New Framework and Evaluation Metrics Illuminate VLM Selection Across Diverse Tasks and Domains
- Rise of Visual Question-Answering: Visual Question-Answering (VQA) has gained prominence in enhancing user experiences, particularly with the success of Vision-Language Models (VLMs) in zero-shot inference tasks.
- Challenges in Model Evaluation: Despite advancements, evaluating different VLMs for specific application needs remains a complex challenge due to the lack of standardized frameworks.
- Introducing GoEval: This article presents a new comprehensive framework for evaluating VLMs tailored to VQA tasks, along with the introduction of GoEval, a multimodal evaluation metric that aligns closely with human judgments.
The field of Visual Question-Answering (VQA) has become increasingly vital in a variety of applications, from customer support to educational tools. As Vision-Language Models (VLMs) demonstrate remarkable capabilities in zero-shot inference, the need for a structured approach to evaluate these models has never been more urgent. This paper introduces a comprehensive framework aimed at guiding the selection of VLMs for VQA tasks, facilitating more effective use of these technologies in practical settings.
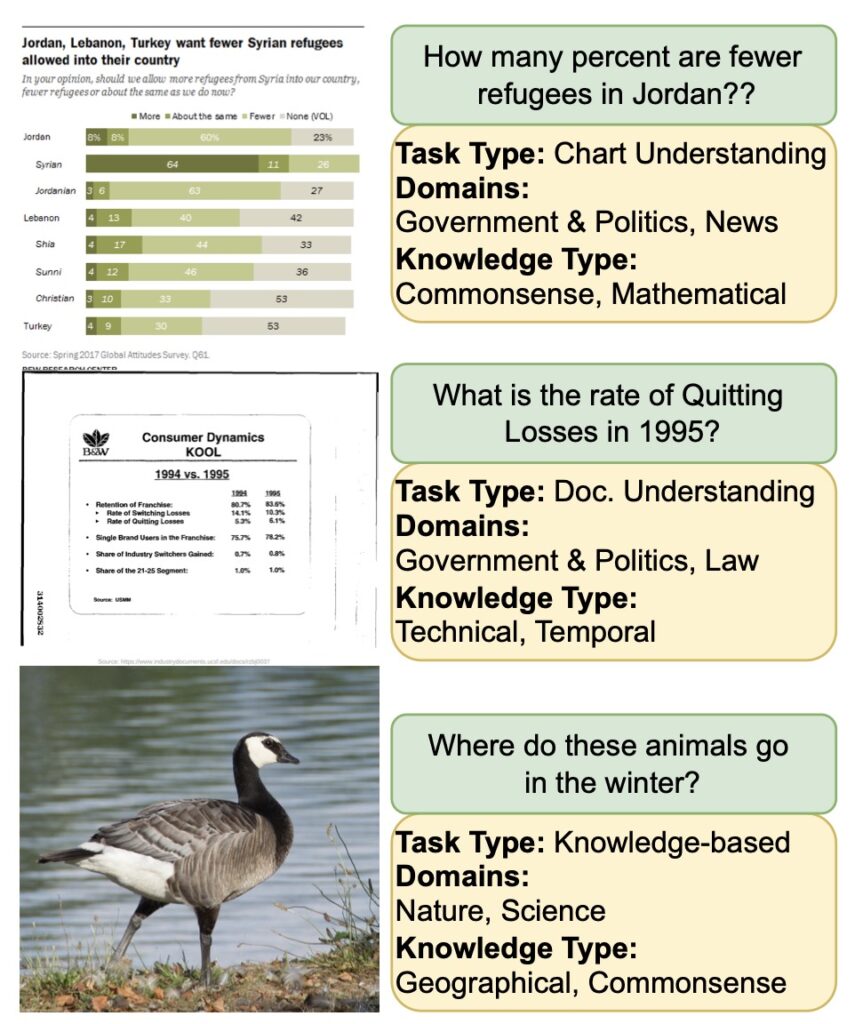
The proposed framework categorizes VQA tasks based on three essential dimensions: task types, application domains, and knowledge types. By structuring the evaluation process around these dimensions, practitioners can better match VLMs to their specific requirements. To support this framework, a novel dataset derived from established VQA benchmarks has been created, featuring annotations for four task types, 22 application domains, and 15 knowledge types. This rich dataset serves as a foundation for rigorous comparisons of different VLMs.
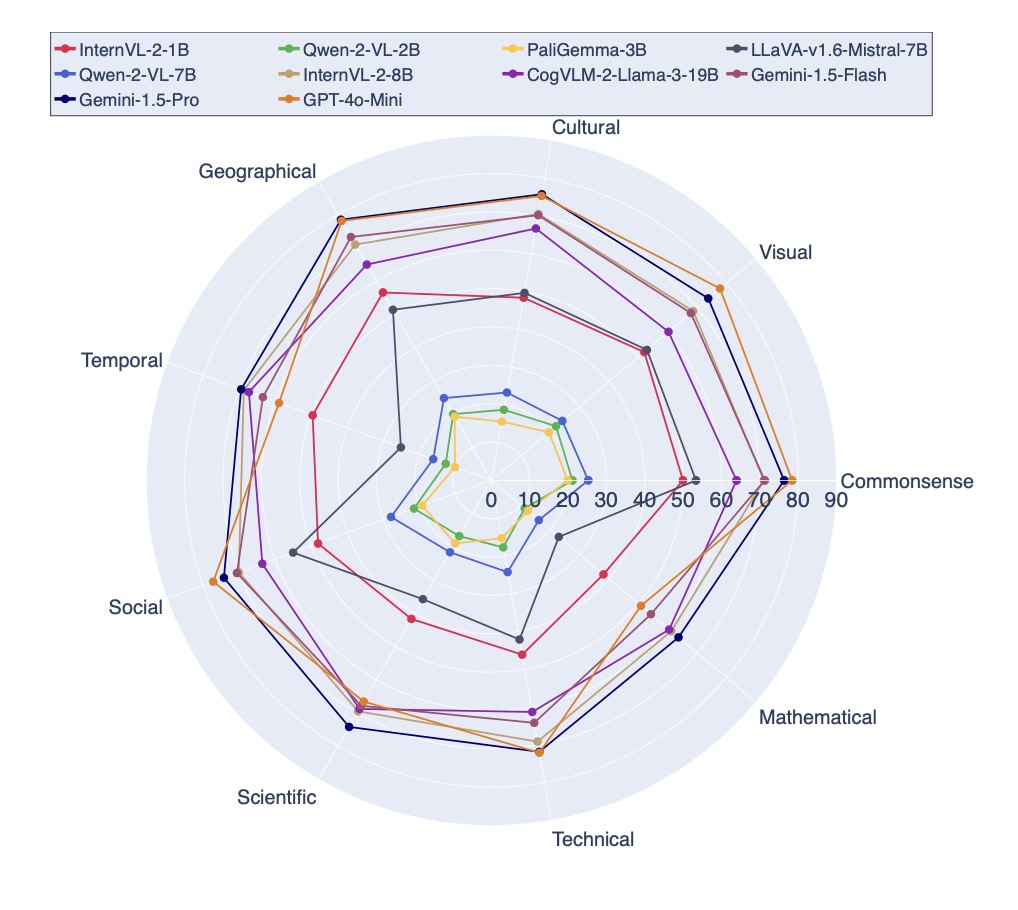
A key highlight of this research is the introduction of GoEval, a new evaluation metric designed using GPT-4o. With a correlation factor of 56.71% with human judgments, GoEval offers a more nuanced understanding of VLM performance compared to traditional metrics. By leveraging both visual and textual information, GoEval enhances the evaluation process, allowing for better insights into how VLMs perform in various scenarios.
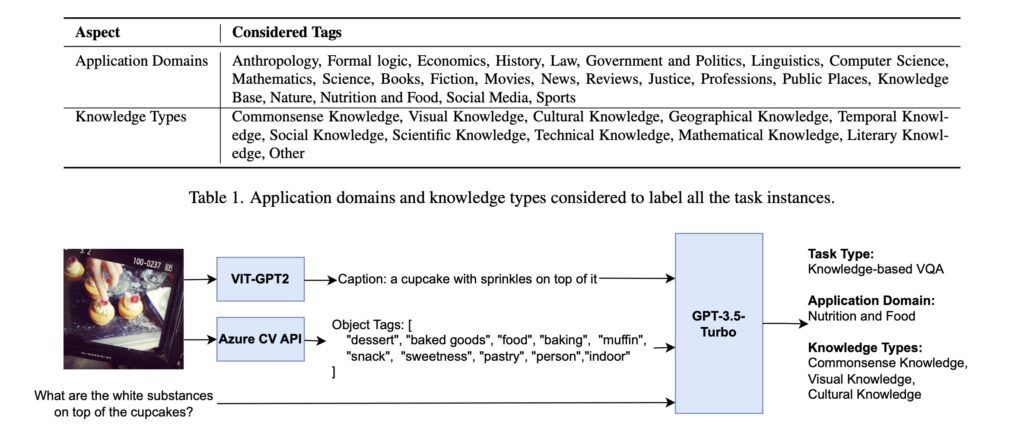
Experimental results reveal that no single VLM excels universally across all categories. Proprietary models like Gemini-1.5-Pro and GPT-4o-mini often outperform others in general metrics, yet open-source models such as InternVL-2-8B and CogVLM-2-Llama-3-19B show competitive strengths in specific contexts. This variability underscores the importance of selecting the right model based on task-specific requirements and resource constraints, providing practitioners with practical insights for optimal VLM selection.
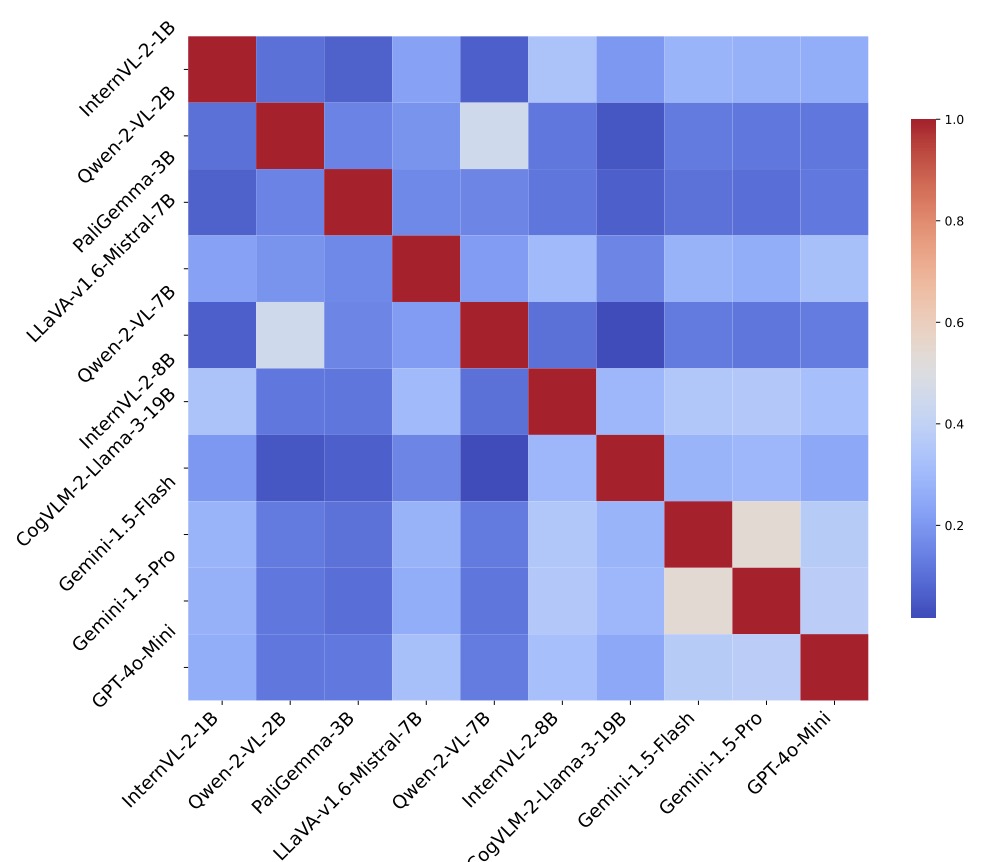
The implications of this study extend beyond VQA. The established evaluation framework can be applied to other vision-language tasks, promoting advancements in multimodal research. As AI continues to evolve, having robust methods for assessing model performance will be essential in leveraging these technologies effectively across a variety of domains.
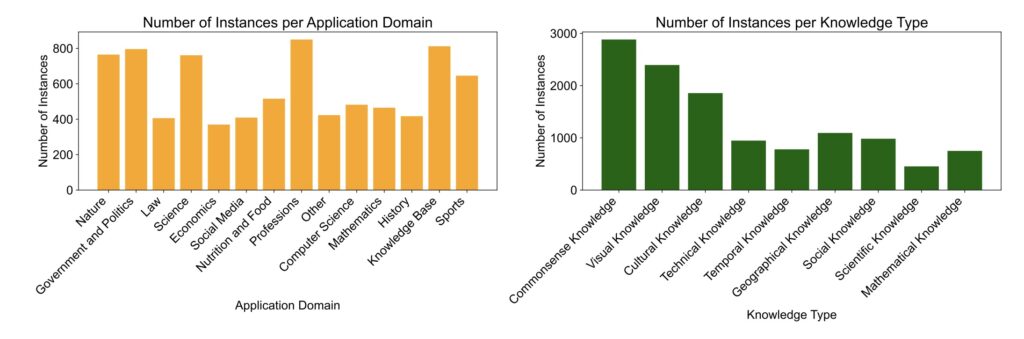
The introduction of a comprehensive framework for evaluating Vision-Language Models marks a significant step forward in the VQA landscape. By utilizing the new GoEval metric and focusing on key task dimensions, researchers and practitioners can make informed decisions about which models to deploy. As VQA and multimodal applications grow, these insights will be crucial in advancing the capabilities and effectiveness of AI technologies in real-world scenarios.
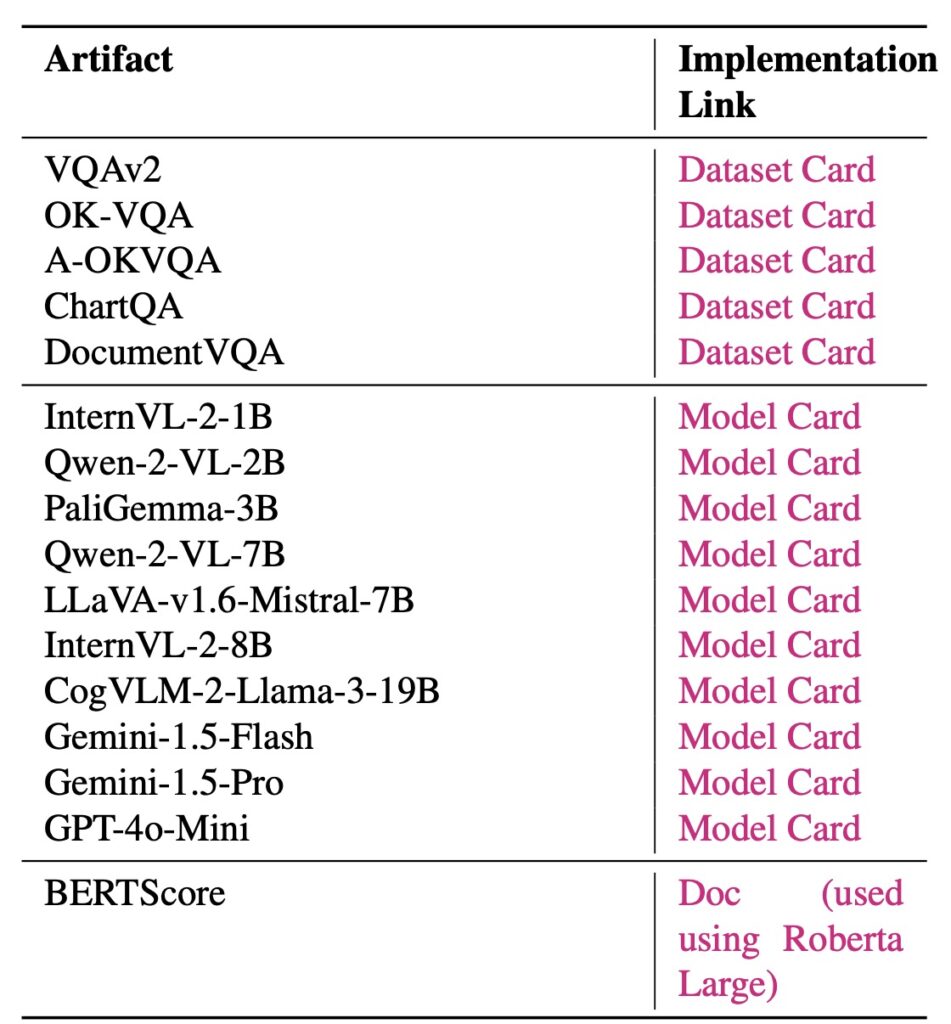