Enhancing AI’s World Alignment with Rule Learning
In a groundbreaking study, researchers have introduced a novel approach that allows large language models (LLMs) to function as effective world models for model-based agents.
- World Alignment through Rule Learning: The study demonstrates that LLMs can be aligned with their deployed environments using minimal additional rules, effectively bridging gaps in prior knowledge and environmental dynamics.
- Neurosymbolic Approach: By employing a neurosymbolic method, the researchers enable LLMs to generate predictions without extensive gradient updates, making the system more efficient and faster in adapting to real-world scenarios.
- Impressive Performance: The embodied LLM agent, named WALL-E, showcases superior performance in tasks within open-world environments, achieving higher success rates with fewer resources compared to existing methods.
The advent of large language models has transformed numerous domains, from natural language processing to complex reasoning tasks. However, the deployment of these models in dynamic environments, such as games or autonomous systems, has revealed significant challenges. The primary issue lies in the gap between the LLMs’ pre-trained knowledge and the specific dynamics of the environments they operate in. This gap can lead to inaccuracies in predictions, misinterpretations, and even unsafe decision-making.
To address these concerns, researchers have developed WALL-E, a new agent that utilizes a unique 2-Dimensional Autoregressive Transformer architecture for enhanced world model alignment. By leveraging a process known as rule learning, WALL-E can bridge the knowledge gap effectively. The dual-lifting method enables the generation of high-fidelity 3D Gaussian representations, capturing identity and facial details with unprecedented precision. This aligns LLM predictions more closely with the realities of their operational environments.
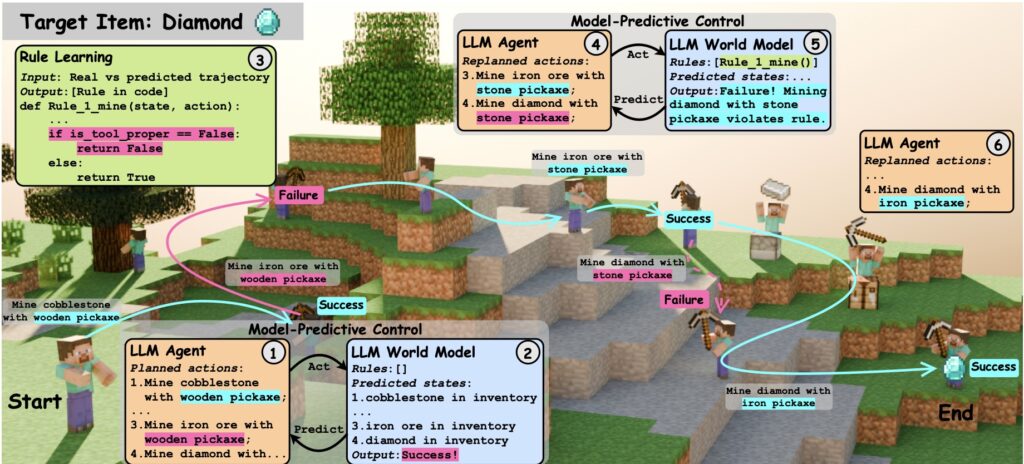
The core innovation of the WALL-E framework is its ability to integrate minimal rules that enhance the LLM’s predictions without extensive retraining. Instead of relying on complex trajectories, WALL-E uses a streamlined approach, processing data through a model-predictive control (MPC) framework. This not only improves the efficiency of learning but also enhances the agent’s ability to navigate and explore environments more effectively.
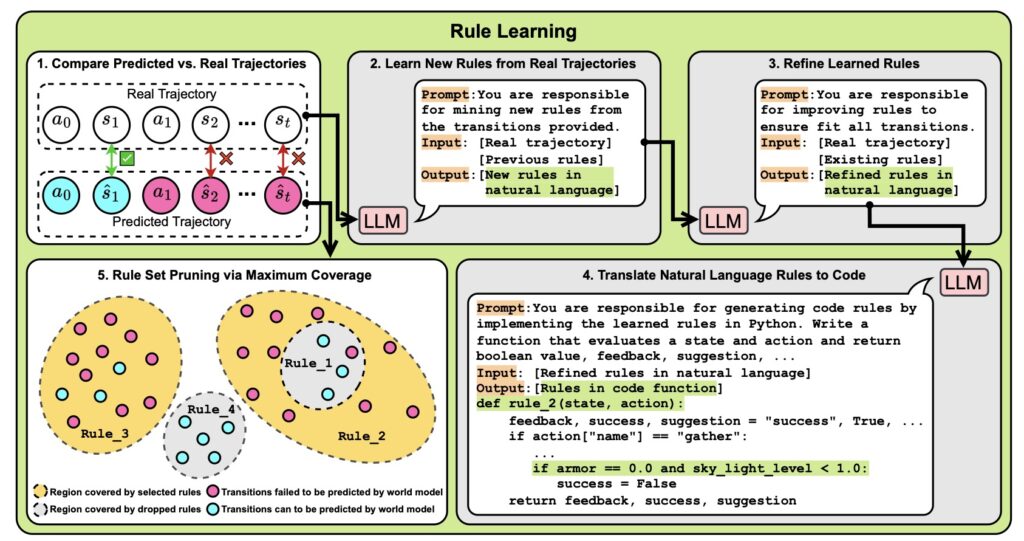
Experiments conducted in various open-world challenges, such as Minecraft and ALFWorld, have demonstrated WALL-E’s superior capabilities. In Minecraft, the agent outperformed existing baselines, achieving a 15–30% higher success rate while using 8–20 fewer replanning rounds. This remarkable efficiency showcases the potential of WALL-E in real-world applications, where resource optimization is critical.
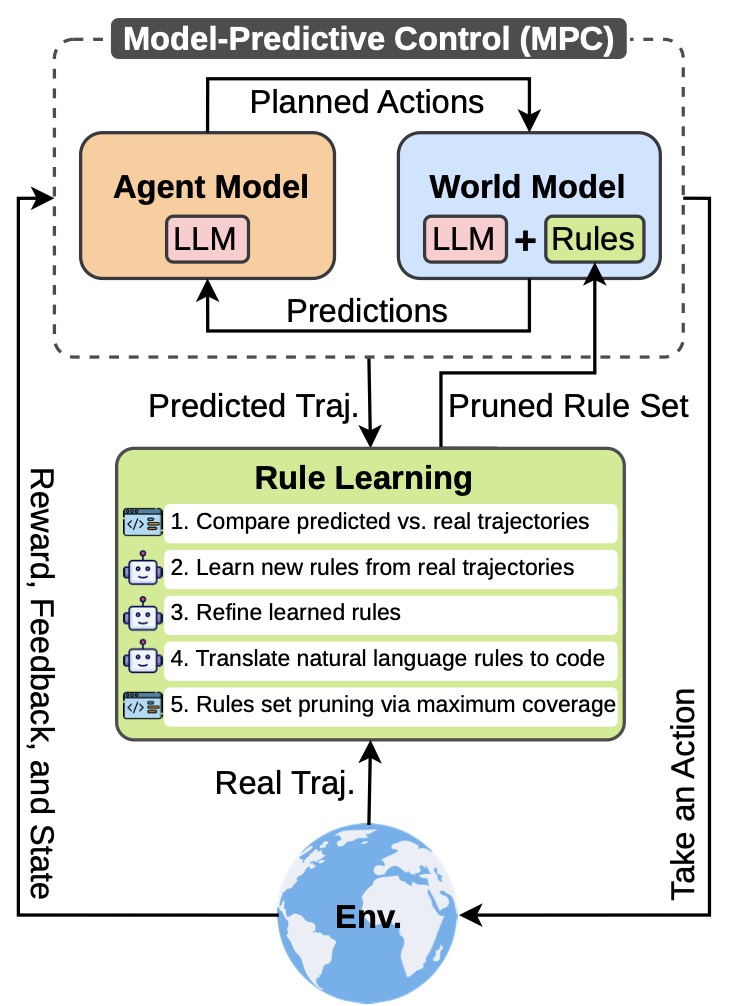
Moreover, the advancements brought by WALL-E open up new avenues for future research in AI. The ability to quickly adapt to new environments while maintaining high performance could be crucial in fields such as robotics, autonomous vehicles, and gaming. The findings indicate that LLMs can effectively serve as world models when properly aligned, paving the way for more sophisticated and reliable AI systems.
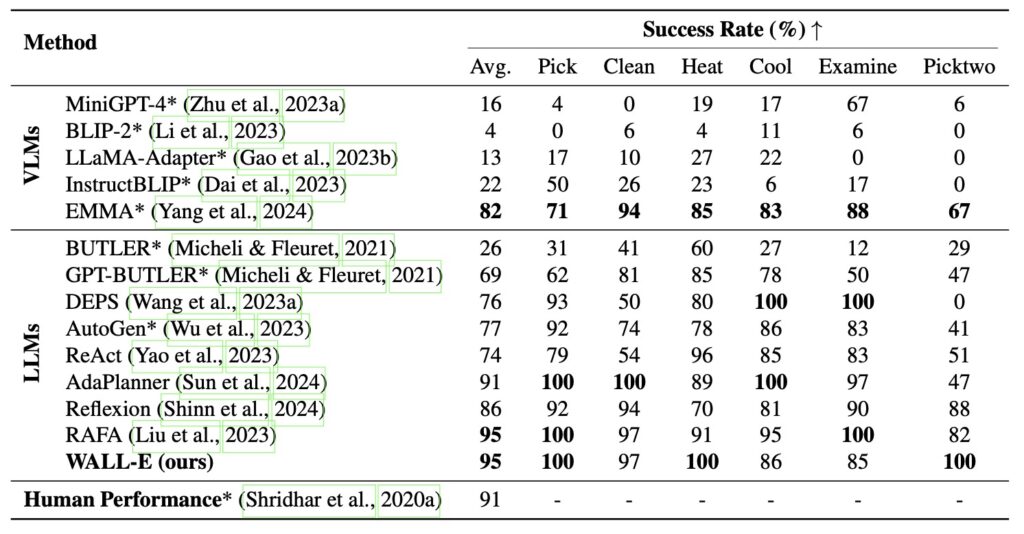
The introduction of WALL-E represents a significant leap forward in the application of large language models in dynamic environments. By focusing on world alignment through rule learning, researchers have demonstrated that it is possible to enhance the capabilities of LLMs while minimizing the need for extensive retraining. As AI continues to evolve, frameworks like WALL-E could become essential tools for developing intelligent agents capable of navigating complex and ever-changing worlds.