A Benchmark for Analyzing the Foundations of Visual Mathematical Reasoning
- Benchmark Introduction: WE-MATH is the first benchmark focused on the problem-solving principles behind LMMs’ performance, not just end results.
- Novel Metrics: Introduces a four-dimensional metric to assess issues in LMMs’ reasoning processes, including Insufficient Knowledge (IK) and Inadequate Generalization (IG).
- Evaluation Insights: Reveals a shift in challenges for advanced models like GPT-4o from IK to IG, highlighting a move towards knowledge generalization.
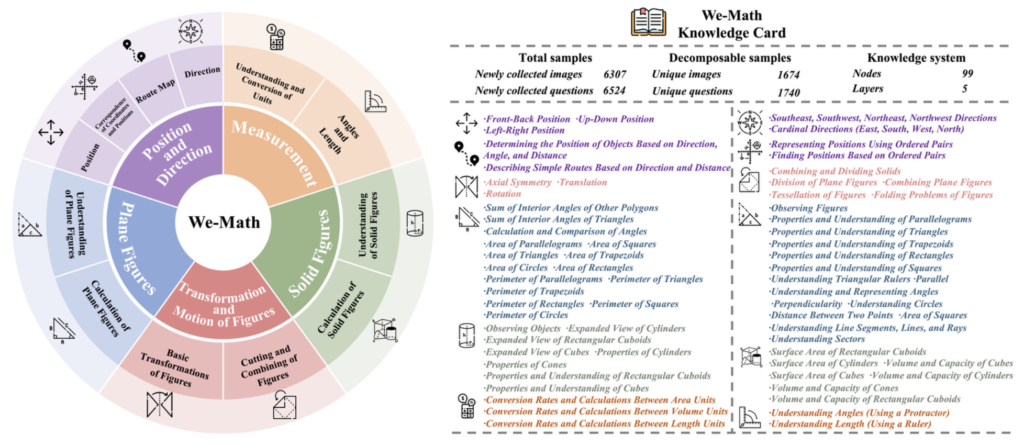
Visual mathematical reasoning has emerged as a fundamental area of interest in the Large Multimodal Models (LMMs) community. Traditional benchmarks have predominantly emphasized result-oriented performance, often neglecting the underlying principles that guide knowledge acquisition and generalization. Addressing this gap, the newly introduced WE-MATH benchmark provides a comprehensive framework to explore the foundational problem-solving principles that extend beyond mere end-to-end performance.
WE-MATH meticulously curates and categorizes 6.5K visual math problems, encompassing 67 hierarchical knowledge concepts and five layers of knowledge granularity. This rich dataset allows for an in-depth examination of how LMMs approach complex mathematical problems. The benchmark’s innovative approach decomposes composite problems into sub-problems, aligning them with the necessary knowledge concepts. This decomposition is crucial for understanding the reasoning process at a granular level.
A novel aspect of WE-MATH is its introduction of a four-dimensional metric system designed to hierarchically assess the inherent issues in LMMs’ reasoning processes. These metrics are:
- Insufficient Knowledge (IK): Evaluates whether the model lacks the necessary foundational knowledge.
- Inadequate Generalization (IG): Assesses the model’s ability to generalize knowledge across different contexts.
- Complete Mastery (CM): Indicates the model’s thorough understanding and ability to apply knowledge correctly.
- Rote Memorization (RM): Identifies if the model relies on memorized solutions rather than understanding.
Through extensive evaluation, WE-MATH reveals critical insights into the performance of current LMMs in visual mathematical reasoning. One significant finding is the negative correlation between the number of solving steps and problem-specific performance. This suggests that more steps do not necessarily equate to better problem-solving abilities, emphasizing the need for more efficient reasoning strategies.
The benchmark also highlights the primary challenge for advanced models like GPT-4o, which has transitioned from IK to IG. This transition indicates that GPT-4o is advancing towards the knowledge generalization stage, setting it apart from other LMMs that still exhibit a marked inclination towards rote memorization. These models can solve composite problems involving multiple knowledge concepts but struggle with the underlying sub-problems.
The introduction of WE-MATH is poised to open new pathways for advancements in visual mathematical reasoning for LMMs. By focusing on the cognitive and reasoning patterns inspired by human intelligence, WE-MATH aims to drive the development of models that not only perform well but also understand and generalize knowledge in a manner akin to human cognition.
Ideas for Further Exploration
- Adaptive Learning Techniques: Implementing adaptive learning algorithms that can dynamically adjust based on the model’s performance on sub-problems.
- Cross-Disciplinary Benchmarks: Extending the principles of WE-MATH to other domains such as physics or engineering, where visual reasoning plays a critical role.
- Human-Machine Collaboration: Exploring hybrid models that combine human cognitive strengths with the computational power of LMMs to enhance problem-solving capabilities.
WE-MATH represents a significant step forward in understanding and improving the foundational reasoning abilities of LMMs. By emphasizing problem-solving principles over end results, this benchmark offers a robust framework for evaluating and advancing the state of visual mathematical reasoning in AI models.