enhanced Two-Stage Panorama Reconstruction:
- Two-Stage Optimization: The method utilizes 3D Gaussian Splatting (3D-GS) for quick and efficient 3D reconstruction. The first stage establishes the primary structure, while the second stage introduces additional cameras to inpaint missing regions, enhancing the scene’s integrity.
Experimental Success and Visual Consistency
Extensive experiments demonstrate that HoloDreamer excels in generating visually consistent and harmonious 3D scenes. The integration of multiple diffusion models ensures that the panoramas are not only detailed but also stylistically coherent. The two-stage reconstruction process significantly improves the rendering robustness, making the scenes more immersive and visually appealing.
Challenges and Future Directions
Despite its advancements, HoloDreamer faces several challenges:
- Data Scarcity: Panoramic image data are less abundant compared to perspective image data, limiting the model’s ability to handle more complex text descriptions. Future work could involve generating more diverse panorama datasets, potentially leveraging forthcoming video generation models.
- Optimization Efficiency: To ensure efficient 3D reconstruction, HoloDreamer currently limits itself to a two-stage process. Enhancing the number of iterative inpainting stages and optimizing camera setup strategies could further improve the scene’s integrity and rendering robustness.
- Error Compounding: The use of multiple diffusion models, while expanding the generative domain, can introduce compounded errors and increase variability. Refining the parameters and integration methods of these models will be crucial for improving overall consistency.
HoloDreamer represents a significant step forward in the realm of 3D scene generation, offering a comprehensive solution that transforms text descriptions into fully enclosed, visually coherent 3D worlds. By combining advanced diffusion models with robust reconstruction techniques, HoloDreamer sets a new benchmark for the industry. As the framework continues to evolve, it holds the promise of further advancements in virtual reality, gaming, and film, making the creation of immersive digital environments more accessible and sophisticated than ever before.