New method enhances simulated humanoid’s ability to grasp and transport varied objects
- Innovative Control Method: Introduces a controller for simulated humanoids to grasp and follow object trajectories.
- Broad Applicability: Demonstrates scalability across diverse objects and complex trajectories without extensive training datasets.
- Future Enhancements: Aims to improve trajectory following, grasping diversity, and integration with vision-based systems.
Researchers have made significant strides in the realm of simulated humanoid robotics, presenting a groundbreaking method that enables humanoids to grasp and transport a wide variety of objects along complex trajectories. This method addresses the limitations of previous approaches, which often relied on disembodied hands and constrained movements, by introducing a more holistic and versatile solution.
Innovative Control Method
The new method leverages a humanoid motion representation to imbue simulated humanoids with human-like motor skills, greatly accelerating the training process. Unlike prior techniques that focused on vertical lifts or short trajectories, this approach allows for the manipulation of over 1,200 objects, following randomly generated paths with high precision. By using only basic reward, state, and object representations, the method shows exceptional scalability and adaptability to diverse scenarios.
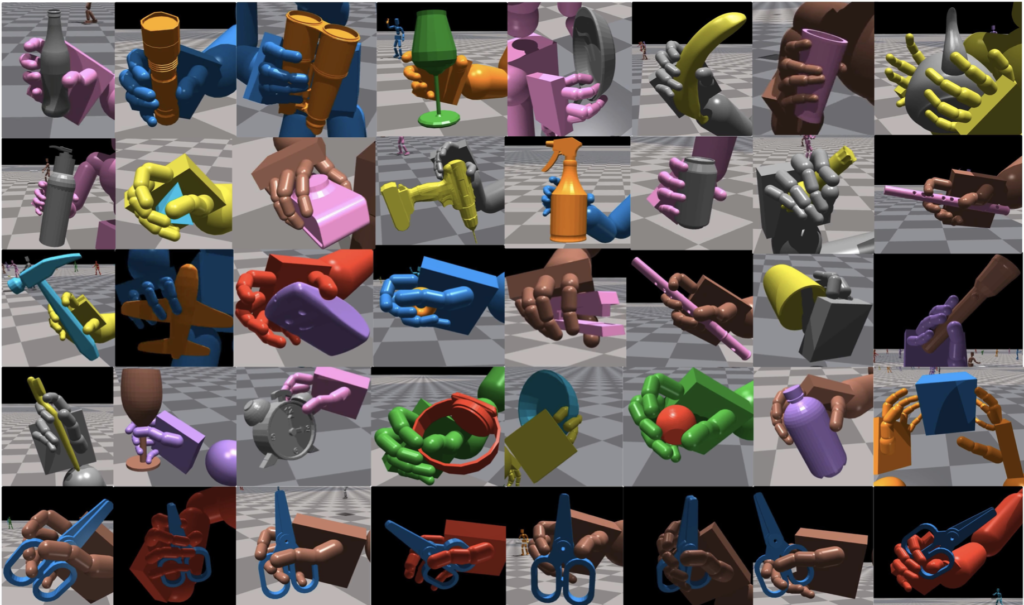
Broad Applicability
One of the standout features of this new approach is its broad applicability. Designed to enhance human-object interaction in animation, augmented reality (AR), virtual reality (VR), and potentially humanoid robotics, the method provides a robust solution for complex object manipulation tasks. The controller can handle various object shapes and sizes, ensuring stable grasps and accurate movements, even in dynamic and unpredictable environments.
At its core, the system integrates a universal humanoid motion representation that simplifies the learning process. This allows the humanoid to pick up objects and follow plausible trajectories without requiring extensive datasets of paired full-body motion and object interactions. The only inputs needed are the object mesh and the desired trajectories, making the method highly efficient and practical for a wide range of applications.
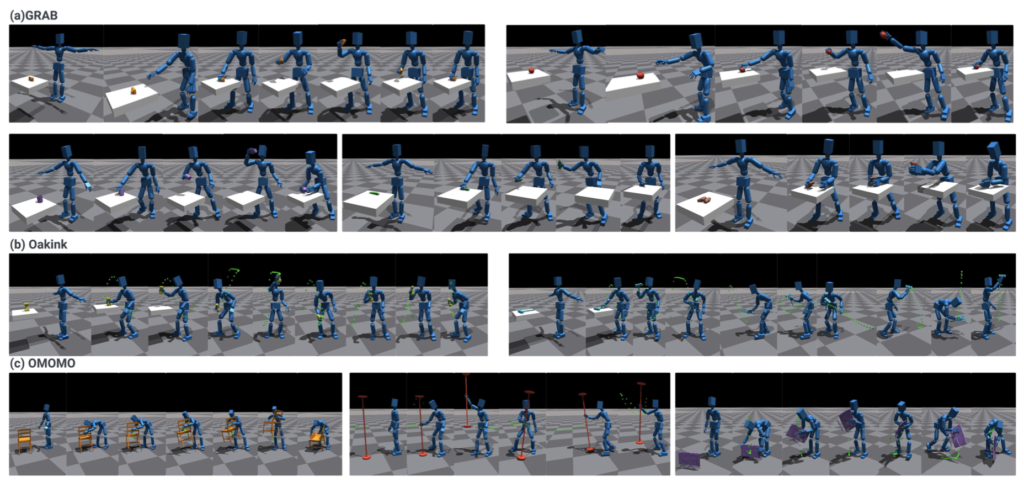
Future Enhancements
Despite its impressive capabilities, the method still has room for improvement. The researchers plan to enhance the success rate of trajectory following, increase the diversity of grasping techniques, and support a broader array of object categories. Additionally, they aim to refine the humanoid motion representation by potentially separating the motion representation for hands and body, which could lead to even greater control and precision.
Another promising direction for future research is the development of effective object representations that do not rely on canonical poses. This would allow the system to generalize better to vision-based inputs, further expanding its applicability and robustness. By continuously refining these aspects, the researchers hope to create a system that can seamlessly integrate into real-world applications, providing a versatile and powerful tool for various industries.
The introduction of this advanced method for controlling simulated humanoids marks a significant milestone in the field of embodied AI. By enabling humanoids to grasp and transport diverse objects along complex trajectories, the researchers have paved the way for more realistic and functional human-object interactions in digital and physical spaces. As they continue to refine and expand the capabilities of this system, the potential applications in animation, AR/VR, and robotics will only grow, offering exciting new possibilities for the future.