How the New AI Model Balances Text and Visual Inputs for Superior Results
- EMMA integrates multi-modal prompts, combining text with additional visual cues for image generation.
- The model maintains high fidelity and detail, surpassing the capabilities of existing methods.
- EMMA’s adaptable framework allows for seamless integration into various existing systems.
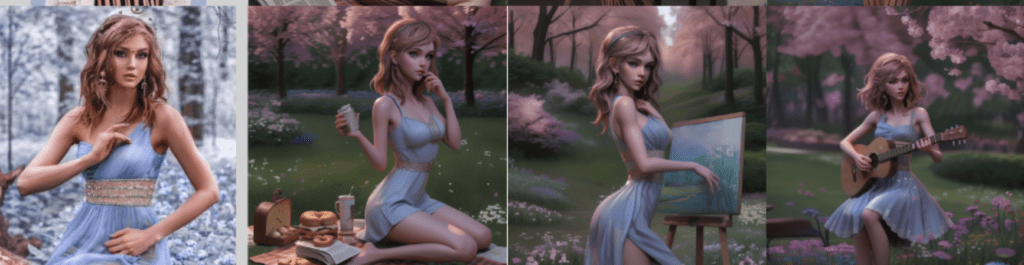
The landscape of image generation has seen remarkable advancements in recent years, driven by breakthroughs in AI and machine learning. Building on this momentum, researchers have introduced EMMA, a cutting-edge image generation model capable of accepting multi-modal prompts. This innovation promises to push the boundaries of how we create and interact with visual content, blending text inputs with other visual cues to produce highly detailed and contextually rich images.
The Challenge of Multi-Modal Prompts
Traditional text-to-image (T2I) models like DALLE-3 and Stable Diffusion 3 have set high standards for generating images from textual descriptions. However, these models often struggle when tasked with balancing multiple input modalities, such as text combined with reference images. Typically, they tend to prioritize one input over the others, leading to less optimal results.
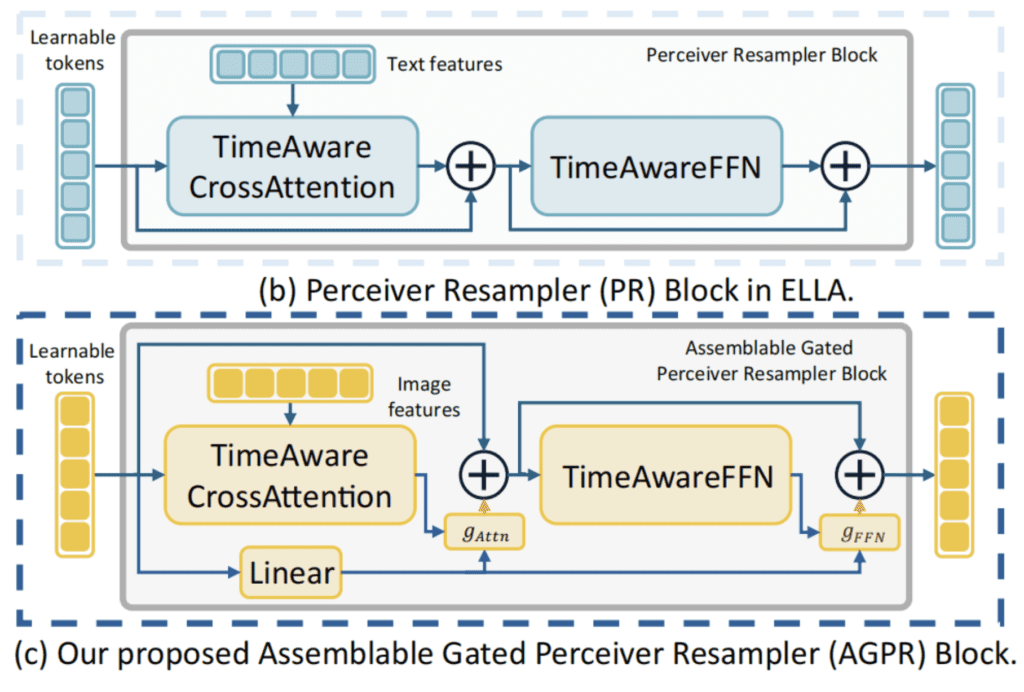
To address this challenge, EMMA introduces a novel approach that seamlessly integrates additional modalities alongside text. This is achieved through a Multi-modal Feature Connector, an innovative design that employs a special attention mechanism to harmonize textual and supplementary visual information.
The EMMA Approach
EMMA builds upon the existing ELLA T2I diffusion model, freezing its original parameters and adjusting additional layers to unlock the model’s potential to accept multi-modal prompts. This unique property allows EMMA to adapt easily to various existing frameworks, making it a flexible and powerful tool for personalized and context-aware image generation.
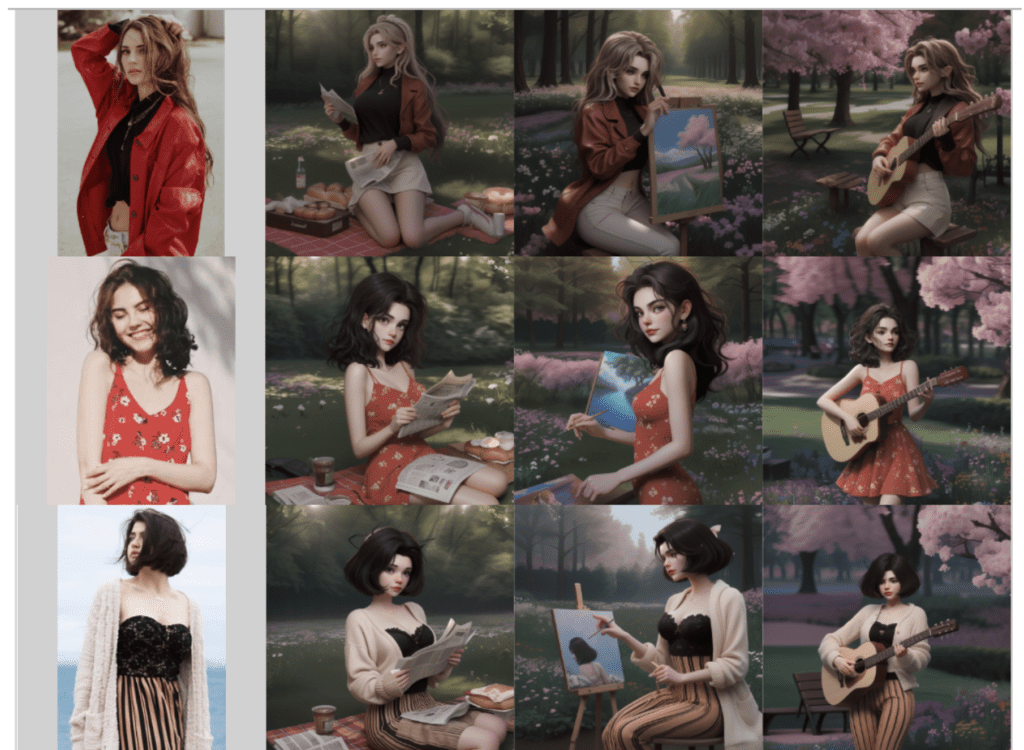
One of the standout features of EMMA is its ability to assemble learned modules to produce images conditioned on multiple modalities simultaneously, without the need for extra training with mixed prompts. This capability not only enhances the efficiency of the model but also broadens its applicability across different use cases.
Extensive Testing and Results
Through extensive experiments, EMMA has demonstrated its superiority over existing models in maintaining high fidelity and detail in generated images. Whether dealing with simple text prompts or complex multi-modal inputs, EMMA consistently delivers impressive results, showcasing its potential as a robust solution for advanced image generation tasks.
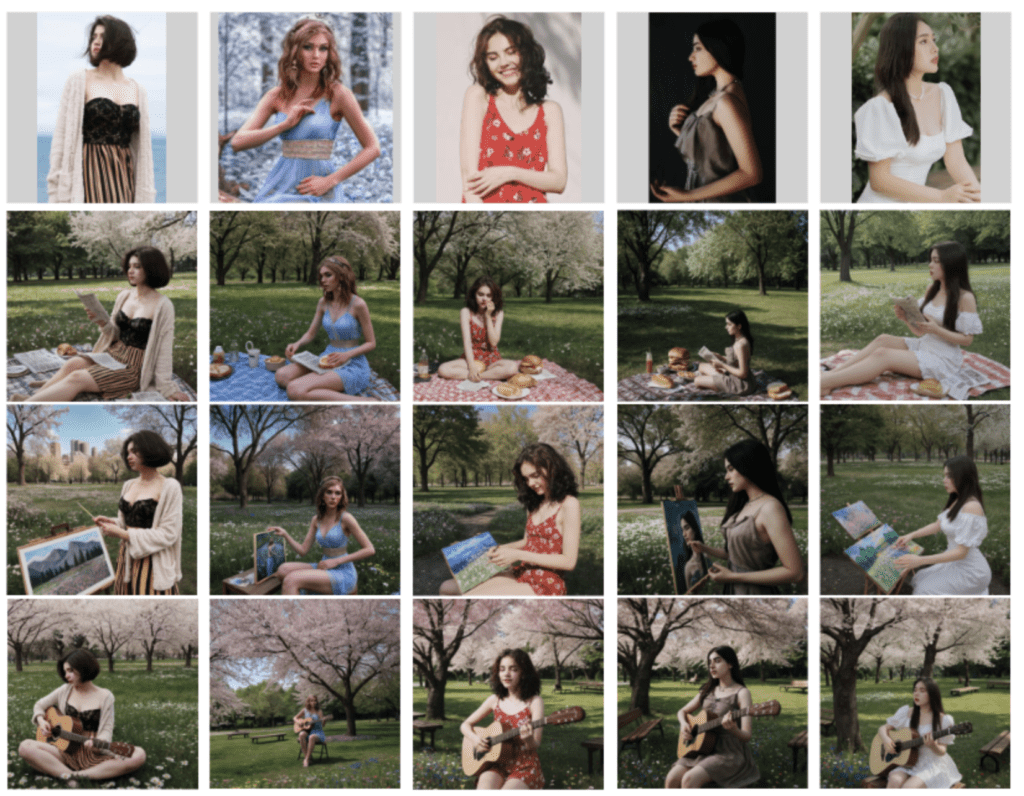
Applications and Future Directions
EMMA’s innovative approach opens up new possibilities for various applications. It can be used for subject-driven image generation, personalized image production, and even artistic portrait creation. The model’s flexibility and efficiency make it an ideal candidate for integration into different systems, enhancing the capabilities of existing technologies.
The development of EMMA marks a significant milestone in the field of generative models. Its ability to balance multiple input modalities sets a new benchmark for image generation, paving the way for more sophisticated and user-friendly AI-driven content creation tools.
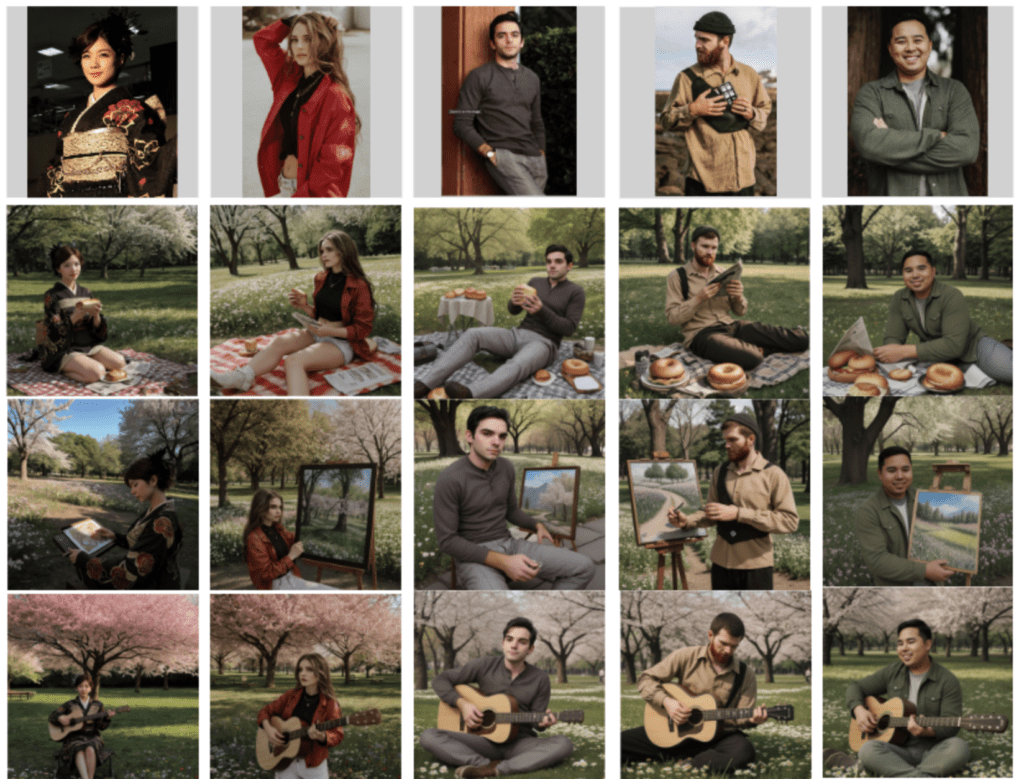
As the field of AI and machine learning continues to evolve, models like EMMA are poised to lead the next wave of innovation. By addressing the challenges of multi-modal prompts and setting new standards for image fidelity and detail, EMMA represents a significant advancement in the realm of AI-driven content creation. Its adaptable and efficient framework ensures that it will play a crucial role in the future of image generation, benefiting a wide range of applications and industries.