With advanced techniques like Chain-of-Thought and Monte Carlo Tree Search, Marco-o1 sets a new standard for tackling complex, ambiguous challenges.
- Beyond the Metrics: Marco-o1 addresses the challenge of solving open-ended problems where clear evaluation criteria are lacking, expanding AI’s applicability to real-world scenarios.
- Cutting-Edge Techniques: The model employs advanced methods like Chain-of-Thought fine-tuning and Monte Carlo Tree Search to enhance reasoning and problem-solving abilities.
- Promising Results: Marco-o1 has shown significant improvements over its predecessors in accuracy, translation tasks, and managing ambiguous challenges.
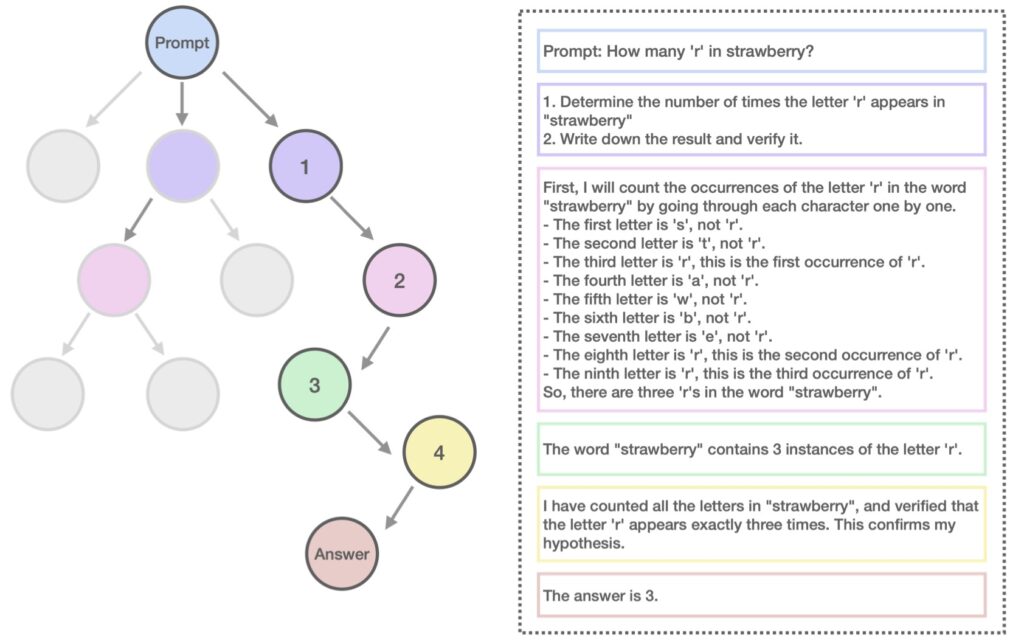
As AI continues to revolutionize industries, one area has remained notably challenging: solving open-ended, real-world problems. These scenarios often lack clear-cut answers or definitive metrics for success. Enter Marco-o1, Alibaba’s latest Large Reasoning Model (LRM), designed to push the boundaries of artificial intelligence by tackling these ambiguous tasks.
Developed by Alibaba’s MarcoPolo team, Marco-o1 builds upon lessons from OpenAI’s o1 model while introducing significant innovations. Unlike many existing models that excel in structured environments, Marco-o1 is engineered to generalize across multiple domains, including tasks that demand creativity, cultural understanding, and nuanced problem-solving.
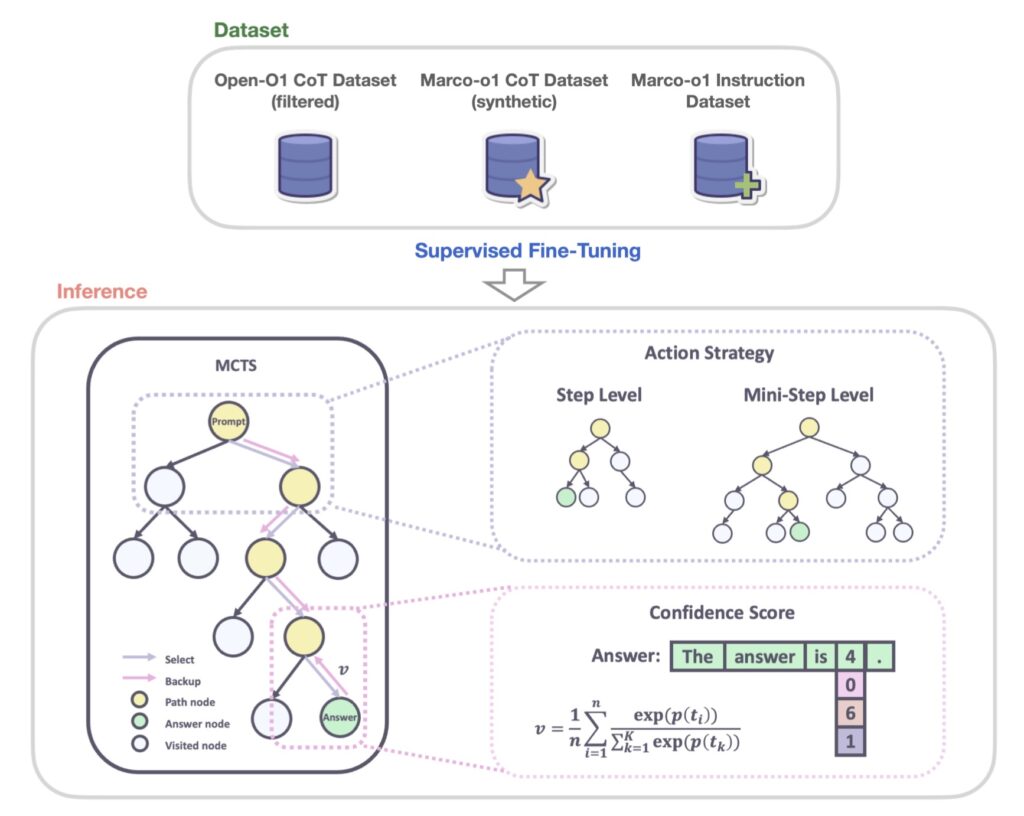
A New Toolkit for Complex Reasoning
Marco-o1 employs a series of advanced techniques to enhance its capabilities:
Chain-of-Thought (CoT) Fine-Tuning: By explicitly tracing its reasoning steps, Marco-o1 provides a transparent and systematic approach to solving complex problems. This technique not only improves accuracy but also makes the solution process easier to evaluate and refine.
Monte Carlo Tree Search (MCTS): This method enables the model to explore multiple reasoning paths, assigning confidence scores to alternative solutions. By prioritizing the most promising paths, Marco-o1 improves its efficiency in navigating complex challenges.
Dynamic Reasoning Action Strategy: This feature adjusts the level of granularity in problem-solving, ensuring an optimal balance between precision and speed.
Together, these innovations empower Marco-o1 to excel in both structured tasks and nuanced, real-world applications, such as translating cultural expressions or handling open-ended queries.
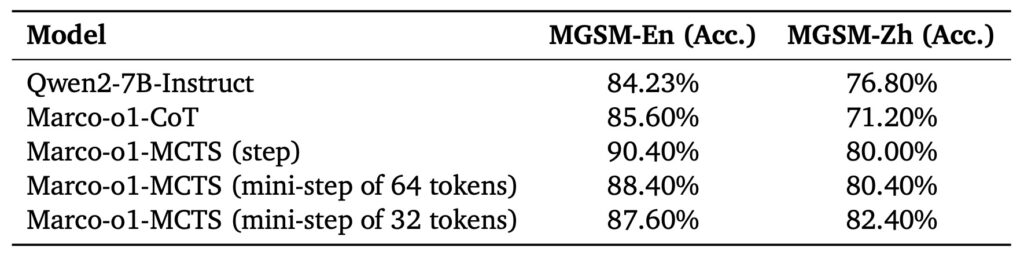
Results That Speak for Themselves
Marco-o1’s capabilities have been validated through rigorous testing. On the MGSM dataset, the model achieved a 6.17% accuracy improvement for English tasks and a 5.60% improvement for Chinese tasks, surpassing its predecessors. Its ability to translate colloquial phrases with cultural nuance further underscores its versatility.
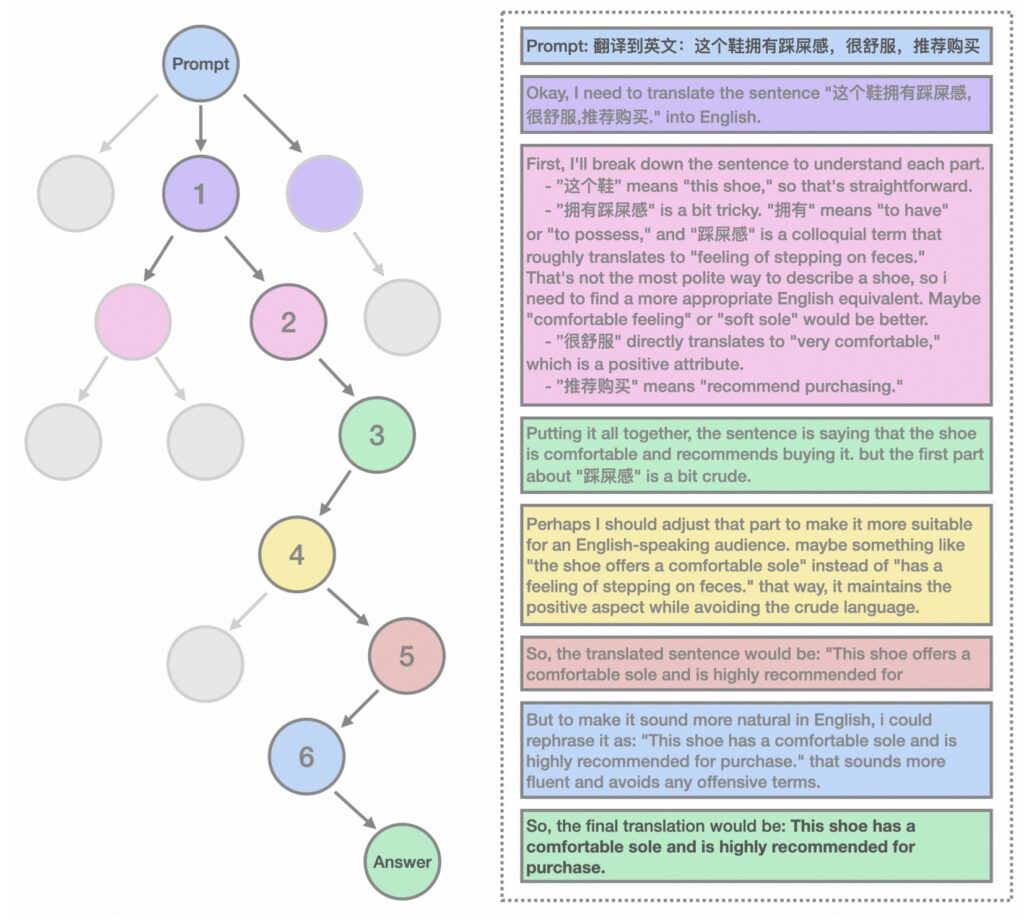
These advancements position Marco-o1 as a significant leap forward in AI, particularly for applications where clear metrics are unavailable or where solutions require more than straightforward computation.
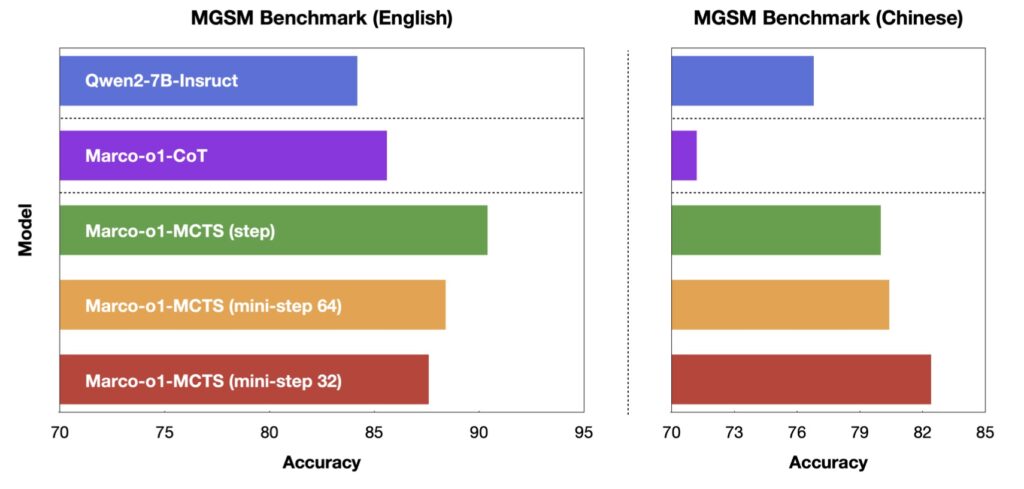
The Road Ahead for Marco-o1
Alibaba plans to refine Marco-o1 further by integrating Outcome and Process Reward Modeling. This approach aims to reduce randomness in decision-making, allowing the model to solve an even broader range of problems with higher reliability.
Marco-o1 represents more than just a technological advancement—it signals a shift in how AI can approach the complexities of real-world challenges. By blending structured reasoning with the flexibility to handle ambiguity, Marco-o1 is poised to play a transformative role in industries ranging from language translation to creative problem-solving.
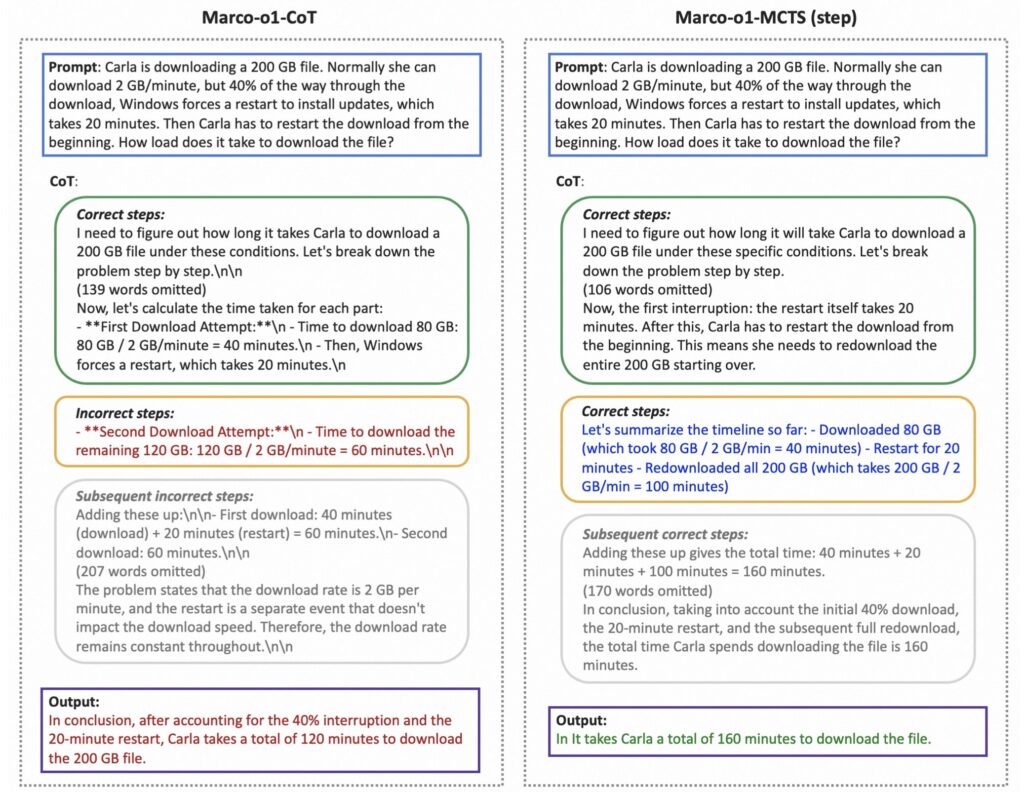