The Future of Tailored Video Content Without Complex Fine-Tuning
In a groundbreaking advancement for video generation technology, DreamVideo-2 introduces a zero-shot customization framework that allows users to create videos tailored to specific subjects and motion trajectories without the need for complicated fine-tuning. This innovative model utilizes reference attention and mask-guided motion control to achieve precise motion dynamics while ensuring accurate subject representation.
- Seamless Integration of Motion Control and Subject Customization:Â DreamVideo-2 employs advanced techniques to balance motion control and subject learning, addressing significant challenges faced by previous models in video generation.
- Efficiency in Video Generation:Â The new model can generate high-quality videos at impressive speeds, making it suitable for various real-world applications while simplifying the user experience.
- Open Access for Further Development:Â The creators of DreamVideo-2 plan to share their dataset, code, and models publicly, encouraging further research and development in the field of customized video generation.
The rise of AI-driven video generation tools has opened doors to a new realm of creative possibilities, allowing users to generate content that meets their specific needs. However, existing solutions often require complex test-time fine-tuning and struggle to balance subject representation with motion dynamics. This imbalance has hindered the effectiveness and applicability of these models in practical scenarios. Recognizing these challenges, DreamVideo-2 seeks to streamline the customization process, making it more accessible and efficient for users across various industries.
The Innovative Mechanics Behind DreamVideo-2
At the core of DreamVideo-2 lies its unique architecture that facilitates the generation of videos with precise motion control and subject customization. The introduction of reference attention allows the model to leverage its inherent capabilities for subject learning effectively. Meanwhile, the mask-guided motion module capitalizes on robust motion signals derived from bounding boxes to achieve a high level of motion accuracy. This dual approach ensures that the model can generate videos that not only accurately depict the specified subject but also follow defined motion trajectories seamlessly.
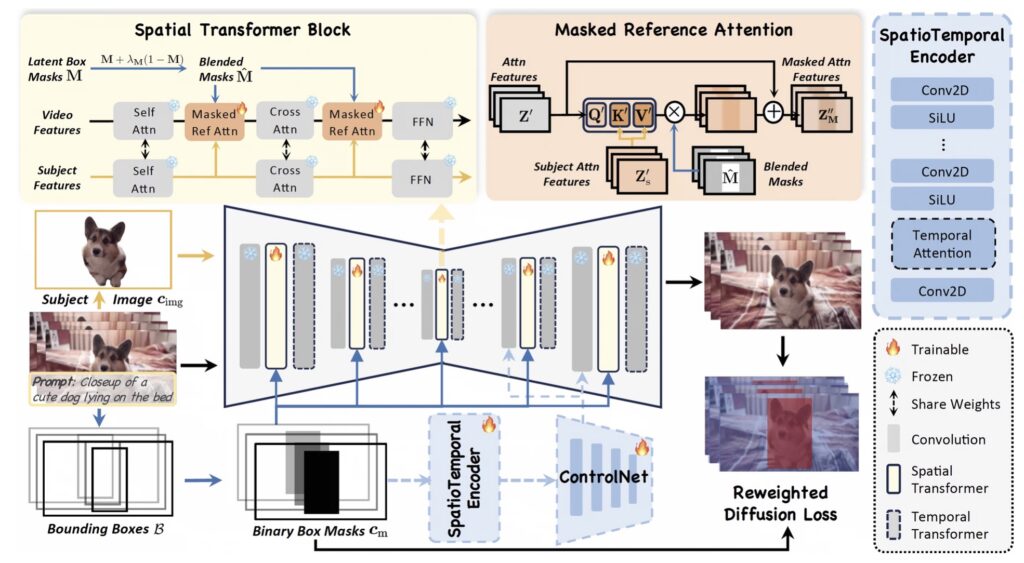
Striking the Balance: Enhancing Subject Representation
One of the significant challenges encountered during the development of DreamVideo-2 was the tendency for motion control to overshadow subject learning. To address this issue, the creators implemented two crucial innovations: masked reference attention and a reweighted diffusion loss. The masked reference attention integrates blended latent masks, enhancing subject representation in targeted areas. Simultaneously, the reweighted diffusion loss differentiates contributions from within and outside the bounding boxes, ensuring a balanced approach that maintains the integrity of both subject and motion dynamics. This careful calibration results in videos that deliver a cohesive visual experience without sacrificing detail or accuracy.
Real-World Applications and Future Prospects
DreamVideo-2’s capabilities are not just theoretical; extensive experiments on a newly curated dataset have demonstrated its superiority over state-of-the-art methods in both subject customization and motion control. This breakthrough positions DreamVideo-2 as a valuable tool for various applications, including content creation for marketing, gaming, and virtual environments. As users increasingly seek tailored video content, the demand for models like DreamVideo-2 that can deliver high-quality results efficiently will continue to grow.
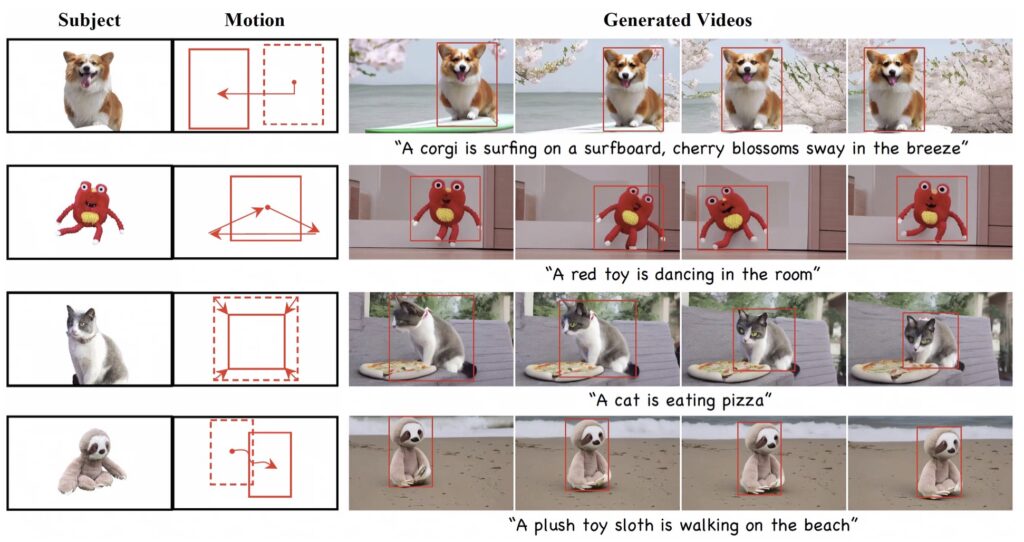
A Call for Open Collaboration in Video Generation
The commitment to sharing the dataset, code, and models for DreamVideo-2 underscores the importance of collaboration in advancing AI technologies. By providing open access to these resources, the creators invite further exploration and innovation within the field of customized video generation. As researchers and developers build upon DreamVideo-2’s foundations, we can expect to see even more sophisticated and versatile applications emerge, pushing the boundaries of what’s possible in video content creation.
DreamVideo-2 marks a significant step forward in the realm of AI-driven video generation, offering a powerful framework that balances motion control and subject representation. As the technology continues to evolve, its potential to transform how we create and consume video content is undeniable, paving the way for a future where customized videos become the norm.