Enhancing App Searchability Through Advanced Image-Text Matching
- Novel Matching Approach: Apple introduces a new fine-tuning approach for pre-trained cross-modal models, significantly enhancing the matching of application images to relevant search phrases used by potential users.
- High Performance: The approach achieves high accuracy, with AUC scores of 0.96 and 0.95, outperforming existing models by 8%-17% in aligning images with developer and annotator intuitions on search phrase relevance.
- Dual Perspective Evaluation: The model’s effectiveness is demonstrated through evaluations based on both application developers’ insights and professional human annotators’ opinions, ensuring robustness and relevance in real-world applications.
In the digital age, app visibility and engagement depend heavily on how well an application can be discovered through search. Recognizing this, Apple has launched ‘Automatic Creative Selection with Cross-Modal Matching‘, a groundbreaking initiative to optimize how apps are visually represented in search results based on textual queries. This new technology employs a sophisticated cross-modal matching framework that leverages a fine-tuned version of a pre-trained model, specifically designed to associate application images with search phrases effectively.
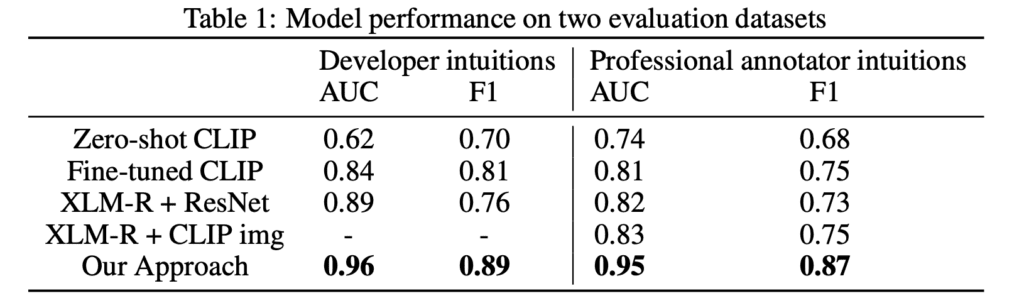
The technology utilizes a dual approach in its evaluation: one that considers the app developers’ expectations of which images align with specific search phrases, and another that takes into account the judgments of professional annotators. This comprehensive assessment strategy ensures the model is versatile and effective across various real-world applications.
The core of the technology lies in its advanced cross-modal BERT architecture, which integrates both textual and visual data to predict the relevance of images to search phrases accurately. Unlike previous models that relied heavily on direct description matching, Apple’s method understands the nuanced relationship between images and the search phrases they are likely to trigger. This is achieved through strategic mid-fusion of modalities, allowing for deeper interaction and better alignment compared to traditional early-fusion techniques.
Furthermore, the model’s training involves a unique dataset comprising pairs of search phrases and images, labeled for relevance, enabling the system to learn and predict with high precision. This setup not only enhances the model’s ability to select the most appropriate images for app promotion but also allows developers to effectively engage a broader audience by improving app discoverability and relevance.
As app markets become increasingly competitive, Apple’s ‘Automatic Creative Selection’ stands out as a crucial tool for developers seeking to capture user attention and improve engagement rates through smarter, data-driven image selections. This innovation is set to transform app marketing strategies, making it easier for developers to connect with potential users in meaningful and impactful ways.