Leveraging the Semantic Power of CLIP for Enhanced Image Manipulation
- Introduction of pOps Framework: pOps trains specific semantic operators directly on CLIP image embeddings, allowing for advanced visual content generation.
- Improved Semantic Control: By working directly over image embeddings, pOps enhances the ability to learn and apply semantic operations, providing precise control over generated images.
- Potential and Limitations: While pOps shows significant promise, it faces challenges with visual attribute preservation and may benefit from further computational scaling.
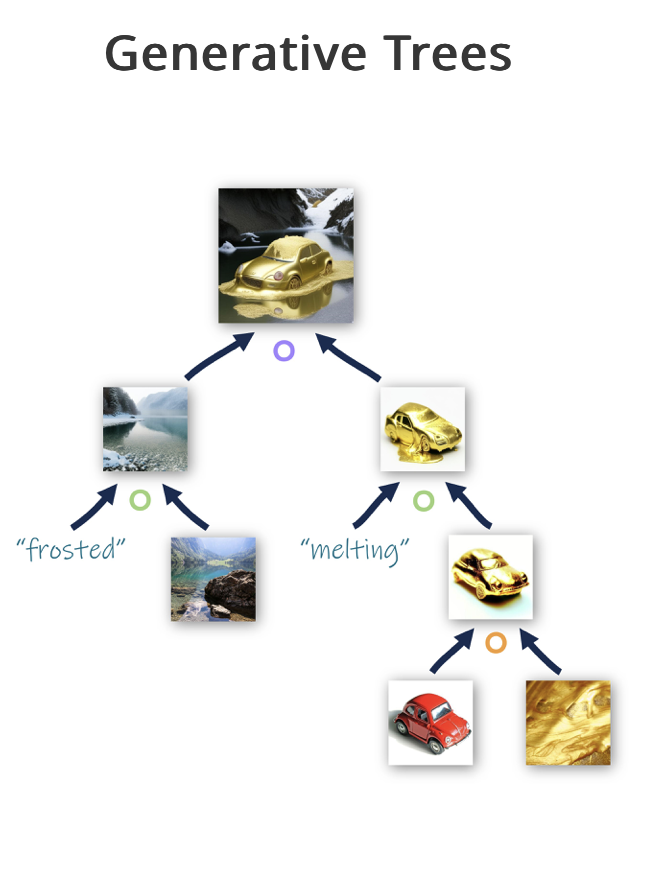
The quest for precise and intuitive control in visual content generation has taken a significant leap forward with the introduction of Photo-Inspired Diffusion Operators, or pOps. Developed as a novel framework for training semantic operators on CLIP image embeddings, pOps aims to enhance the way we generate and manipulate images based on textual descriptions.
Understanding pOps and Its Innovations
Text-guided image generation has become a popular method for creating visual content. However, language alone often falls short in conveying certain visual concepts, leading to a resurgence of interest in using CLIP image embedding space for more visually-oriented tasks. This space has shown to be semantically meaningful, where linear operations can yield semantically rich results. Yet, the specific meanings of these operations can vary unpredictably across different images.
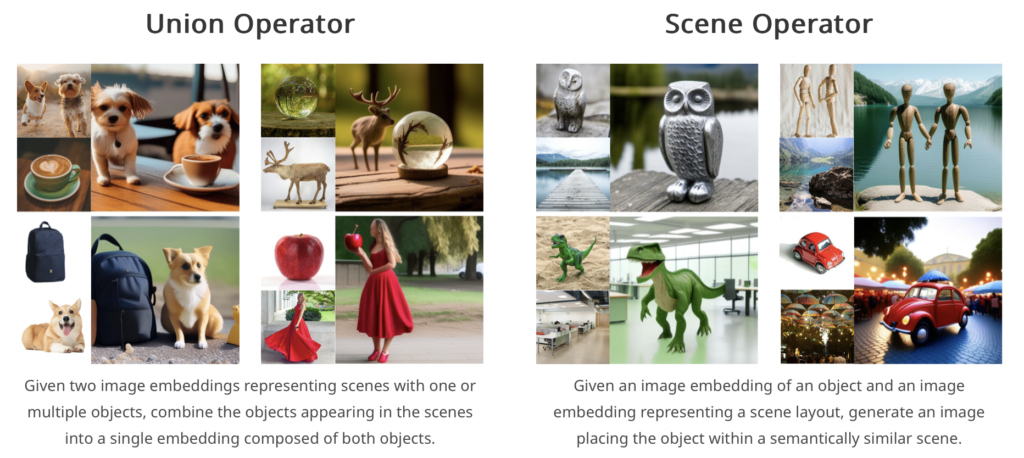
To harness the potential of this embedding space, pOps introduces a framework that trains specific semantic operators directly on CLIP image embeddings. Each pOps operator is built upon a pretrained Diffusion Prior model. While this model was initially designed to map between text and image embeddings, it can be tuned to create diffusion operators, enhancing our ability to perform semantic operations on images.
How pOps Enhances Image Generation
The pOps framework leverages the CLIP embedding space’s inherent semantic richness to enable more nuanced image generation. The key innovation lies in training these operators to perform specific semantic tasks. This process involves sampling a pool of images at each denoising step, selecting suitable win-lose pairs, and using these pairs to guide the next step of denoising. This approach ensures that each step in the image generation process is aligned with the desired semantic outcome.
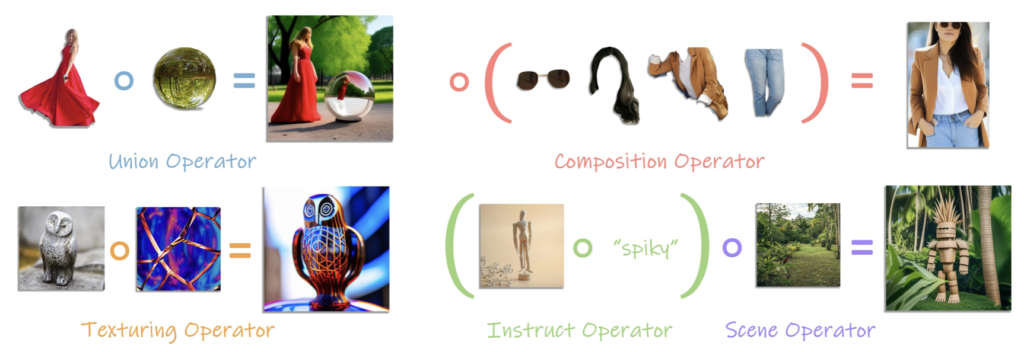
One significant advantage of pOps is its ability to use a textual CLIP loss for additional supervision, making the training process more robust and the generated images more aligned with complex, detailed prompts. This method has been shown to outperform existing techniques like Diffusion-DPO, particularly in enhancing aesthetics and achieving faster training times—over 20 times more efficient.
The Potential and Challenges of pOps
The introduction of pOps opens up new possibilities for semantic control in image generation. By allowing users to manipulate image embeddings directly, it provides a level of control that was previously difficult to achieve. This capability is particularly valuable for tasks that require precise visual manipulation, such as creating compositions or merging concepts.
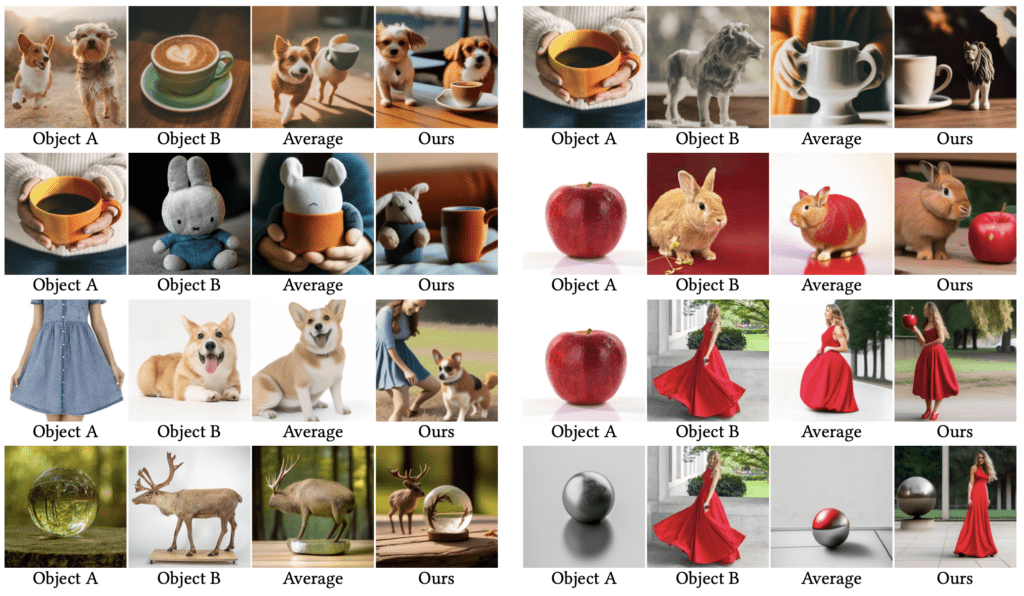
However, the pOps framework does have its limitations. Operating within the CLIP domain means that some visual attributes may not be preserved as effectively as desired. For example, while the embedding space can encode objects semantically, it may struggle with encoding distinct visual appearances or binding different visual attributes to separate objects. Additionally, pOps operators are tuned independently, which may not be as efficient as training a single diffusion model capable of handling all operations.
POps represents a significant advancement in the field of text-guided image generation. By training semantic operators directly on CLIP image embeddings, it offers a new level of control and flexibility in creating visual content. Despite some limitations, the framework’s potential for enhancing image generation is undeniable.
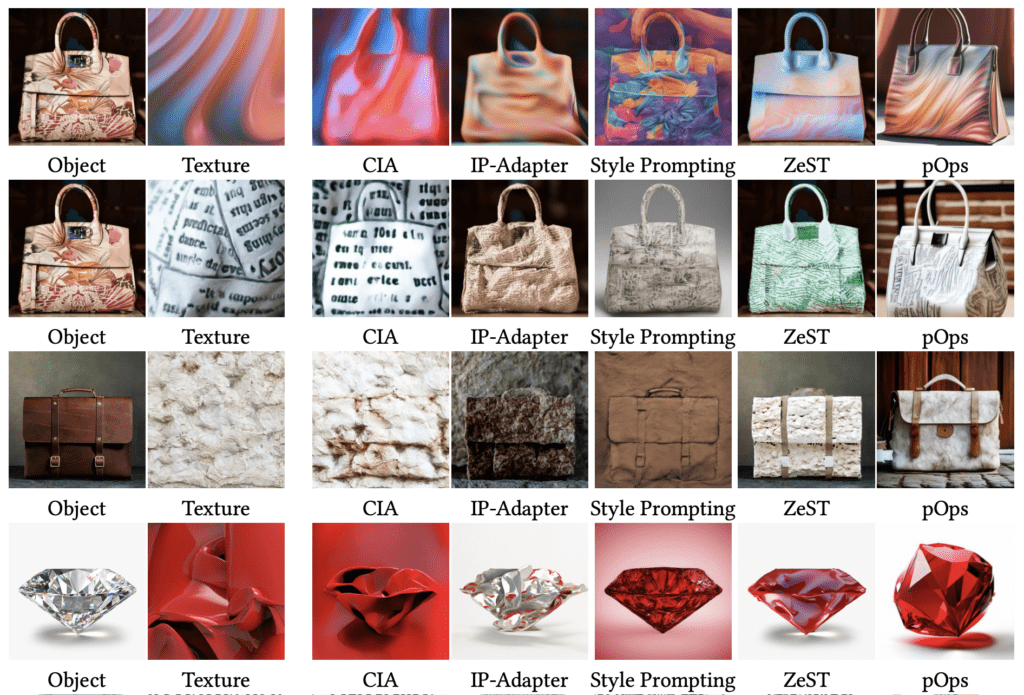
Looking ahead, further computational scaling and refined training techniques could improve pOps’ performance, even within the current limitations of the CLIP space. The development of more advanced operators and their integration into a unified model could pave the way for even more creative possibilities in the realm of visual content generation.
As the field continues to evolve, the introduction of frameworks like pOps underscores the importance of combining semantic richness with advanced computational techniques to push the boundaries of what’s possible in image generation.