Enhancing Temporal Consistency and Image Quality Without Additional Training
- No Additional Training Required: VideoGuide enhances the performance of pretrained T2V models without necessitating further training or fine-tuning, streamlining the deployment process for developers.
- Improved Temporal Consistency: By utilizing a guiding video diffusion model (VDM) during the denoising process, VideoGuide significantly enhances the temporal coherence of generated video samples, addressing a critical issue in current T2V models.
- Versatile Framework: The framework allows for the use of any existing VDM as a guide, providing flexibility and the potential to improve underperforming models while maintaining their unique characteristics.
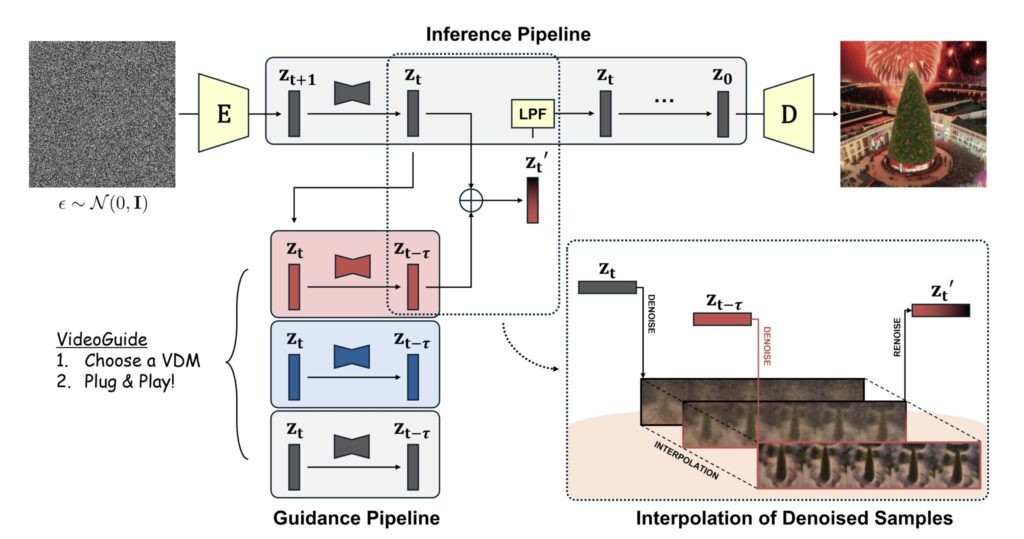
As text-to-image diffusion models have transformed visual content creation, the focus is now shifting towards their application in video generation. However, the complexities of maintaining temporal consistency while generating coherent video sequences from textual descriptions remain a significant hurdle. To address this challenge, researchers have introduced VideoGuide—a robust framework that leverages the strengths of pretrained video diffusion models to enhance the temporal quality of T2V outputs.
VideoGuide operates by employing a guiding VDM during the initial stages of the denoising process. This guiding model can be the same as the one used for inference or any other superior model, offering flexibility in choice. The innovative aspect of VideoGuide lies in its ability to interpolate the guiding model’s denoised samples into the sampling model’s denoising process. This integration helps the sampling model follow a more coherent trajectory, ultimately producing video samples with improved temporal consistency. Such enhancements are particularly valuable for applications where smooth motion and logical progression are critical, such as in film production or animation.
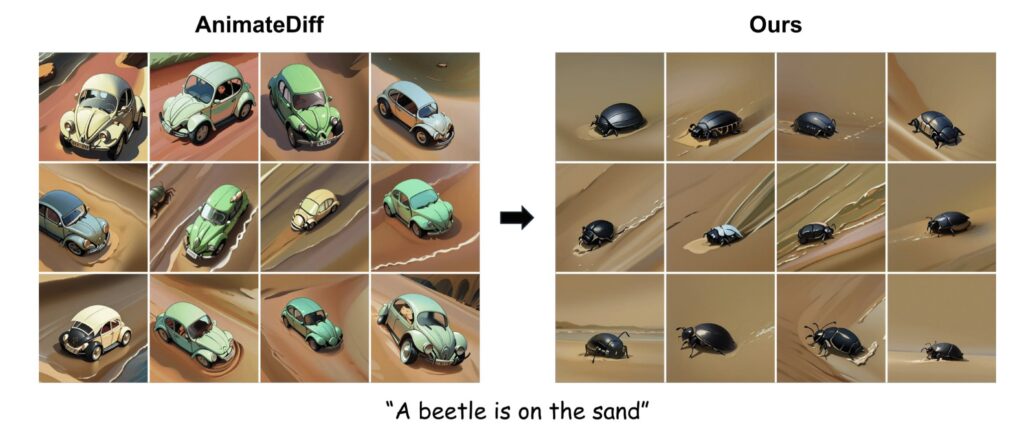
Extensive experiments have demonstrated that VideoGuide significantly outperforms existing methods focused on enhancing temporal quality. Traditional approaches often compromise on image fidelity or require impractically long computational times, making them less viable for real-world applications. In contrast, VideoGuide strikes a balance, providing a cost-effective solution that maintains both the quality of the generated images and the smoothness of motion. This dual focus not only elevates the user experience but also broadens the applicability of T2V models across various domains.
Another noteworthy feature of VideoGuide is its versatility. It allows users to select from a range of pretrained VDMs to serve as a guiding model, empowering developers to elevate their underperforming models to state-of-the-art quality. This adaptability is particularly beneficial when unique characteristics of the base model are desired, as VideoGuide enhances the model’s capabilities without sacrificing its inherent traits. This flexibility positions VideoGuide as an essential tool for developers looking to maximize the potential of their T2V models.
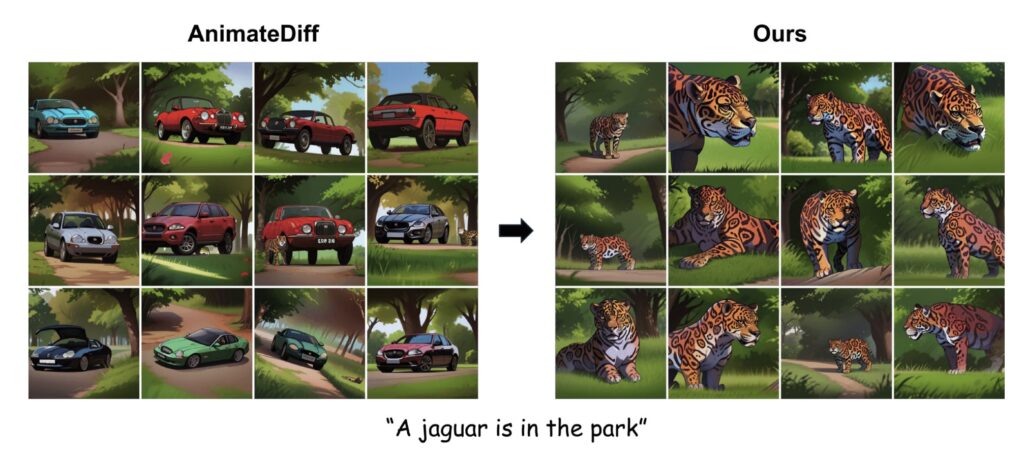
Looking ahead, the potential of VideoGuide extends beyond its current capabilities. As video diffusion models continue to evolve and improve, the framework will remain relevant, allowing users to integrate new and emerging VDMs as guides. This ongoing adaptability ensures that existing models can compete effectively in a rapidly changing landscape, further enriching the possibilities for T2V generation. By continuing to refine this approach, researchers aim to overcome the remaining challenges in video generation, ultimately paving the way for a new era of AI-driven multimedia creation.
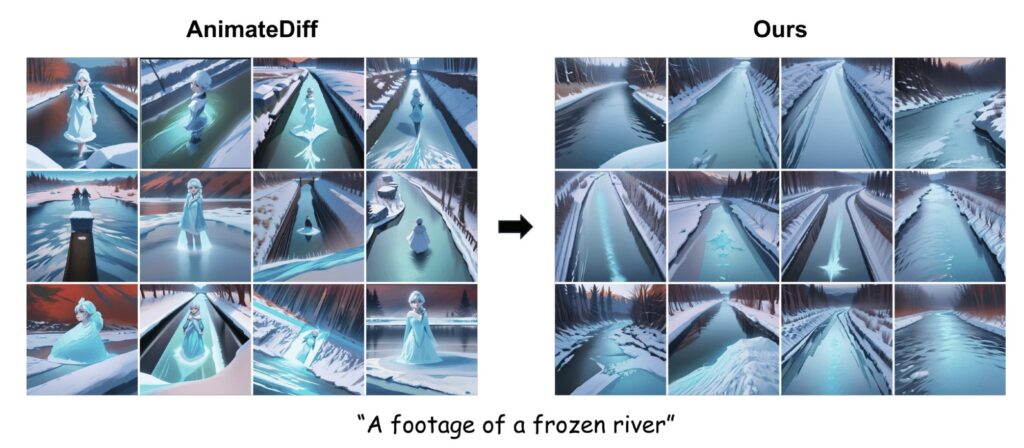
VideoGuide represents a significant advancement in the realm of text-to-video diffusion models, addressing the critical issue of temporal consistency without the need for additional training. By harnessing the strengths of pretrained video diffusion models and offering a flexible, user-friendly framework, VideoGuide sets a new standard for quality and efficiency in the generation of coherent video sequences. As the demand for high-quality, engaging video content continues to rise, innovations like VideoGuide will play a crucial role in shaping the future of AI-generated media.