Maximizing Human Utility with Binary Feedback to Refine AI-Generated Imagery
- Innovative Alignment Strategy: Diffusion-KTO introduces a novel utility maximization approach to align text-to-image diffusion models with human preferences, leveraging simple binary feedback instead of complex preference data.
- Superior Model Performance: Post fine-tuning with Diffusion-KTO, text-to-image models showcase enhanced alignment with human judgments, outperforming traditional methods in both human evaluations and automated metrics like PickScore and ImageReward.
- Broader Applicability and Ethical Considerations: While demonstrating significant advancements in aligning models with user preferences, Diffusion-KTO also highlights the need to mitigate potential biases and undesirable content generation.
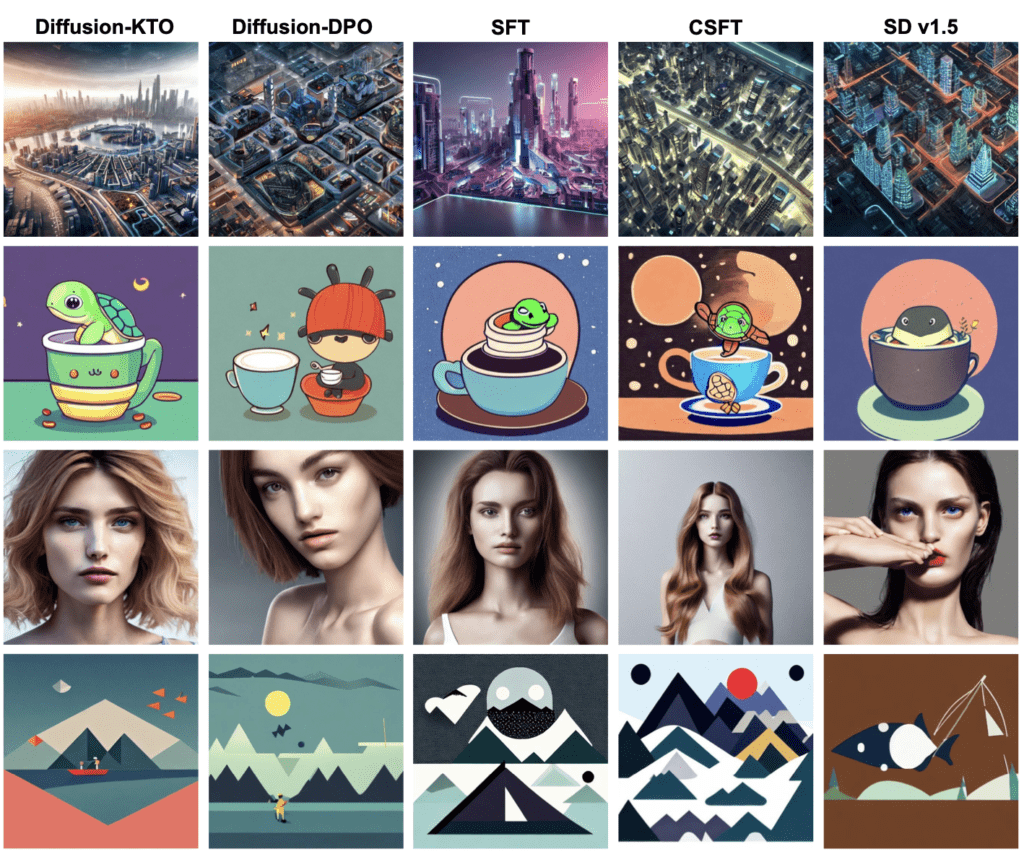
In the rapidly advancing domain of generative AI, the quest for creating models that can produce content aligned with human preferences has taken a significant leap forward with the introduction of Diffusion-KTO. This groundbreaking approach redefines how text-to-image diffusion models are fine-tuned to cater to individual tastes, eschewing the need for extensive preference datasets in favor of accessible binary feedback signals such as “likes” and “dislikes.”
A New Paradigm in Model Alignment
Diffusion-KTO stands out by framing the alignment objective as the maximization of expected human utility, a method that applies to each generated image independently. This innovative strategy circumvents the traditional pitfalls of collecting pairwise preference data, which is often costly and time-consuming. By harnessing readily available binary feedback, Diffusion-KTO offers a more scalable and efficient pathway to refine text-to-image models in accordance with human preferences.
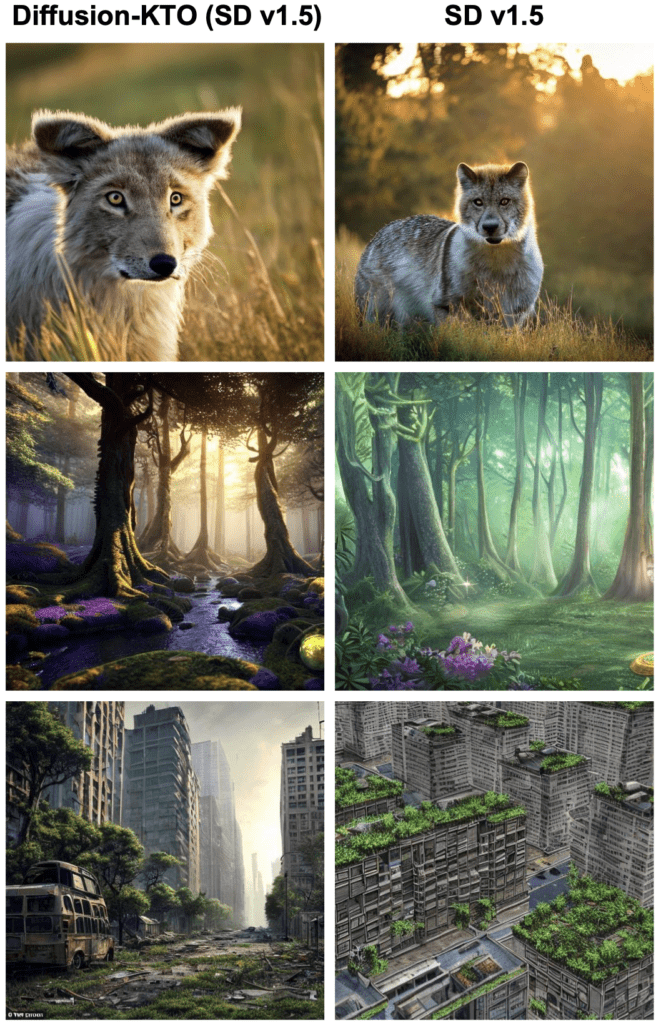
Enhanced Performance and User Satisfaction
The efficacy of Diffusion-KTO is evident in the marked improvement of model performance post fine-tuning. When assessed through rigorous human judgment panels and objective automated metrics, models aligned using Diffusion-KTO consistently outshine those fine-tuned via conventional methods. This superior alignment with human preferences underscores the potential of utility maximization approaches in elevating the quality and relevance of AI-generated imagery.
Navigating Challenges and Future Directions
Despite its considerable successes, Diffusion-KTO is not without its challenges. The reliance on online user-generated prompts and off-the-shelf text-to-image models introduces the risk of skewing preference data towards inappropriate or undesired content. Additionally, the inherited limitations of pre-trained models, such as the perpetuation of negative stereotypes or misalignment with prompts, underscore the importance of continuous scrutiny and improvement.
Diffusion-KTO’s broader framework for model alignment opens up new avenues for exploration, particularly in the choice and application of utility functions. The Kahneman-Tversky model of human utility has shown promise, but the field remains ripe for innovation, with numerous other utility functions awaiting evaluation.
Diffusion-KTO represents a significant stride toward realizing more personalized and human-centric digital content creation. By prioritizing human utility and simplifying the feedback mechanism, it sets a new standard for aligning AI capabilities with user preferences. As we move forward, the continuous refinement of these models, coupled with a vigilant approach to ethical considerations, will be paramount in harnessing the full potential of generative AI while safeguarding against its misuse.