From Hallucination-Free Play to Grandmaster Elo Ratings, MAV Redefines AI Strategy and Planning
- Integrated Decision-Making: The Multi-Action-Value (MAV) model combines state tracking, planning, and action evaluation into a single, efficient system.
- Grandmaster-Level Performance: MAV achieves a chess Elo rating of 2923, rivaling human grandmasters while using far fewer computational resources than traditional AI systems.
- Generalized Applications: Beyond chess, MAV excels in diverse games like Chess960, Connect Four, and Hex, highlighting its adaptability and strategic depth.
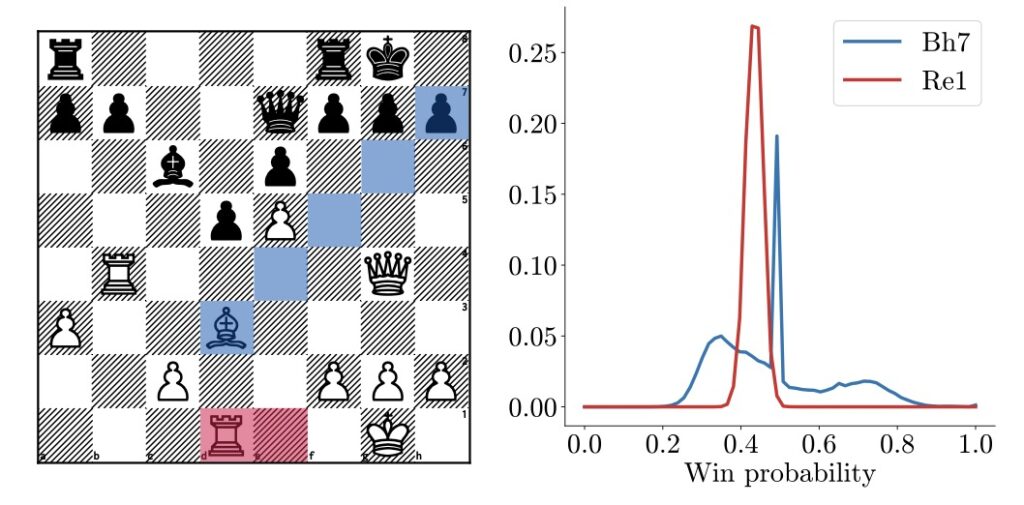
Board games like chess and Connect Four have long been fertile ground for AI development, challenging systems to master decision-making and strategy. Yet, even the most advanced AI models often falter in multi-step reasoning and long-term planning. Enter DeepMind’s Multi-Action-Value (MAV) model, an AI breakthrough that achieves Grandmaster-level performance while addressing key limitations of traditional systems.
Unlike previous approaches, MAV operates without external engines, relying instead on an innovative Transformer-based architecture. Trained on billions of game states and action values, it autonomously tracks game dynamics, predicts legal moves, and evaluates strategies—all with unprecedented precision.
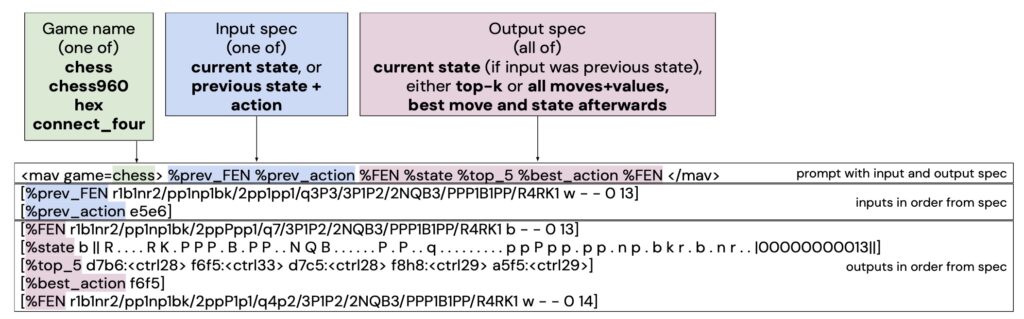
Key Innovations in MAV
Comprehensive Integration
The MAV model consolidates world modeling, policy evaluation, and action prediction into a unified framework. This eliminates the reliance on external tools like Monte Carlo Tree Search (MCTS), significantly improving efficiency and scalability.
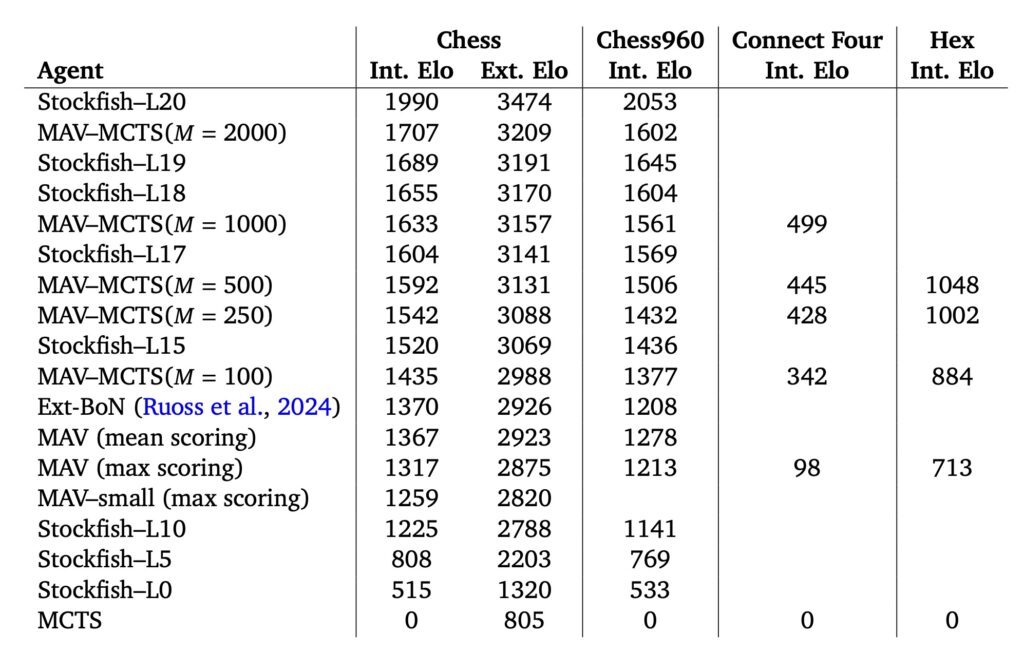
Internal and External Search Mechanisms
MAV employs internal search to simulate potential moves and backtrack to refine strategies. External search mechanisms further enhance decision-making, boosting performance by over 300 Elo points in chess and 244 Elo points in Connect Four.
Precision and Reliability
MAV’s state prediction boasts 99.9% precision and recall for legal moves in chess, minimizing errors and eliminating hallucinations common in less advanced systems.
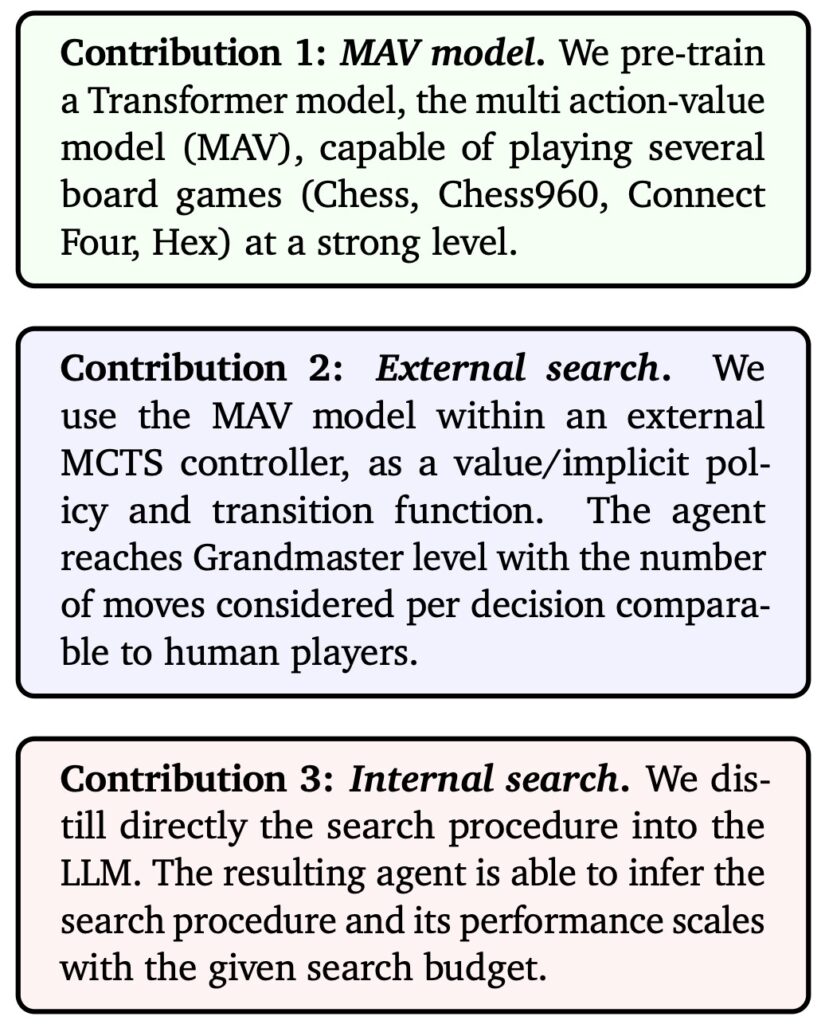
Performance Across Games
MAV’s versatility is evident in its success across multiple board games:
- Chess: Achieving an Elo rating of 2923, MAV surpasses Stockfish L10 and performs on par with human Grandmasters while requiring only 1,000 simulations—far fewer than AlphaZero’s 10,000.
- Chess960: MAV leverages its training on diverse positions to excel in this variant, demonstrating adaptability to dynamic rules.
- Connect Four and Hex: MAV’s superior state-tracking capabilities enable consistent improvements, even in games with limited pre-existing AI benchmarks.
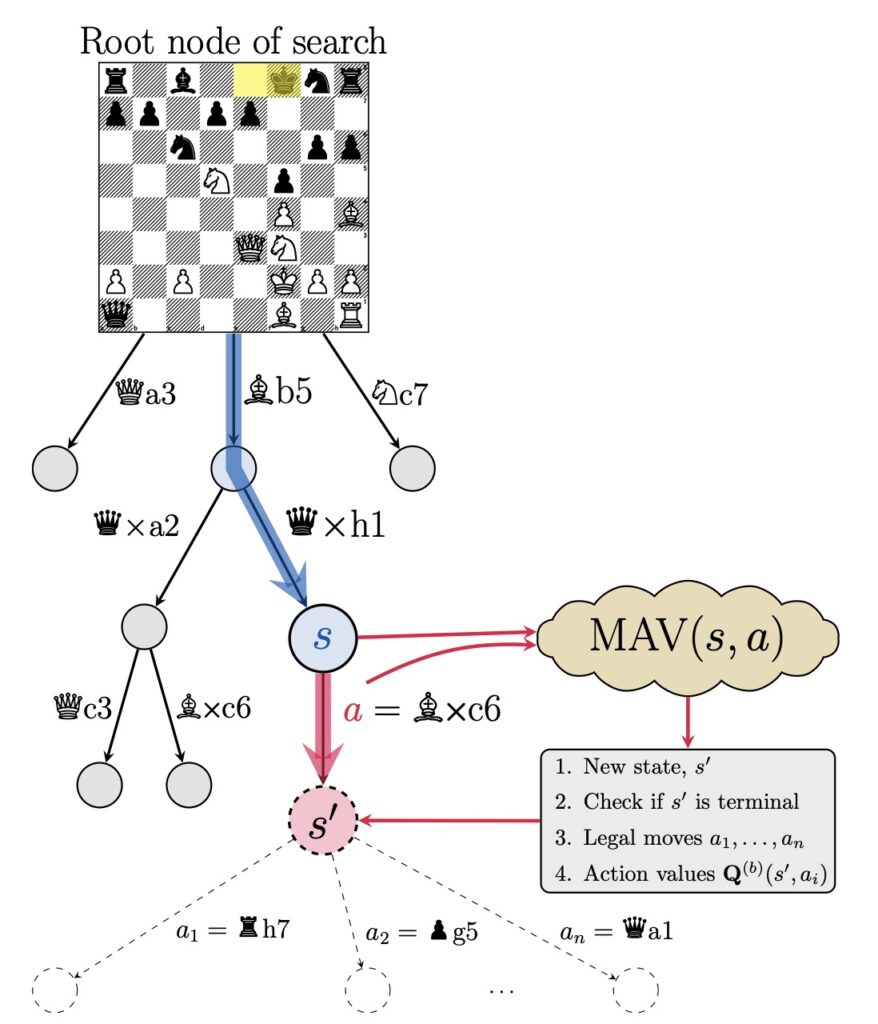
Implications Beyond Games
DeepMind’s innovations in MAV extend far beyond the gaming world. By integrating planning, reasoning, and decision-making into a single system, MAV sets the stage for broader applications in domains requiring strategic foresight, such as logistics, autonomous systems, and complex simulations.
Moreover, MAV’s ability to generalize its search-based methods offers exciting possibilities for enhancing large language models in non-gaming contexts. Tasks like legal analysis, scientific discovery, and multi-step programming could benefit from the techniques pioneered by MAV.
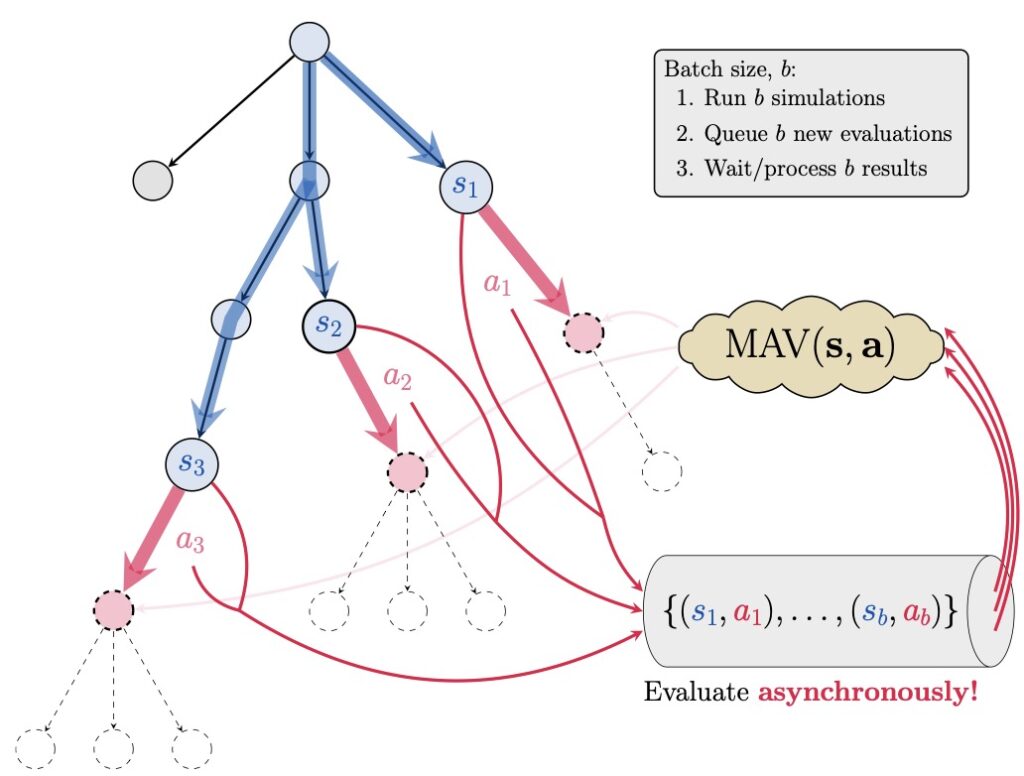
Pioneering the Future of AI Strategy
DeepMind’s MAV model represents a significant leap forward in AI planning and strategy. By achieving Grandmaster-level performance across multiple games while maintaining efficiency, MAV redefines the potential of AI systems. Its integration of internal and external planning mechanisms, coupled with high precision and adaptability, makes it a cornerstone for the future of AI-driven problem-solving.
As researchers continue to explore MAV’s applications beyond board games, its impact on AI strategy and decision-making promises to be nothing short of transformative.
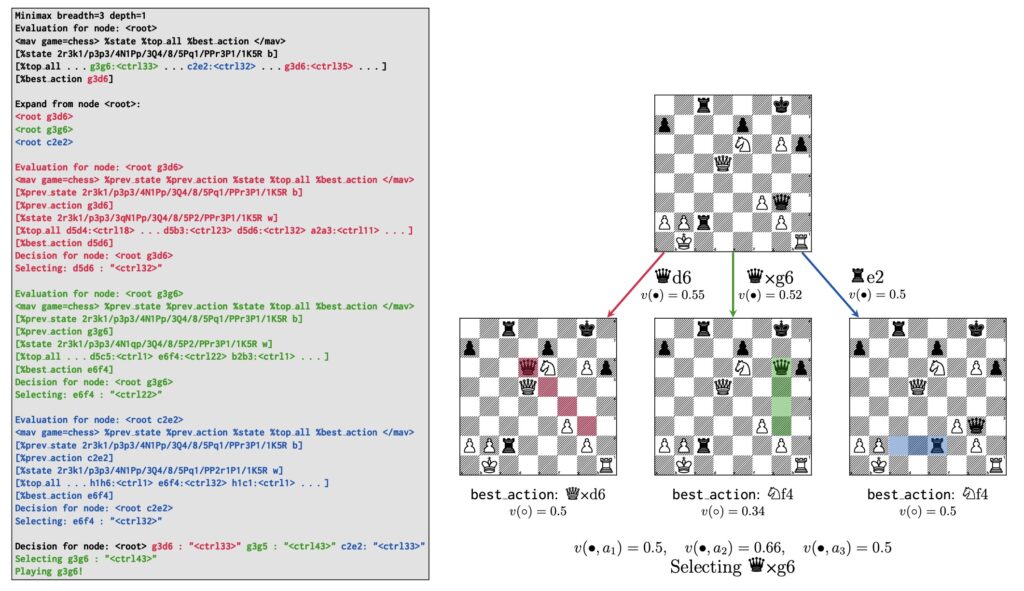