Resolving Ambiguity in Image-based Conditioning with Instruct Prompts
- IPAdapter-Instruct combines natural-image conditioning with instruct prompts to clarify user intent in image generation.
- This new approach maintains high-quality output while efficiently learning multiple tasks.
- Challenges include creating training datasets and biases in the conditioning model.
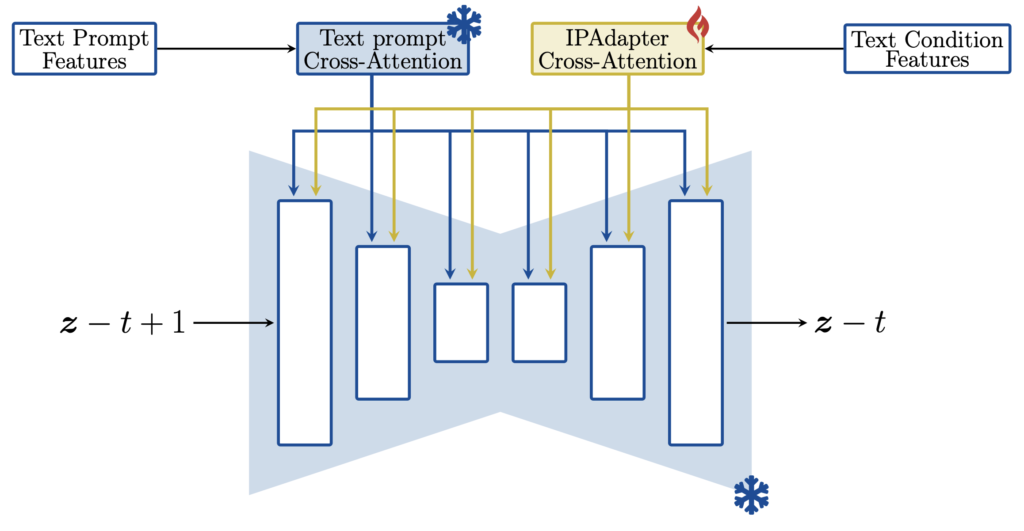
The field of image generation has made significant strides with the advent of diffusion models, which have pushed the boundaries of state-of-the-art performance. These models, which iteratively reverse the diffusion of images into pure noise, have demonstrated robustness in training and versatility in application, surpassing the capabilities of previous GAN-based models. However, the challenge of nuanced control in image generation persists, particularly when relying on textual prompts to define image style or structural details.
Addressing this gap, researchers have introduced IPAdapter-Instruct, a novel approach that combines the strengths of natural-image conditioning with the precision of “Instruct” prompts. This method allows users to specify their intent more clearly, whether it’s for style transfer, object extraction, or other tasks, within the same conditioning image. By incorporating instruction prompts, IPAdapter-Instruct efficiently manages multiple interpretations without significant loss in output quality.
Advancements in Image Generation Control
Traditional methods like ControlNet and IPAdapter have advanced the field by conditioning the generative process on imagery. However, each instance typically models a single conditional posterior, necessitating multiple adapters for diverse posteriors within a workflow. This approach can be cumbersome and inefficient for practical applications.
IPAdapter-Instruct tackles this issue by integrating instruction prompts that guide the model in interpreting the conditioning image. This technique not only consolidates multiple tasks into a single prompt-image combination but also retains the benefits of the original IPAdapter workflow. The result is a more streamlined and versatile image generation process that remains compatible with the base diffusion model and its associated LoRAs (Low-Rank Adaptations).
Efficiency and Quality in Training
One of the standout features of IPAdapter-Instruct is its ability to learn multiple tasks efficiently while maintaining high-quality outputs. The model achieves this by leveraging a joint training approach that minimizes performance loss typically associated with dedicated per-task models. This efficiency is crucial for practical deployment, where rapid and reliable image generation is essential.
However, the process of creating training datasets poses a significant challenge. It is both time-consuming and limited by the availability of source data. These constraints can impact the performance of specific tasks and introduce biases into the conditioning model. For instance, style transfer may be biased towards certain styles like those found in MidJourney, while face extraction performs best with real photos. Object extraction, on the other hand, benefits from colored padding, highlighting the variability in task performance based on training data nuances.
Future Directions and Challenges
Despite its promising capabilities, IPAdapter-Instruct faces limitations in tasks that require pixel-precise guidance. The compression into CLIP space, while effective for broader semantic guidance, struggles with tasks needing detailed pixel-level accuracy. The ultimate goal is to integrate both pixel-precise and semantic guidance into a unified conditioning model, further enhancing the clarity and accuracy of user instructions.
The introduction of IPAdapter-Instruct represents a significant step forward in the realm of text-to-image generation, providing a more intuitive and efficient means of controlling the output. By resolving ambiguities in user intent and streamlining the generation process, this innovative approach sets the stage for more advanced and versatile applications in image generation.
IPAdapter-Instruct offers a robust solution to the challenges of nuanced image generation control. By integrating instruction prompts with image conditioning, it enables efficient multi-task learning while maintaining high output quality. While the creation of training datasets remains a hurdle, the potential of this approach to refine and enhance image generation workflows is substantial. As the field continues to evolve, IPAdapter-Instruct is poised to play a crucial role in the development of sophisticated and user-friendly image generation models.