Assessing the Next Frontier in Visual Language Models for Real-World Applications
- Understanding Abductive Reasoning: NL-EYE adapts the abductive Natural Language Inference (NLI) task to the visual domain, challenging models to evaluate the plausibility of hypotheses based on images and explain their reasoning.
- Diverse Reasoning Categories: The benchmark comprises 350 carefully curated triplet examples, encompassing various reasoning categories, including physical, functional, logical, emotional, cultural, and social contexts.
- Highlighting VLM Limitations: Experimental results reveal that current VLMs struggle with abductive reasoning, often performing at random levels, while humans excel, indicating a significant gap in capabilities that future models need to address.
Google’s NL-EYE benchmark represents a pivotal moment in the evolution of Visual Language Models (VLMs), focusing on their ability to perform abductive reasoning—a skill that is essential for practical applications in fields such as accident prevention and video verification. While recent advancements in VLMs have demonstrated remarkable capabilities in visual and textual tasks, their ability to infer causes and outcomes has not been sufficiently explored. By addressing this gap, NL-EYE aims to push the boundaries of what VLMs can achieve in understanding complex visual information.
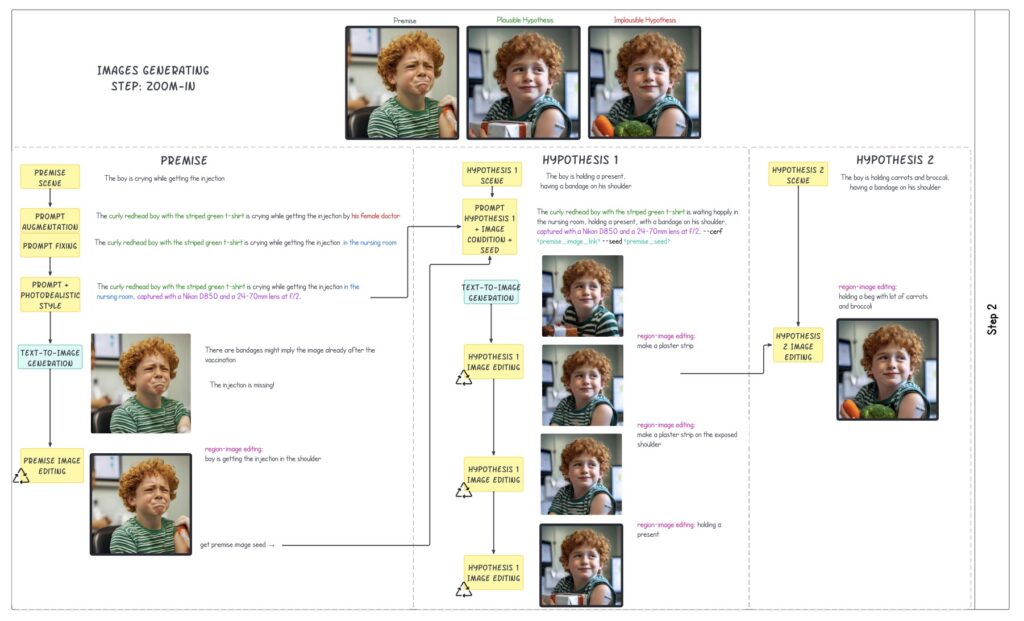
The benchmark consists of 350 triplet examples, totaling 1,050 images, that span multiple reasoning categories. The curation process involved not only generating images using advanced text-to-image models but also writing detailed textual descriptions that challenge VLMs to think critically about the scenarios presented. This rigorous process ensured that the benchmark is not only high-quality but also presents a significant challenge to existing models. The diverse categories of reasoning allow for a comprehensive assessment of a VLM’s capabilities, moving beyond simple visual recognition to deeper cognitive processes.
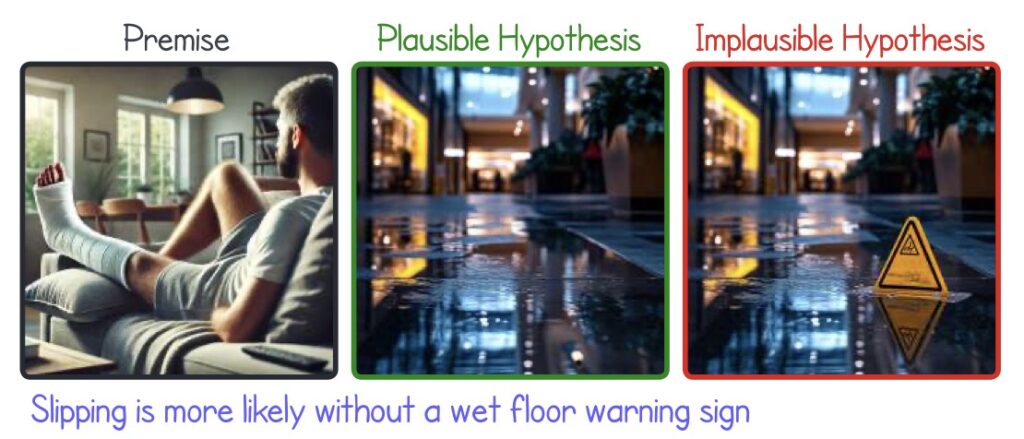
Initial experiments with NL-EYE have revealed some concerning limitations in the performance of current VLMs. While humans consistently excel in predicting the plausibility of hypotheses and providing coherent explanations, the VLMs often perform at random baseline levels. This discrepancy highlights a fundamental deficiency in the ability of these models to integrate visual interpretation with logical reasoning. The struggle of VLMs to provide consistent and useful explanations further underscores the need for more advanced architectures that can mimic human cognitive processes in complex environments.
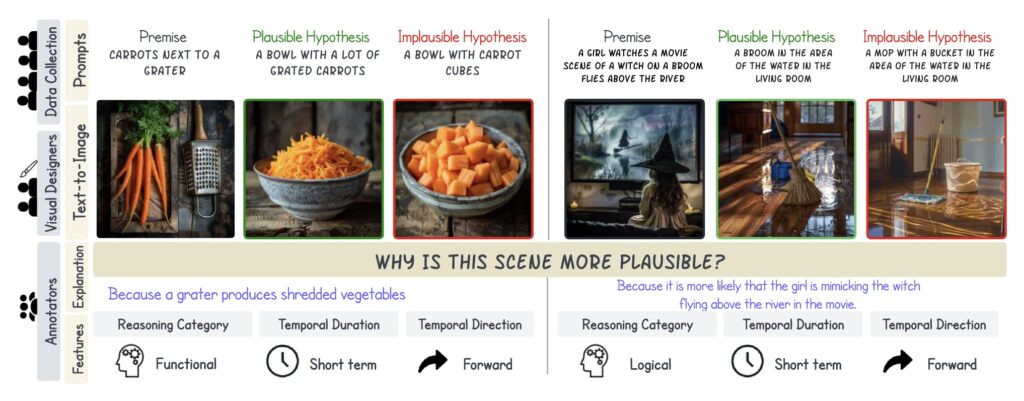
The implications of NL-EYE extend beyond academic interest; they point to the urgent need for improvements in VLM technology to facilitate real-world applications. For instance, consider a potential accident-prevention bot that could analyze visual scenarios and predict hazards, such as detecting a wet floor and alerting users. The ability to accurately assess such situations relies heavily on the model’s abductive reasoning capabilities, making advancements in this area crucial for deploying effective and reliable AI solutions.
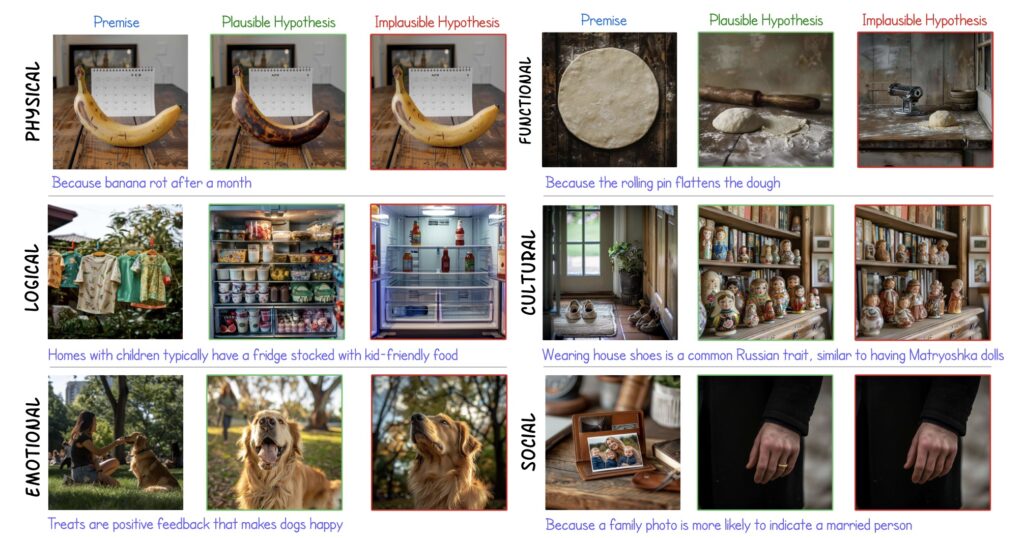
Looking ahead, the team behind NL-EYE is committed to addressing the gaps identified in current VLMs. The insights gained from this benchmark will inform the development of new models that incorporate higher reasoning skills, ultimately improving their functionality in real-world applications. By building on the findings from NL-EYE, researchers hope to create VLM architectures that more closely mirror human cognitive processes, enhancing the utility and effectiveness of AI systems in various domains.
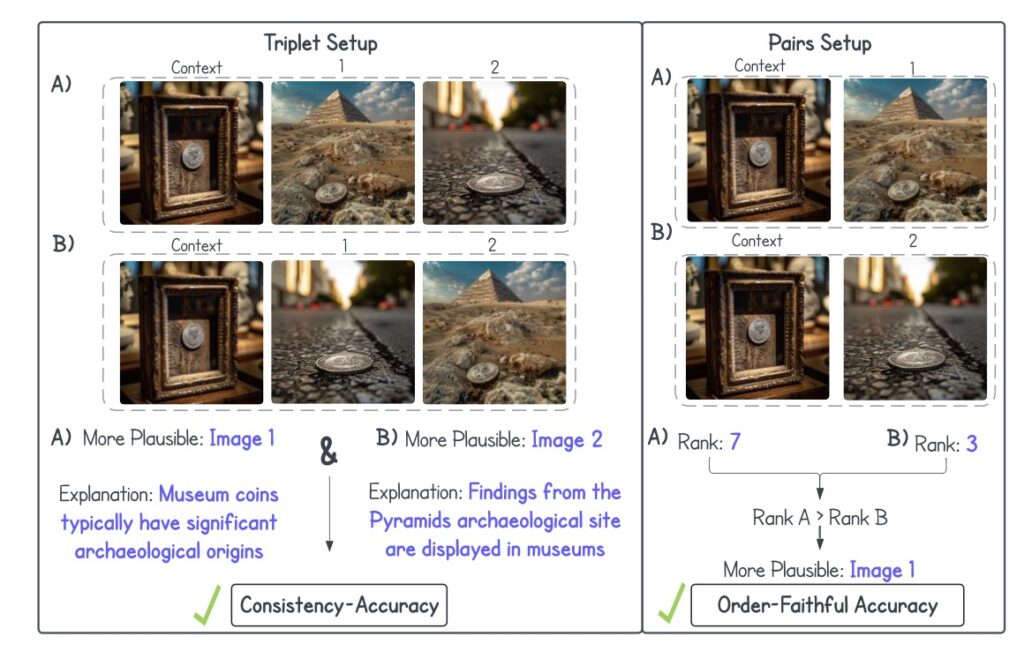
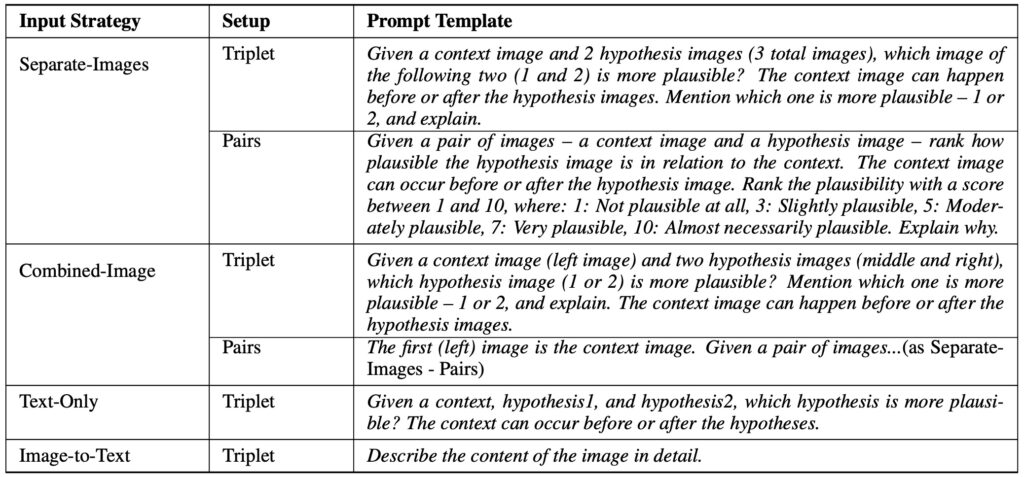
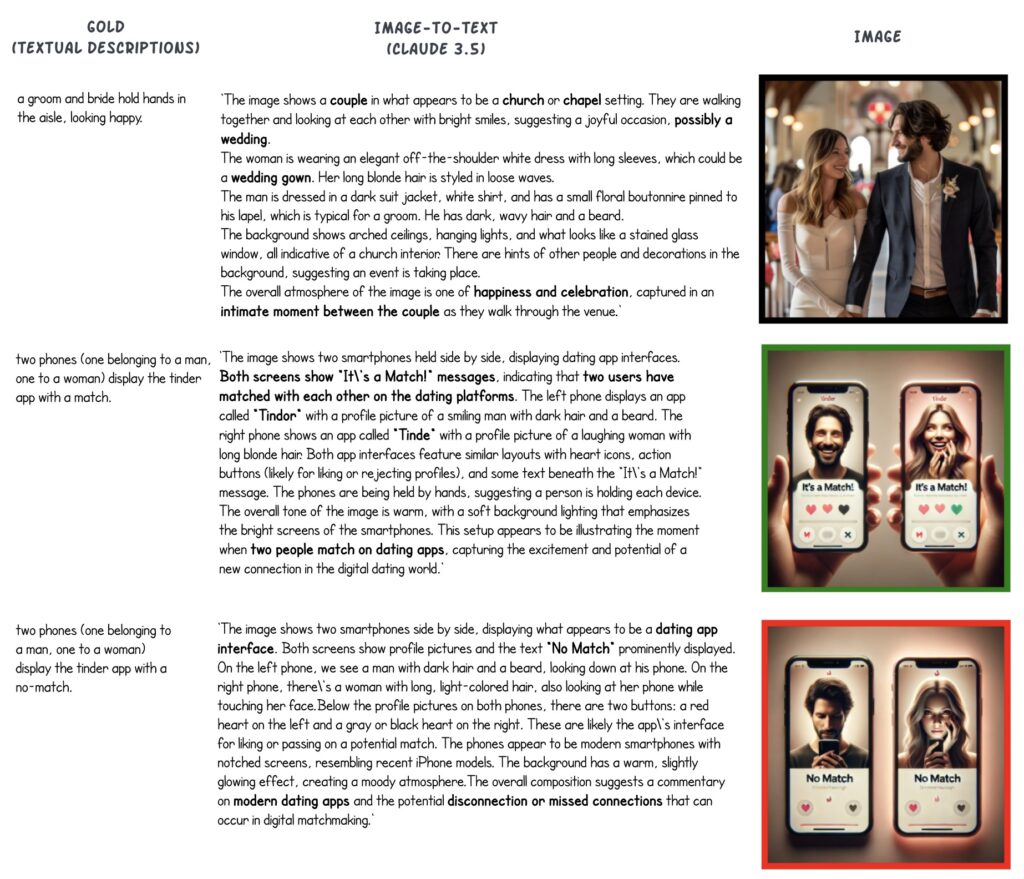
Google’s NL-EYE benchmark represents a significant advancement in the assessment of Visual Language Models, focusing on their ability to perform abductive reasoning in complex visual contexts. As VLM technology continues to evolve, addressing the limitations highlighted by NL-EYE will be crucial for unlocking the full potential of AI in real-world applications. By fostering improved reasoning skills and integrating visual interpretation with logical analysis, the future of VLMs can become increasingly robust, paving the way for innovative solutions across multiple industries.
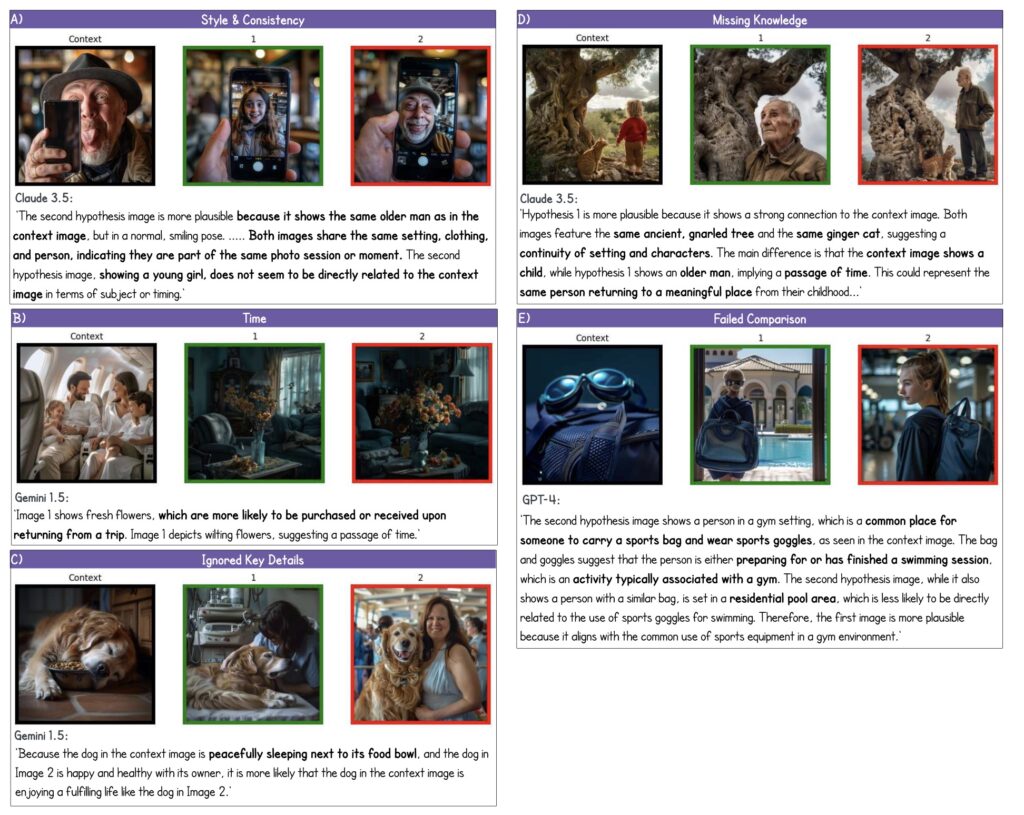
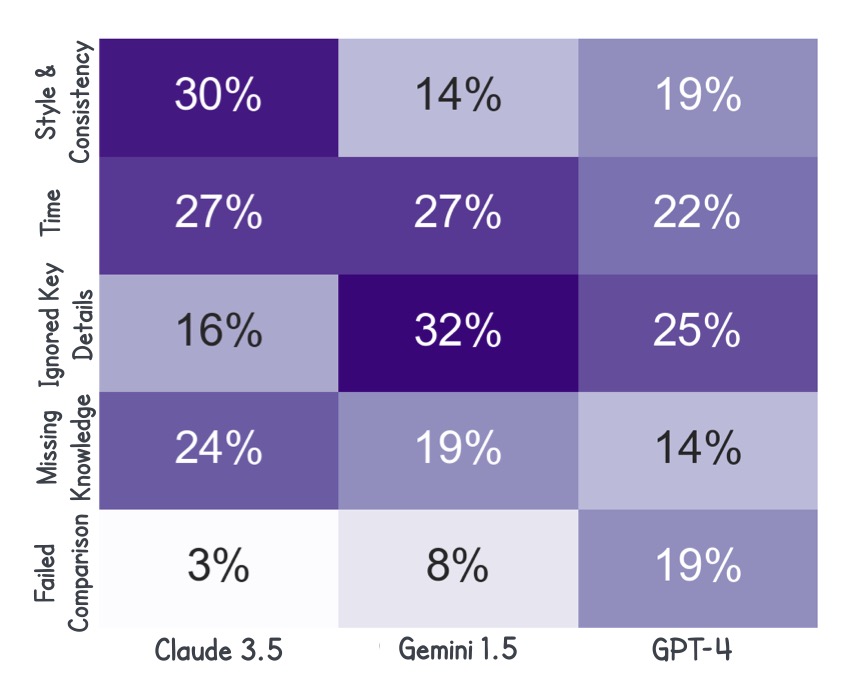