Unveiling Enhanced Facial Recognition in AI-Generated Images through Innovative Loss Functions and Synthetic Data Training
- Innovative Identity-Lookahead Loss: Introducing a novel training approach that leverages an identity-lookahead loss to significantly improve the preservation of facial identities in AI-generated images, enhancing the fidelity and accuracy of personalized content.
- Synthetic Data Utilization: Employing synthetic, consistently generated data to fine-tune the IP-Adapter model, thereby avoiding the common pitfall of collapsing to photorealistic outputs and maintaining creative diversity in stylized images.
- Advanced Encoder Personalization Techniques: Utilizing LCM-LoRA for enhanced previewing of final outputs, enabling more precise backpropagation of image-space losses and leading to improved identity preservation and prompt alignment in text-to-image models.
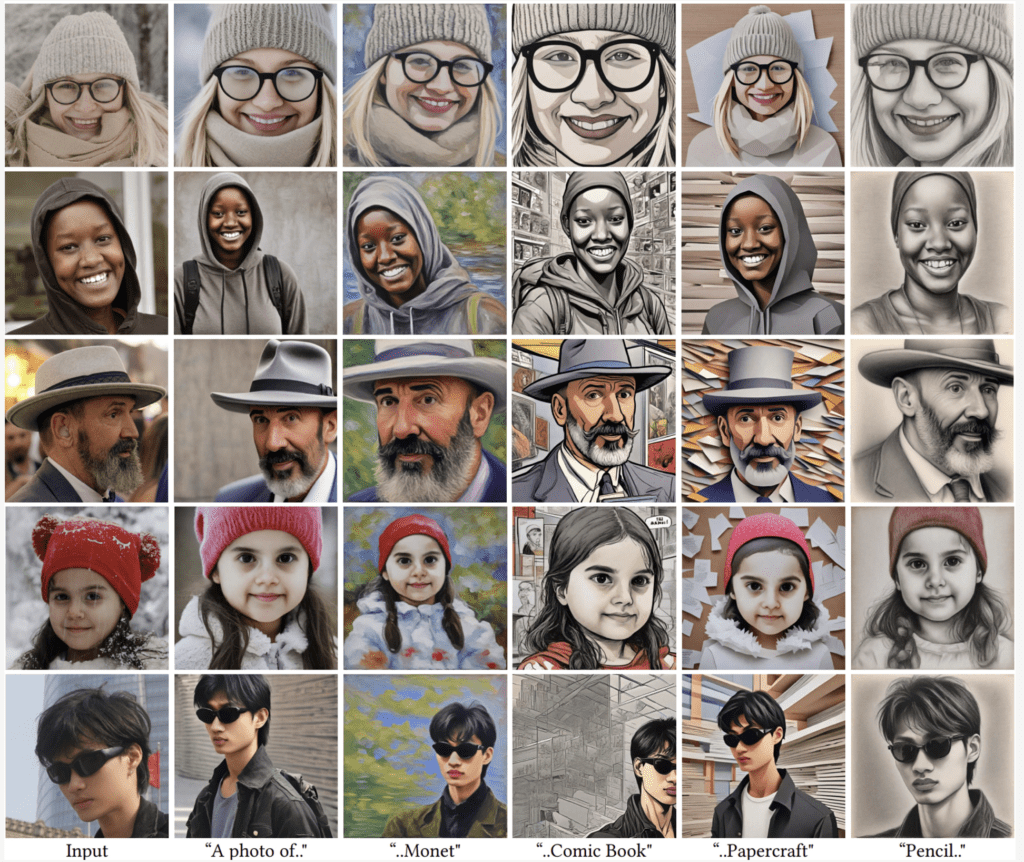
The realm of AI-generated imagery is witnessing a groundbreaking advancement with the introduction of a new technique that promises to redefine the standards of personalized text-to-image synthesis. At the heart of this innovation is the integration of a novel identity-lookahead loss function and the strategic use of synthetic data, both aimed at significantly enhancing the accuracy and diversity of AI-generated facial images.
Breaking New Ground with Identity-Lookahead Loss
The crux of this transformative approach lies in the application of an identity-lookahead loss during the training process. This innovative loss function allows for the propagation of image-space losses, such as ID (identity) and CLIP (Contrastive Language–Image Pre-training) losses, through the LCM-LoRA (Latent Conditioning Module – Low-Rank Adaptation) framework, bypassing the limitations of single-step Diffusion Denoising Probability Models (DDPM) approximations. By leveraging the more accurate previews provided by LCM-LoRA, which align with the final multi-step DDPM outputs, this method enables a more effective calculation of image-space losses, ensuring higher fidelity in identity preservation.
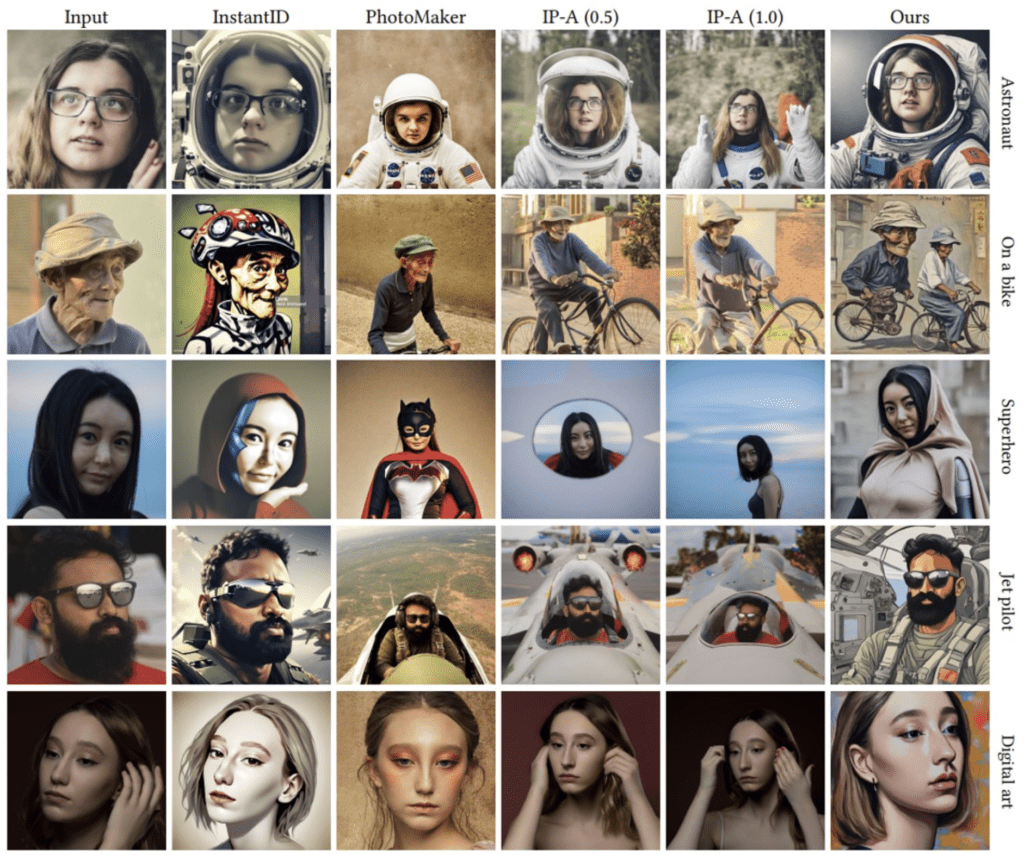
Leveraging Synthetic Data for Enhanced Diversity
A pivotal aspect of this novel approach is its reliance on synthetic data, generated through consistent sampling methods that intentionally exploit certain limitations (e.g., mode collapse) of advanced generative models like SDXL-Turbo. This strategy ensures that the training data encompasses a wide range of stylized images, thereby preventing the model from defaulting to purely photorealistic outputs. The result is a more versatile text-to-image model capable of producing diverse and creative representations while maintaining high fidelity to specified facial identities.
Elevating Encoder Personalization
Further enhancing the efficacy of this method is the use of attention-sharing mechanisms and the fine-tuning of the IP-Adapter model using the LCM-based lookahead identity loss. These techniques, combined with the consistent synthetic data and a self-attention sharing module, contribute to a significant improvement in both identity preservation and prompt alignment. This comprehensive approach not only surpasses the capabilities of existing SDXL encoder-based personalization methods but also introduces a new benchmark for the customization of AI-generated imagery.
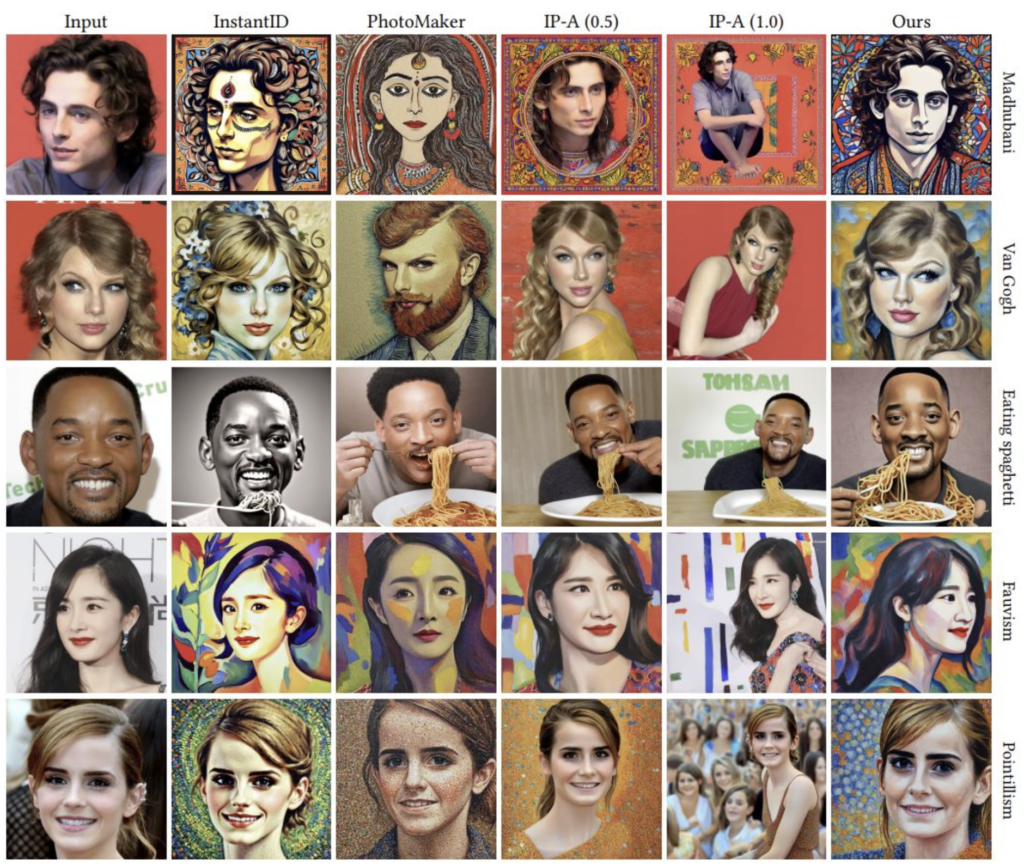
The comparative analysis with prior methodologies, including the use of the HuggingFace spaces implementations, underscores the superior performance of this approach across various metrics. By meticulously fine-tuning the model with a carefully calibrated blend of innovative loss functions, synthetic data, and advanced personalization techniques, this work opens up new horizons in the creation of AI-generated images that are not only visually captivating but also remarkably true to the individual identities they aim to portray.
This pioneering work not only signifies a substantial leap forward in the domain of text-to-image adaptation but also sets a new standard for the integration of personalized elements into AI-generated content, paving the way for more authentic and diverse digital representations of human identities.