Advancing Realistic Human Insertion in Diverse Backgrounds
- Text2Place generates realistic human placements in various scenes using text guidance.
- The method utilizes semantic masks and subject-conditioned inpainting for accurate human insertion.
- Text2Place enables downstream applications like scene hallucination and text-based attribute editing.
In the field of computer vision, realistic human insertion into scenes, known as Semantic Human Placement, has long posed a significant challenge. Text2Place, a novel approach, addresses this by leveraging the capabilities of text-to-image generative models to create coherent and contextually accurate human placements in diverse backgrounds. This method offers a comprehensive solution for placing humans in scenes, preserving both the background and the identity of the inserted individuals.
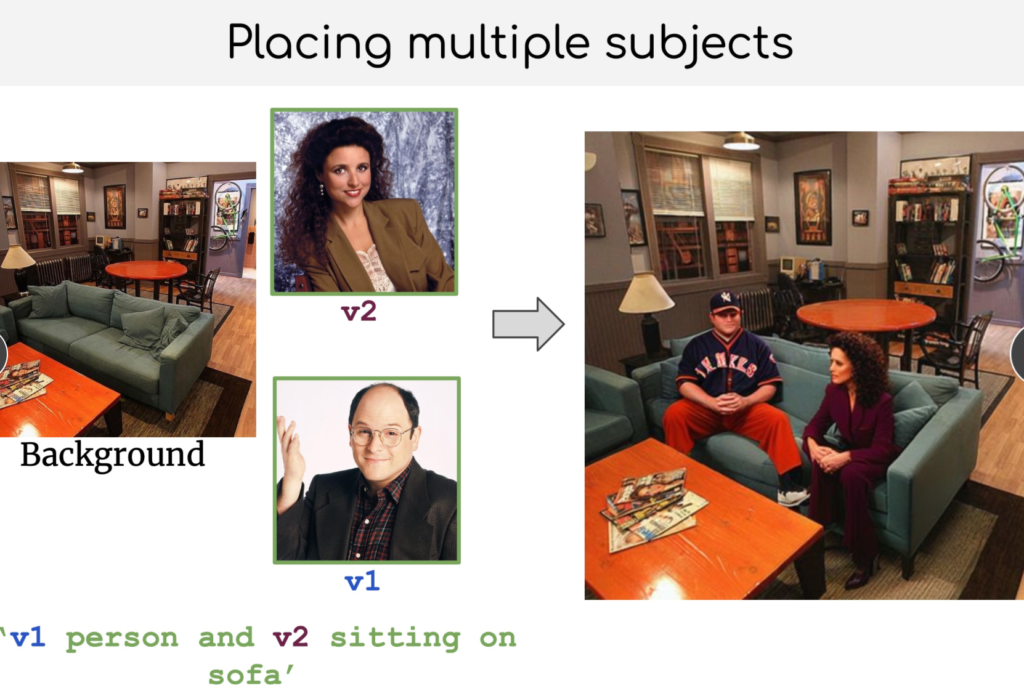
The Challenge of Semantic Human Placement
Semantic Human Placement involves generating human figures that seamlessly integrate into a given scene. This task is challenging due to the need to maintain consistency in scale, pose, and interaction with the environment, all while preserving the identity of the generated person. Previous methods have often been limited by the constraints of specific datasets or have required large-scale training to achieve reasonable results.
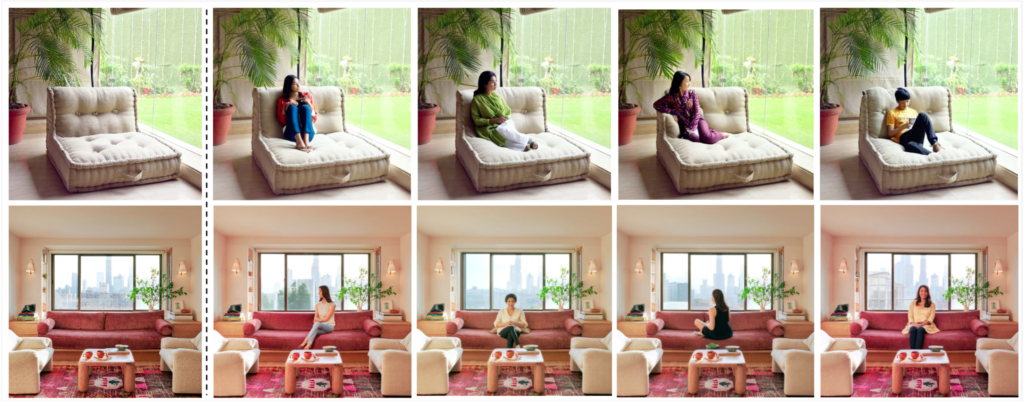
Text2Place Framework
Text2Place innovatively divides the problem into two stages:
- Learning Semantic Masks: The first stage involves creating semantic masks using text guidance. By leveraging rich object-scene priors learned from text-to-image generative models, Text2Place optimizes a novel parameterization of the semantic mask. This eliminates the need for extensive training and allows the model to localize regions in the image where humans can be placed.
- Subject-Conditioned Inpainting: In the second stage, Text2Place uses these semantic masks for subject-conditioned inpainting. This process ensures that the inserted human figures adhere to the scene’s affordances, resulting in realistic and contextually appropriate placements.
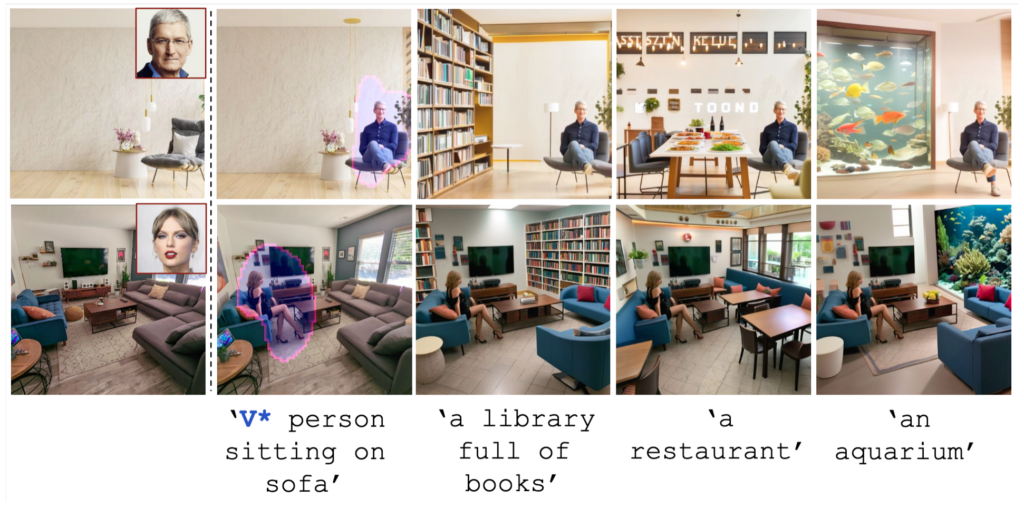
Achievements and Applications
Text2Place’s approach excels in generating highly realistic scene compositions. Extensive experiments have demonstrated its superiority over existing methods, particularly in terms of visual consistency, harmony, and reconstruction quality. The method enables several downstream applications, including:
- Scene Hallucination: Text2Place can generate realistic scenes with one or more human figures based on text prompts, enhancing the richness of the generated environments.
- Text-Based Attribute Editing: Users can modify the attributes of the generated humans through text prompts, allowing for dynamic and versatile scene compositions.
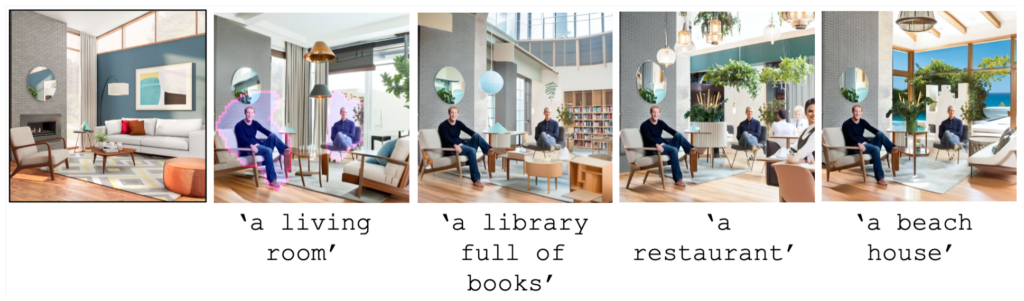
Technical Insights
Text2Place introduces the Stylized Equirectangular Panorama Generation pipeline, combining multiple diffusion models to create detailed equirectangular panoramas from complex text descriptions. This panoramic approach ensures a holistic initialization of the 3D scene, addressing the common issue of global inconsistency seen in outpainting-based methods.
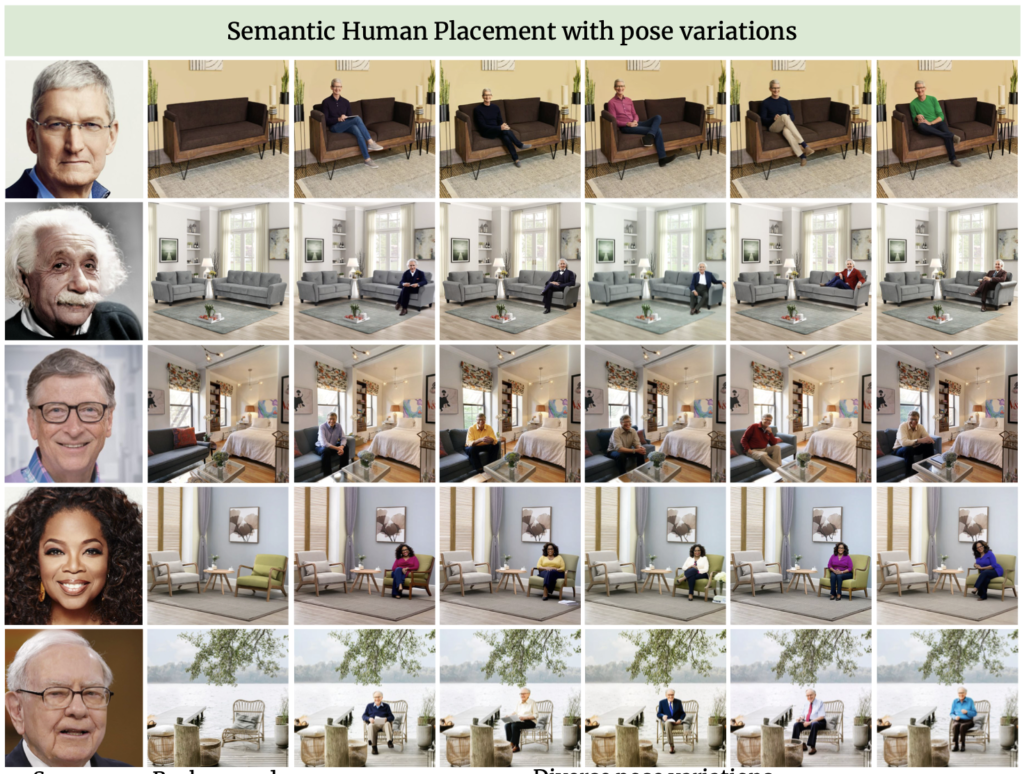
Additionally, the Enhanced Two-Stage Panorama Reconstruction refines the scene’s integrity. This method uses 3D Gaussian Splatting (3D-GS) for quick 3D reconstruction, followed by a second stage that introduces additional cameras to fill in missing regions. This two-stage optimization significantly enhances the rendering robustness and visual quality of the generated scenes.
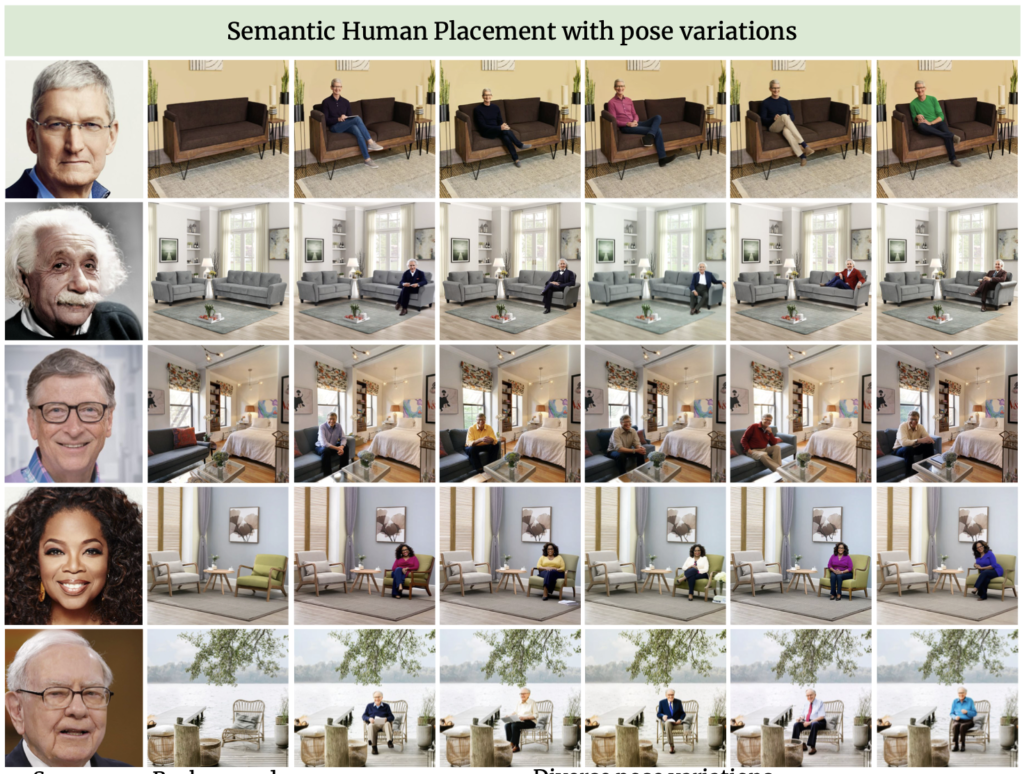
Future Directions and Challenges
While Text2Place marks a significant advancement in Semantic Human Placement, several challenges persist:
- Data Scarcity: The availability of panoramic image data is limited compared to perspective image data, which poses a challenge for processing more complex text descriptions during generation.
- Optimization Efficiency: The method currently limits itself to a two-stage reconstruction process. Future work could explore increasing the number of iterative inpainting stages and optimizing camera setup strategies to further enhance scene integrity and rendering robustness.
- Error Compounding: Combining multiple diffusion models can introduce compounded errors and increase stochastic variability. Refining the integration methods will be crucial for improving overall consistency.
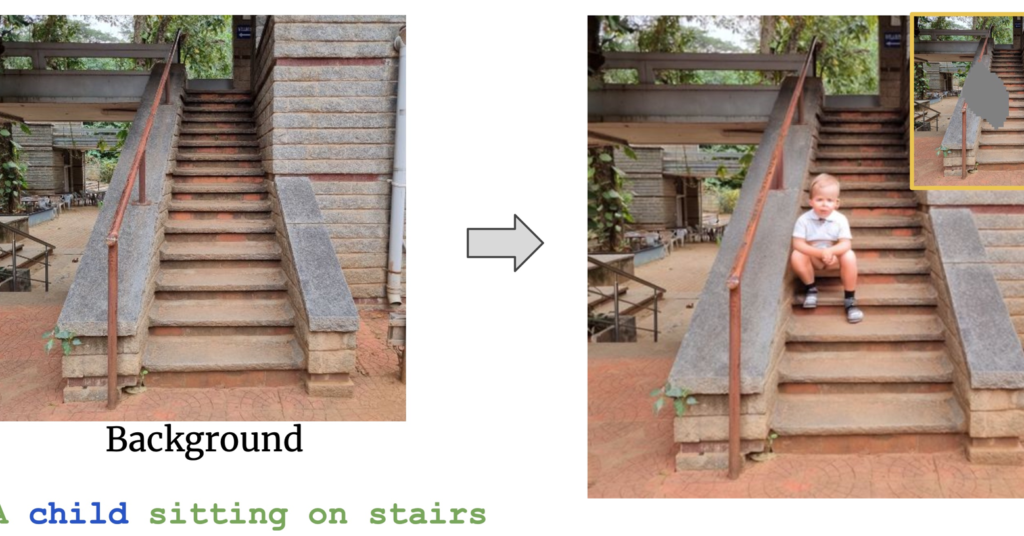
Text2Place offers a robust and innovative solution for text-guided human placement in diverse scenes. By leveraging advanced text-to-image generative models and optimizing semantic masks, it achieves highly realistic and contextually accurate human insertions. The method’s ability to handle various downstream applications, including scene hallucination and text-based attribute editing, further underscores its versatility and potential impact on the field of computer vision.
As the technology continues to evolve, Text2Place could significantly enhance applications in virtual reality, gaming, and film, making the creation of immersive digital environments more accessible and sophisticated than ever before.