A groundbreaking framework merges layered decomposition and fusion for nuanced spatial-aware image editing, surpassing conventional methods.
- Multi-Layered Approach: The framework innovates with a multi-layered latent decomposition and fusion, enabling precise manipulation of image elements across various layers.
- Innovative Techniques for Quality Enhancement: Introduces a key-masking self-attention mechanism and an artifact suppression scheme to refine the editing of background and occluded object layers.
- Unified Framework for Diverse Editing Tasks: Demonstrates versatility across numerous editing tasks, setting a new benchmark in spatial-aware image editing with its unified approach.
Microsoft presents DesignEdit. In the evolving landscape of image editing, particularly with the burgeoning success of text-to-image generation models, the quest for precision has led to remarkable innovations. A recent study proposes a unified framework that adopts a layered approach from the design domain, significantly enhancing the flexibility and accuracy of object manipulation within images.
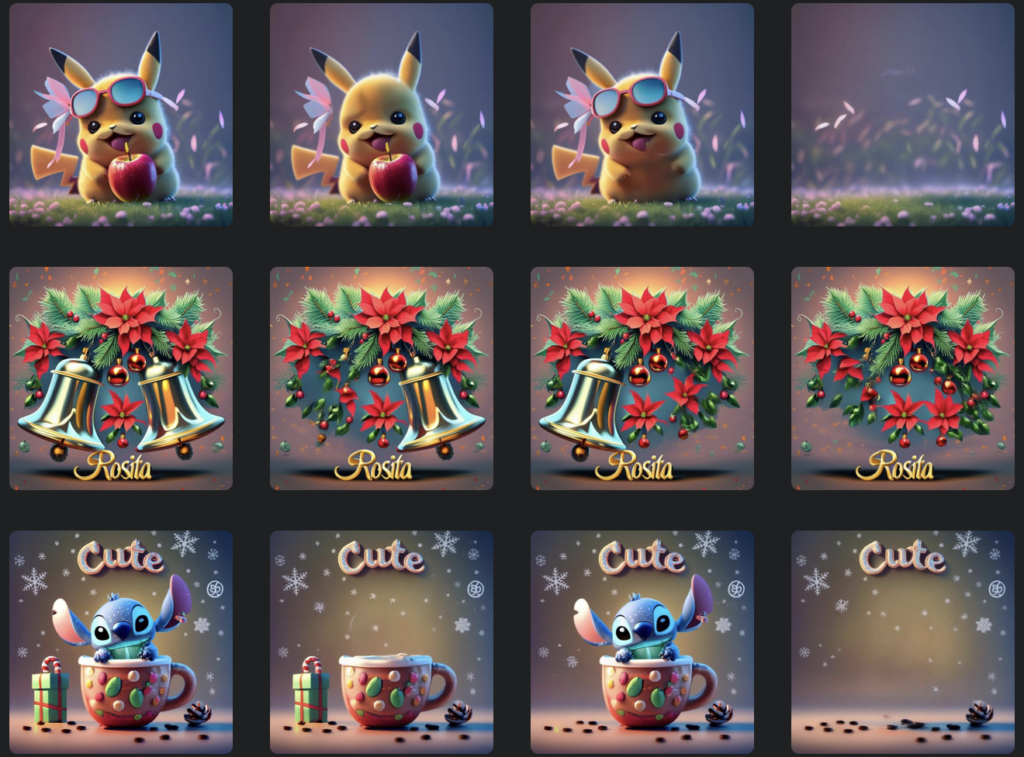
Decomposition and Fusion for Spatial Precision
At the core of this framework is the division of the spatial-aware image editing task into two critical sub-tasks: multi-layered latent decomposition and multi-layered latent fusion. This begins with segmenting the latent representations of source images into multiple distinct layers, including various object layers and an incomplete background layer. The latter necessitates a sophisticated inpainting solution to seamlessly fill in the gaps left by removed objects.
Enhancing Inpainting with Key-Masking Self-Attention
To circumvent the need for additional tuning while addressing the challenges of inpainting, the study introduces an ingenious key-masking self-attention mechanism. This technique allows for the effective propagation of contextual information into masked regions, enhancing the cohesiveness of the inpainted areas without adversely affecting unmasked regions.
Artifact Suppression for Cohesive Layer Fusion
The fusion process involves assembling the multi-layered latent representations onto a canvas latent, guided by specific instructions. To ensure the integration is seamless and free from artifacts, an artifact suppression scheme is employed within the latent space, significantly elevating the quality of the final edited image.
Empirical Validation and Comparison
The framework’s efficacy is not just theoretical but is empirically validated across a spectrum of image editing tasks, from simple object manipulations to complex spatial arrangements. Quantitative and qualitative comparisons with existing spatial editing methods, such as Self-Guidance and DiffEditor, underscore the superior performance of this new approach. Moreover, the framework’s compatibility with layout planning capabilities of advanced models like GPT-4V further underscores its robustness and versatility.
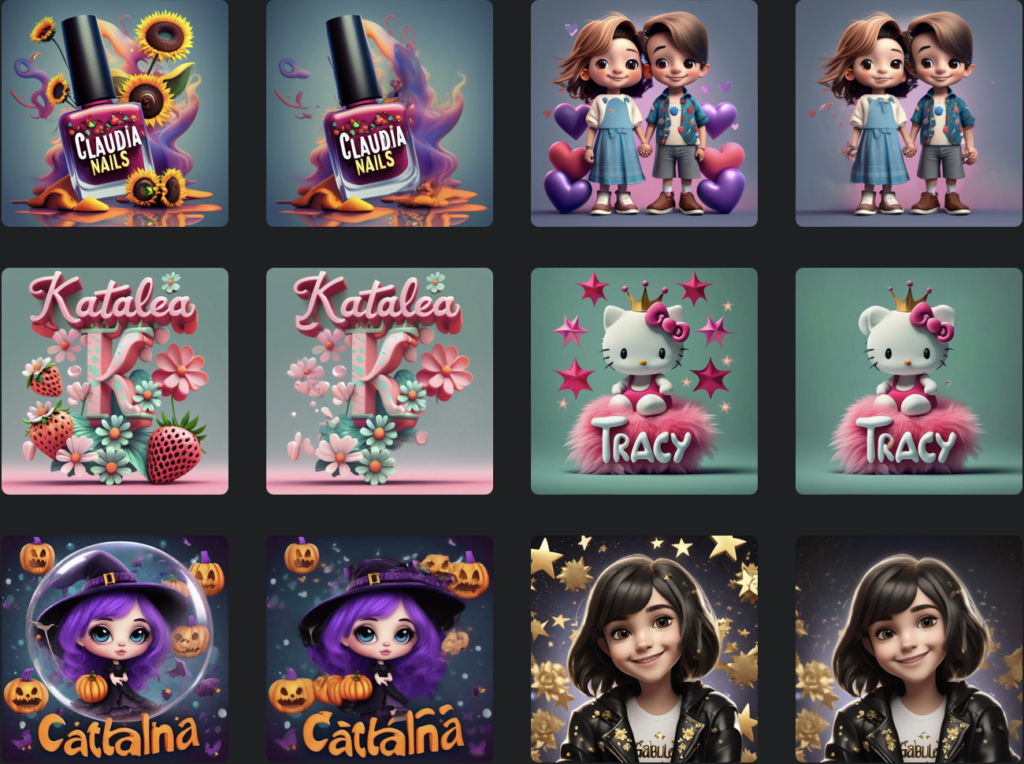
Bridging the Gap in Expectation and Reality
This innovative approach addresses a critical gap in current image generation models, which often struggle with spatial arrangements and numeracy in response to textual prompts. By enabling precise spatial-aware editing, this framework ensures that the final images align more closely with user expectations, as demonstrated in the study’s ability to correct inaccuracies like the number of objects depicted.
This multi-layered latent decomposition and fusion framework heralds a new era in image editing, offering unprecedented precision and flexibility. By combining advanced techniques like key-masking self-attention and artifact suppression, it provides a comprehensive solution for a wide range of spatial-aware image editing challenges, establishing a new standard for future developments in the field.