Adversarial training reduces computational costs while maintaining high-quality video generation.
- Efficiency Boost: The new SF-V model achieves video generation in a single step, significantly speeding up the process.
- High-Quality Output: Despite the speed, the model maintains the quality of traditional multi-step diffusion models.
- Practical Applications: This advancement paves the way for real-time video synthesis and editing in various industries.
The landscape of video generation is undergoing a significant transformation with the introduction of the SF-V (Single Forward Video Generation) model. This novel approach promises to deliver high-fidelity videos with drastically reduced computational costs, leveraging adversarial training to fine-tune pre-trained video diffusion models. The breakthrough comes at a time when the demand for efficient and high-quality video synthesis is higher than ever, especially in fields such as entertainment, digital content creation, and beyond.
The Challenge of Computational Costs
Traditional diffusion-based video generation models, like the widely-used Stable Video Diffusion (SVD), operate through an iterative denoising process. While these models excel at creating photo-realistic frames with consistent motion, they come with a significant downside: high computational costs. Generating high-quality videos requires multiple denoising steps, which translates to longer processing times and higher resource consumption.
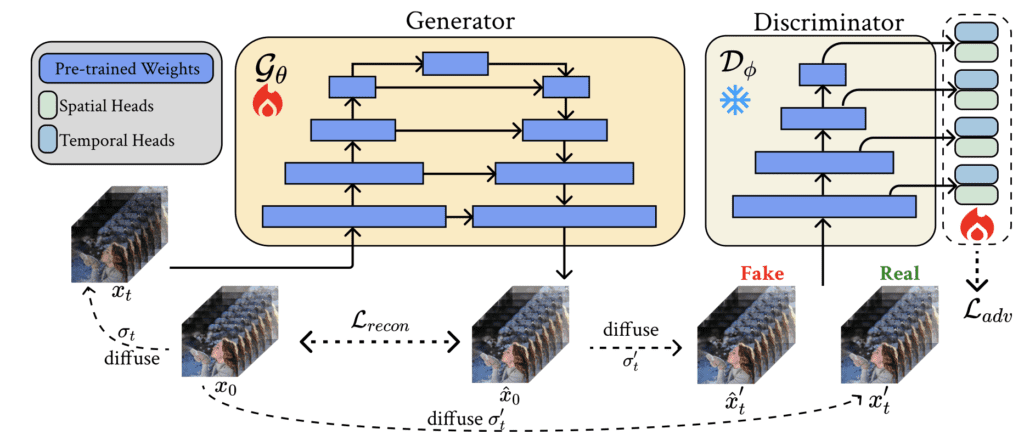
For instance, generating 14 frames using the UNet from the SVD model can take up to 10.79 seconds on an NVIDIA A100 GPU with a conventional 25-step sampling process. This substantial overhead limits the widespread and efficient deployment of these models, especially in real-time applications.
Enter SF-V: A Game-Changer
SF-V addresses these limitations head-on by reducing the denoising steps required for video generation. The model leverages adversarial training to fine-tune pre-trained video diffusion models, enabling a single forward pass to synthesize high-quality videos. This approach captures both temporal and spatial dependencies in video data, ensuring that the generated content remains coherent and visually appealing.
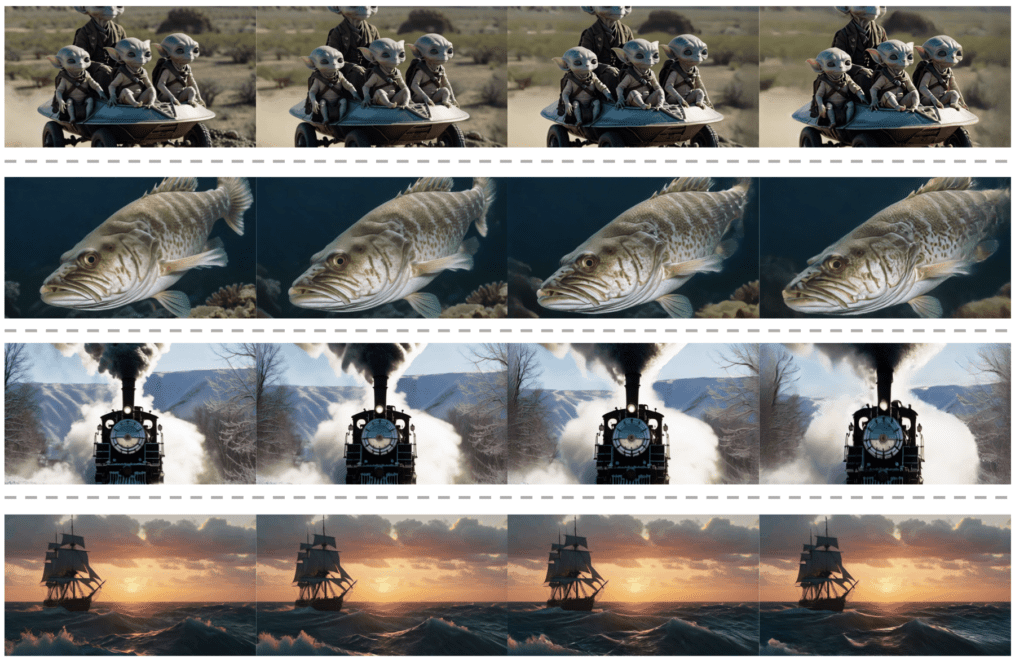
Efficiency Boost: SF-V achieves a remarkable 23× speedup compared to the traditional SVD model and a 6× speedup over existing methods, without compromising on the quality of the output. This efficiency opens up new possibilities for real-time video synthesis and editing, making advanced video generation accessible to a broader audience.
High-Quality Output: Extensive experiments have demonstrated that SF-V can produce videos that match or even surpass the quality of those generated by multi-step models. By introducing spatial-temporal heads in the discriminator, the model enhances video quality and motion diversity, maintaining a high standard of visual fidelity.
Practical Applications: The implications of this breakthrough are vast. From animating images with motion priors to generating videos from natural language descriptions, SF-V can be applied across a range of video generation tasks. This technology is particularly valuable for creating cinematic, temporally consistent videos, potentially transforming industries such as film production, advertising, and social media content creation.
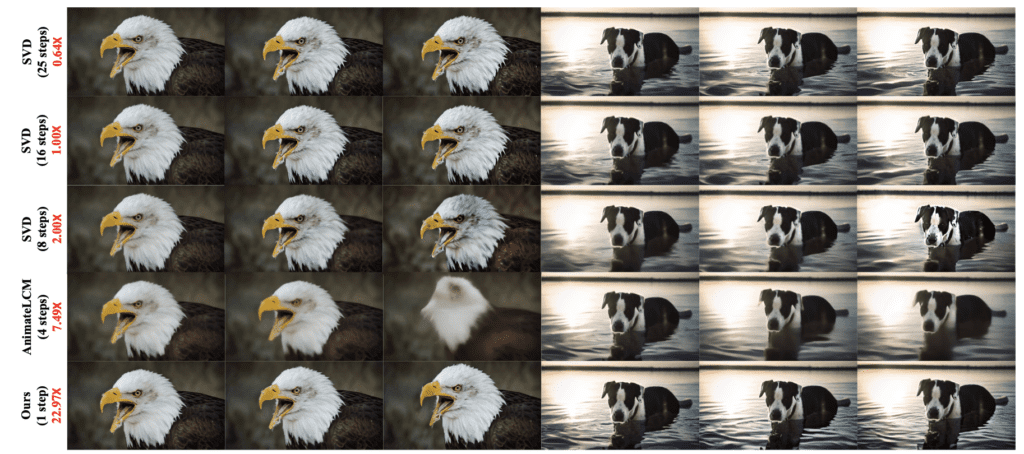
Overcoming Challenges
While SF-V represents a significant advancement, the journey is not without its challenges. One limitation noted is the considerable runtime required by the temporal VAE decoder and the encoder for image conditioning. Addressing these components will be crucial for further optimizing the overall runtime of the model.
Future Directions
The success of SF-V in achieving single-step video generation marks a pivotal moment in the evolution of diffusion models. Future research will focus on accelerating the temporal VAE decoder and image conditioning encoder, as well as exploring more efficient training techniques. Scaling this model with larger, high-quality video datasets could further enhance its capabilities, making it an even more powerful tool for video generation.
SF-V sets a new standard in video generation, demonstrating that it is possible to eliminate MatMul operations and still produce high-quality videos. By significantly reducing computational costs, this model makes real-time video synthesis and editing a reality. As the demand for efficient, high-quality video generation continues to grow, SF-V paves the way for innovative applications and broader accessibility in the world of digital content creation.