Introducing VidGen-1M, a breakthrough dataset designed to enhance text-to-video generation models
- VidGen-1M addresses the shortcomings of existing video-text datasets.
- It ensures high video quality, detailed captions, and excellent temporal consistency.
- Models trained on VidGen-1M outperform state-of-the-art text-to-video models.
The development of high-quality text-to-video generation models has long been hindered by the limitations of available datasets. Current datasets suffer from issues like low temporal consistency, poor-quality captions, substandard video quality, and imbalanced data distribution. To overcome these challenges, researchers have introduced VidGen-1M, a new large-scale dataset meticulously curated to set a new standard in the training of text-to-video generation models.
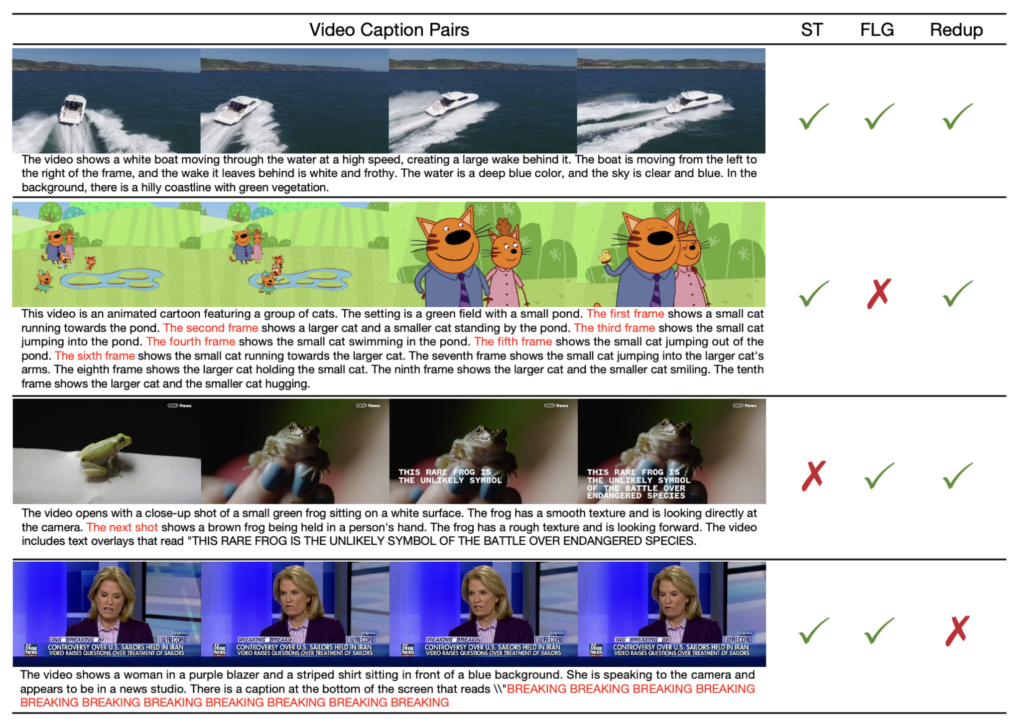
VidGen-1M emerges as a solution to the pervasive issues in video-text datasets. Traditional curation processes often rely on image models for tagging and manual rule-based methods, resulting in high computational loads and unclean data. VidGen-1M employs a coarse-to-fine curation strategy to ensure that each video-text pair is of the highest quality. This approach not only enhances video quality and caption detail but also significantly improves temporal consistency and video-text alignment.
To validate the effectiveness of VidGen-1M, a text-to-video generation model was trained using this dataset. The results were impressive, with the model achieving significantly better FVD (Fréchet Video Distance) scores on the zero-shot UCF101 benchmark compared to state-of-the-art models. This demonstrates VidGen-1M’s potential to boost the performance of text-to-video generation models, making it a valuable resource for researchers and developers in the field.
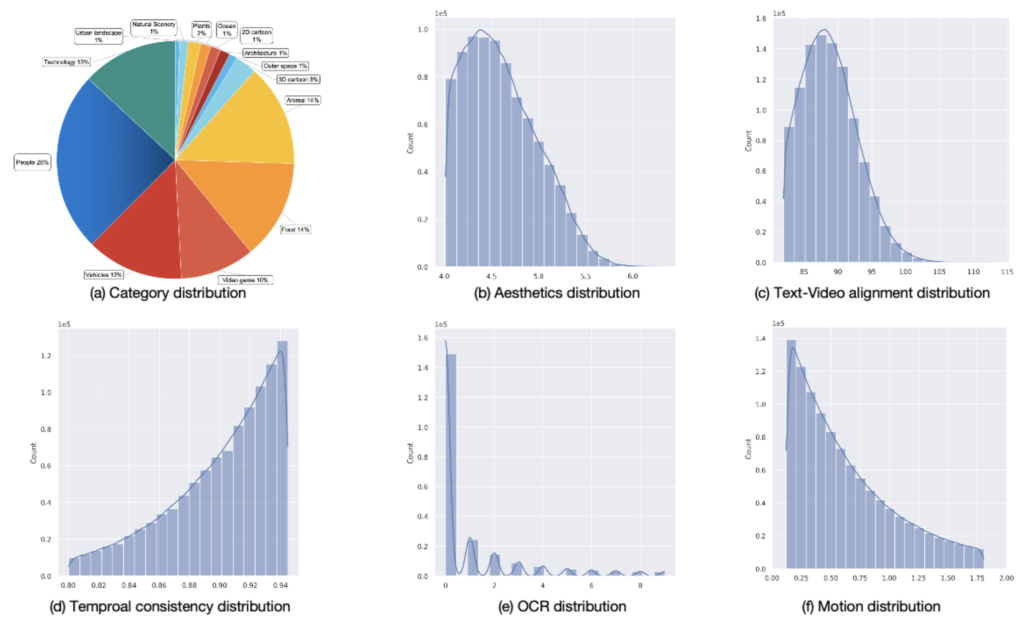
VidGen-1M stands out due to its:
- High Video Quality: The dataset includes high-resolution videos that are free from common defects found in other datasets.
- Detailed Captions: Each video is paired with comprehensive and contextually accurate captions, ensuring robust text-video alignment.
- Temporal Consistency: The dataset maintains high temporal consistency across video frames, a critical factor for generating coherent and realistic videos.
The dataset’s creation involved a rigorous curation process that meticulously filtered and selected video-text pairs to meet these high standards. By ensuring quality at every step, VidGen-1M sets a new benchmark for datasets in this domain.
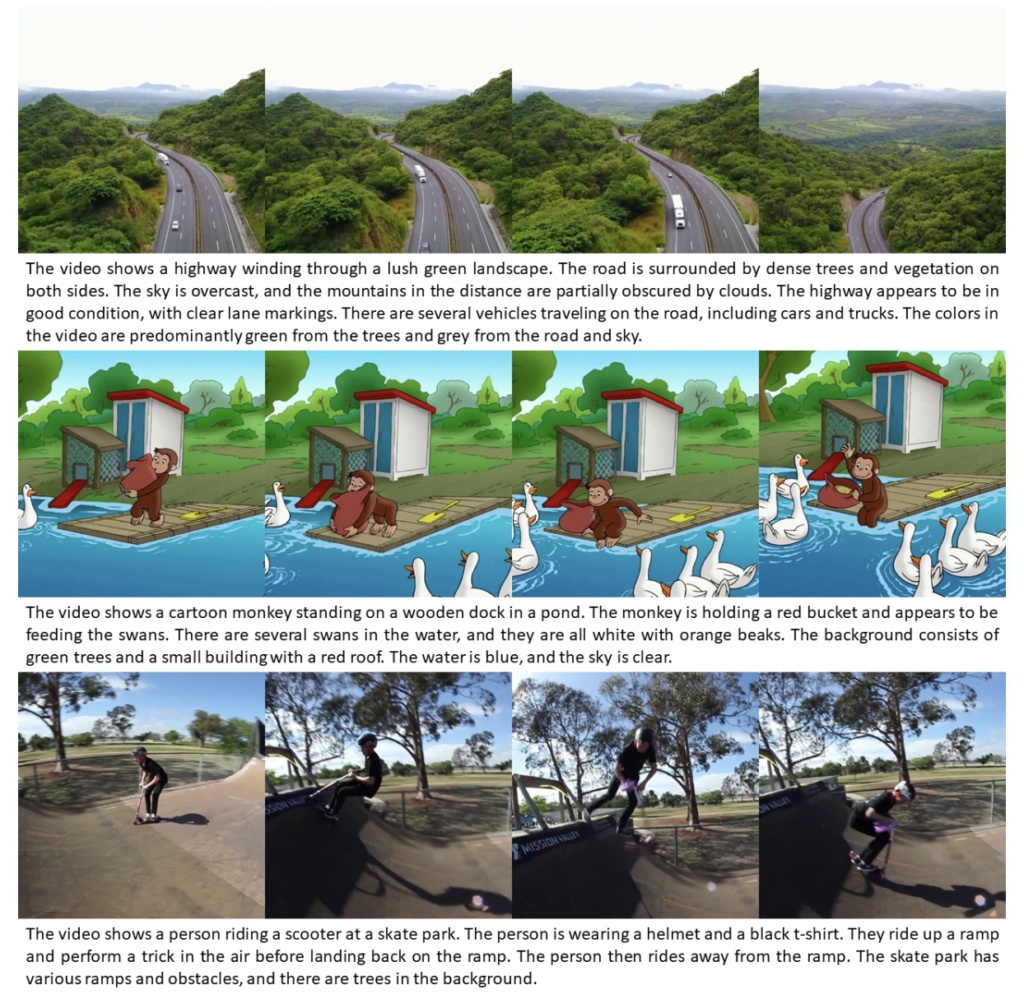
Moreover, to facilitate further advancements in video generation, VidGen-1M, along with the associated codes and the models trained on it, will be made publicly available. This open access is expected to spur innovation and development in text-to-video generation, providing researchers with a robust foundation to build upon.
In conclusion, VidGen-1M addresses the fundamental issues plaguing current video-text datasets and offers a superior alternative for training text-to-video generation models. Its release promises to enhance the capabilities of AI in creating high-quality, coherent, and contextually accurate videos from textual descriptions, pushing the boundaries of what is possible in this exciting field.