Bridging the Gap Between 2D Images and 3D Models with Advanced AI Techniques
- Rapid and Efficient 3D Mesh Generation: InstantMesh combines a multiview diffusion model with a transformer-based sparse-view reconstruction to convert single images into 3D meshes within seconds.
- Open Source and Community-Focused: The framework, including its code, weights, and demos, is fully open-source, aiming to enhance contributions to the field of 3D generative AI and support both researchers and creators.
- Continuous Improvement and Limitations: Despite achieving significant advancements over existing methods, InstantMesh acknowledges current limitations and the need for future enhancements in high-definition modeling and consistency.
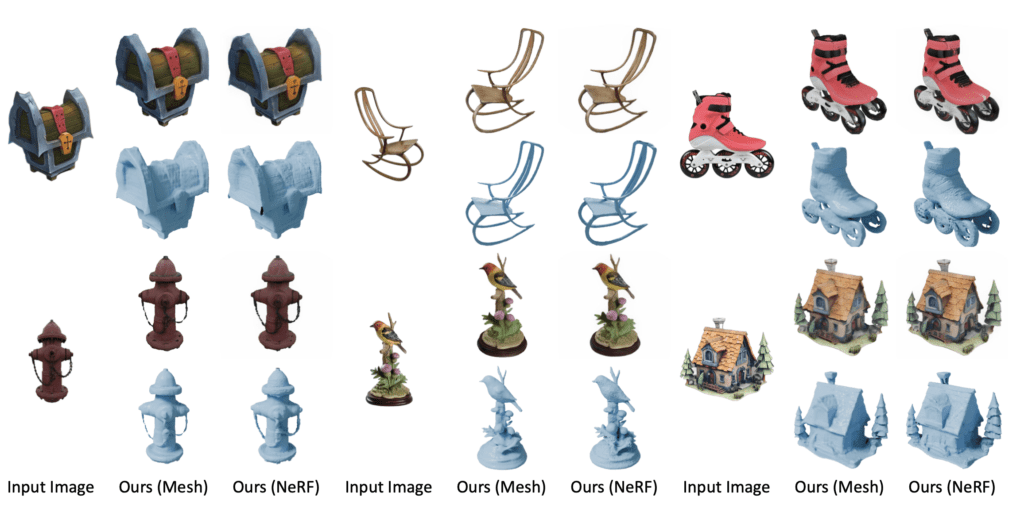
InstantMesh introduces an innovative approach to 3D modeling by generating detailed 3D meshes from a single image, leveraging the latest advancements in AI and computer vision. This tool represents a significant step forward in making 3D asset creation more accessible and efficient, particularly beneficial for industries like gaming, virtual reality, and digital content creation.
Technical Framework and Innovations
InstantMesh utilizes a cutting-edge combination of a multiview diffusion model and a transformer-based sparse-view large reconstruction model. This synergy allows for the rapid creation of 3D models, boasting impressive generation quality and scalability in training. The inclusion of a differentiable iso-surface extraction module optimizes the mesh representation directly, enhancing the geometric accuracy by using depth and normal data for better supervision.
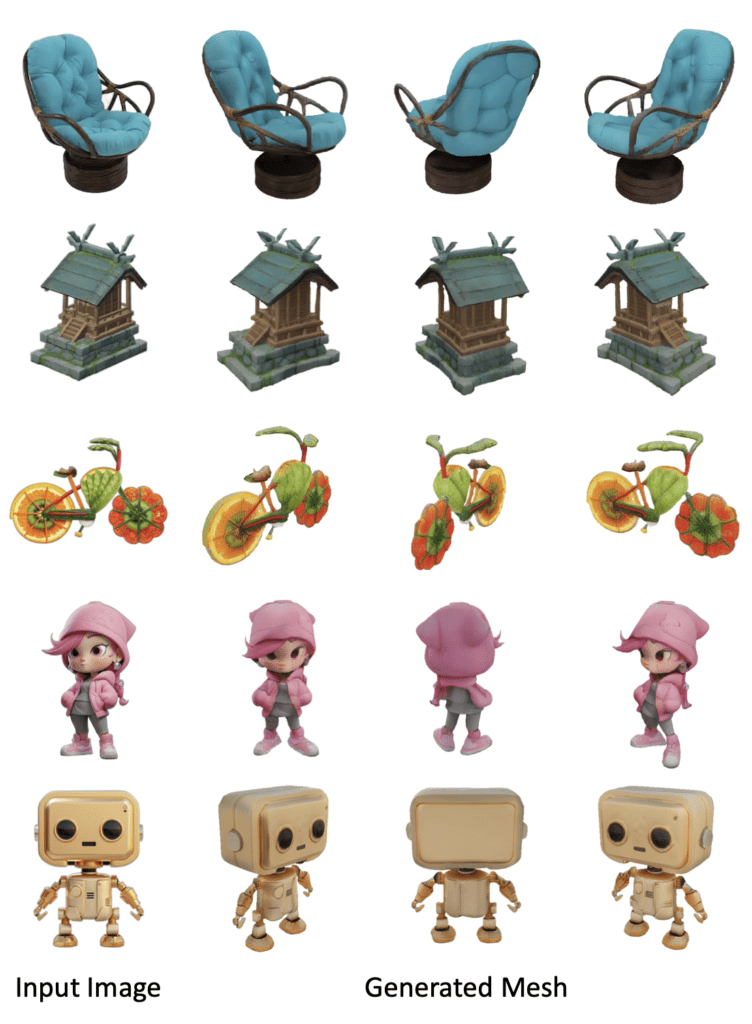
Training Efficiency and Mesh Quality
By integrating advanced geometric supervisions and focusing on a mesh-based representation, InstantMesh significantly improves both the training efficiency and the quality of the reconstructions. These improvements are evident in the framework’s ability to outperform other image-to-3D conversion tools qualitatively and quantitatively on public datasets. The use of FlexiCubes aids in refining the smoothness and reducing surface artifacts, although challenges remain in accurately modeling very fine or thin structures.
Community Engagement and Open Source Commitment
True to the spirit of collaborative advancement in AI, the developers of InstantMesh have made the framework open-source. This decision facilitates greater involvement from the global research community and creative professionals, enabling them to modify, improve, and adapt the tool according to their specific needs. The release includes all necessary code, weights, and a demonstration, making it readily usable and adaptable.
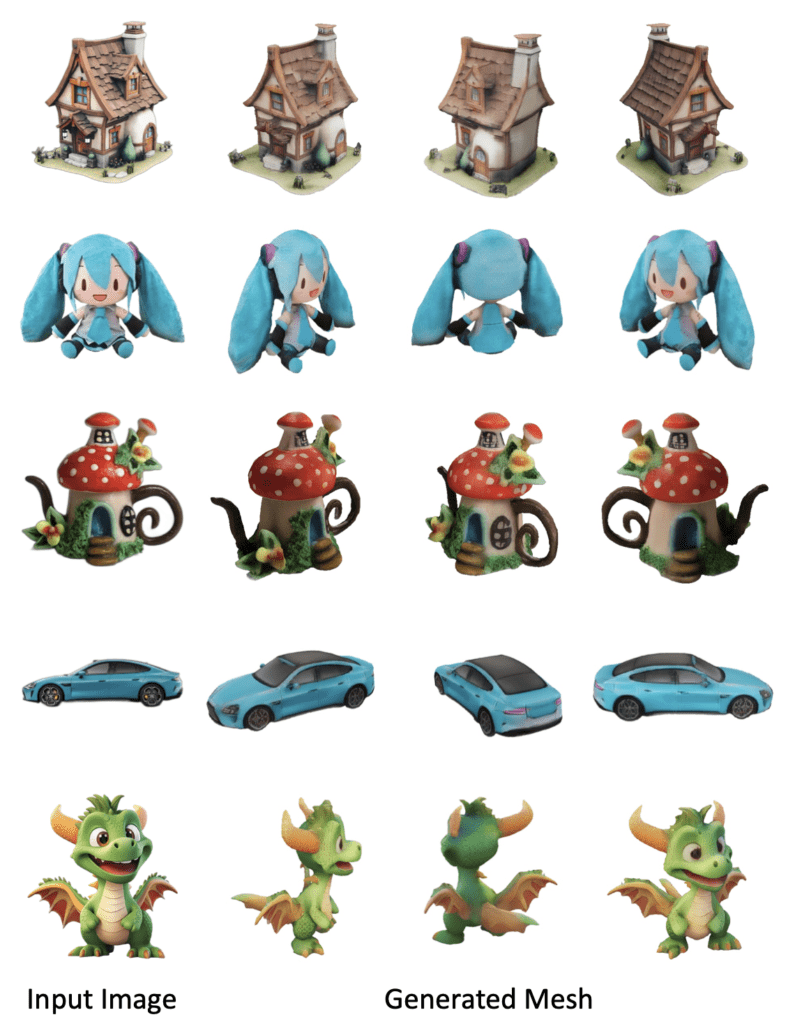
Challenges and Future Directions
Despite its achievements, InstantMesh faces limitations, such as the resolution restrictions imposed by the transformer-based triplane decoder and inconsistencies arising from the multi-view diffusion model. Future developments will focus on incorporating more advanced multi-view diffusion architectures to address these issues and improve the fidelity of the generated 3D models. Enhancements are also needed to better capture the intricacies of tiny and thin structures, a challenge currently better addressed by techniques like NeRF.
InstantMesh represents a formidable progress in the field of 3D generative AI, providing a fast, efficient, and accessible tool for transforming single images into detailed 3D meshes. As the framework evolves, it promises to push the boundaries of what’s possible in digital 3D reconstruction, further blurring the lines between digital and physical realities.