Enhancing Robot Learning with Rich Visual Representations
- Theia leverages multiple vision foundation models to improve robot learning.
- The model outperforms previous approaches with less training data and smaller sizes.
- High entropy in feature norm distributions correlates with better robot learning performance.
Vision-based robot learning, which involves mapping visual inputs to actions, requires a comprehensive understanding of various visual tasks beyond single-task needs like classification or segmentation. Addressing this need, Theia is a new vision foundation model designed for robot learning. Theia stands out by distilling multiple off-the-shelf vision foundation models (VFMs) trained on diverse visual tasks. This approach results in rich visual representations that encode a wide range of visual knowledge, significantly enhancing the performance of downstream robot learning tasks.
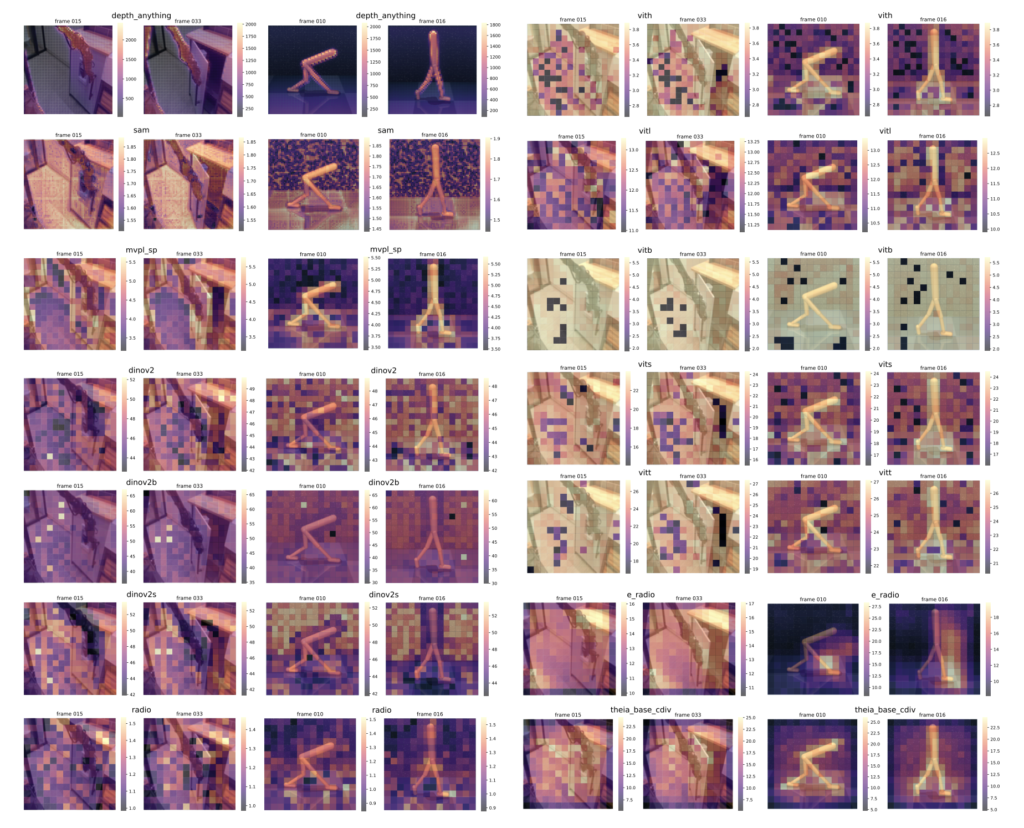
Development of Theia
Theia’s development was motivated by the limitations of existing models, which often focus on specific visual tasks. By combining multiple VFMs, Theia creates a more holistic and versatile visual representation. This method not only improves the model’s ability to understand and interpret complex scenes but also allows it to perform better with less training data and smaller model sizes compared to its predecessors.
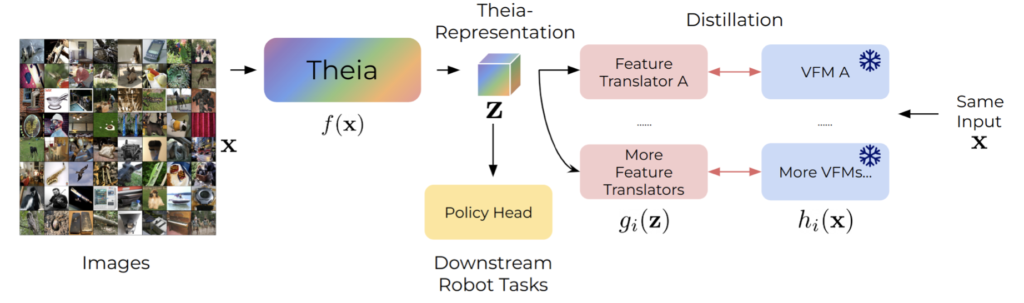
Performance and Experiments
Extensive experiments were conducted to evaluate Theia’s performance. The results showed that Theia consistently outperformed its teacher models and previous robot learning models. This was evident in both simulation environments like MuJoCo and real-world scenarios. One of the key metrics for this evaluation was the entropy of feature norm distributions, which played a crucial role in understanding why certain visual representations led to better robot learning outcomes.
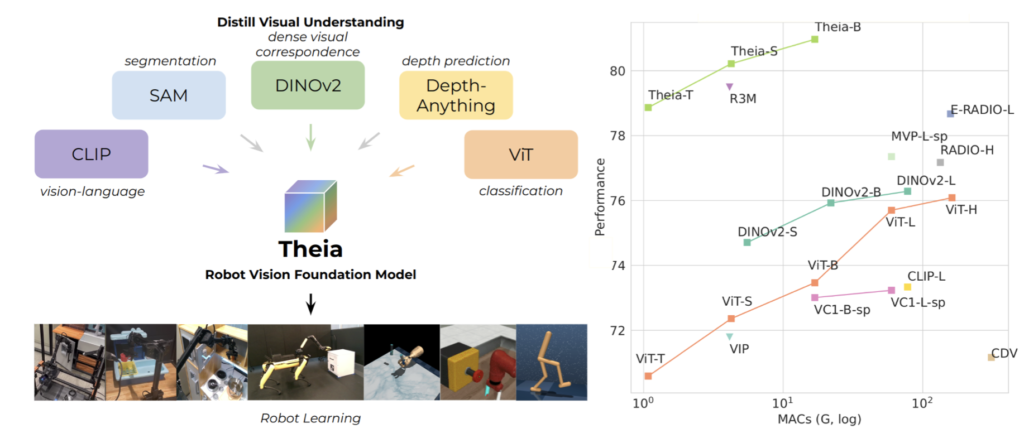
Feature Norm Distributions and Entropy
The research delved into the quality of visual representations by analyzing feature norm distributions. High-norm outlier tokens, which are detrimental to vision task performance, were found to be minimal or non-existent in Theia. In contrast, other models exhibited these outliers, affecting their efficiency. The study found a strong correlation between higher entropy in feature norm distributions and improved robot learning performance. This means that spatial token representations with high entropy, indicating better feature diversity, encode more information that aids in policy learning.
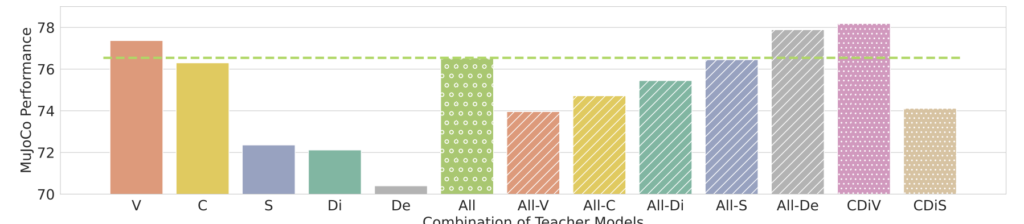
Practical Applications and Future Directions
Theia has practical implications for various domains, including virtual reality, gaming, and film, where 3D scene generation and realistic human insertion are critical. By enhancing the visual comprehension of robots, Theia can significantly improve the interaction between robots and their environments, making them more effective in performing complex tasks.
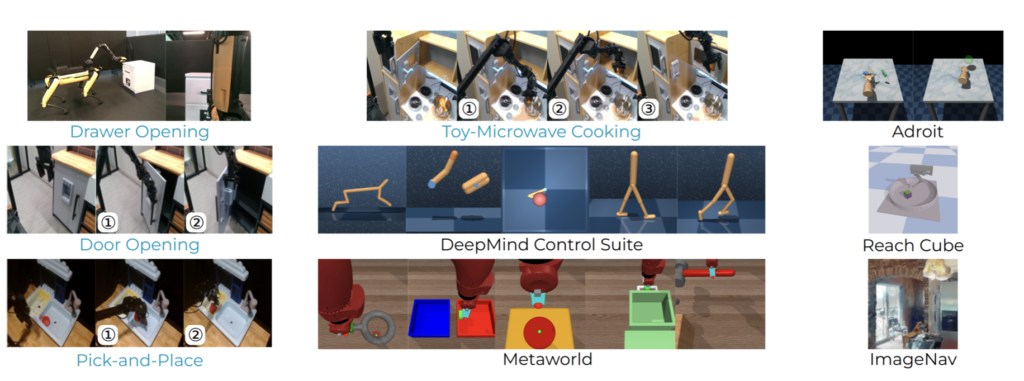
Theia represents a significant advancement in the field of robot learning, offering a comprehensive solution that combines the strengths of multiple VFMs into a single, efficient model. The research highlights the importance of feature norm entropy in visual representations, providing valuable insights for future developments in this area. As the demand for more sophisticated and efficient robot learning models grows, Theia sets a new benchmark for what can be achieved through the innovative distillation of diverse vision foundation models.
