Efficient Training, Self-Enhancing Alignment, and Versatile Applications for the Next Generation of MLLMs
- Unified Multimodal Framework:Â ILLUME integrates understanding and generation capabilities through a next-token prediction approach, achieving state-of-the-art performance across benchmarks.
- Efficient Data Utilization:Â A novel vision tokenizer and progressive training reduce pretraining dataset size to just 15M, enhancing efficiency without sacrificing accuracy.
- Self-Enhancing Multimodal Alignment:Â The model aligns text and image generation with semantic consistency, improving multimodal understanding and avoiding unrealistic predictions.
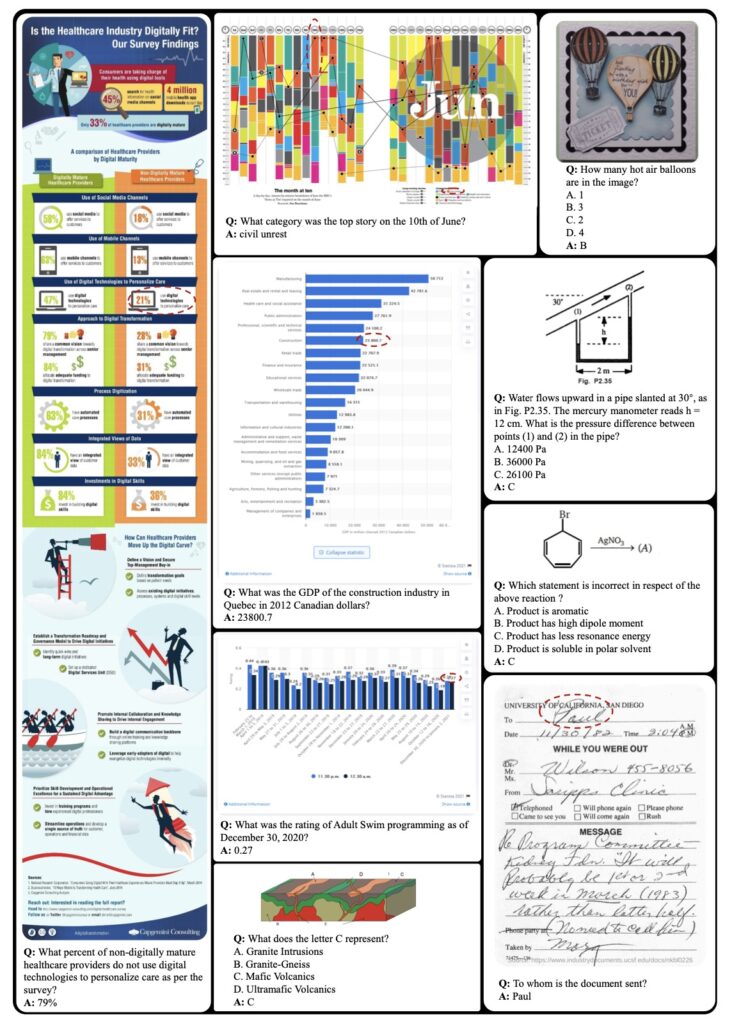
Huawei introduces ILLUME, a cutting-edge Multimodal Large Language Model (MLLM) designed to seamlessly integrate image and text understanding and generation. With its innovative architecture and training strategies, ILLUME achieves state-of-the-art results while significantly reducing the data requirements typically needed for pretraining.
ILLUME is built on a unified next-token prediction framework, allowing it to handle multimodal tasks such as understanding, generating, and editing with remarkable precision. This positions ILLUME as a versatile tool for a wide range of applications, from image captioning to multimodal editing.
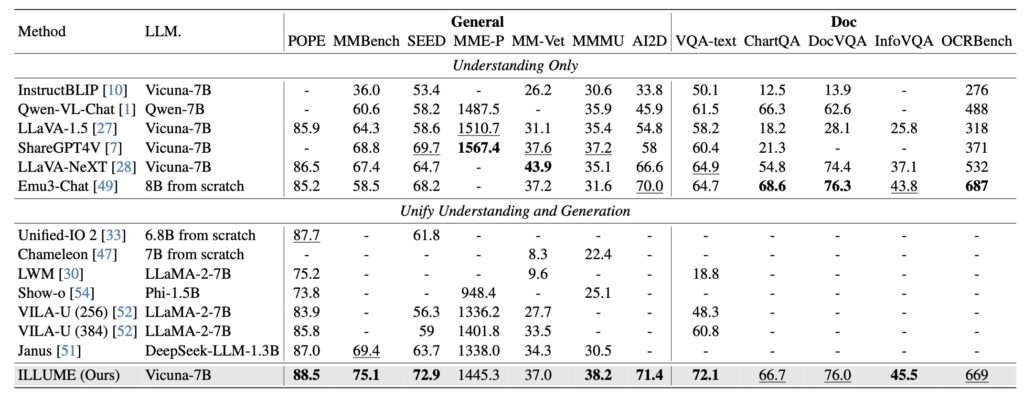
Key Innovations in ILLUME
Data Efficiency with Vision Tokenization
ILLUME leverages a vision tokenizer that incorporates semantic information to align images and text more effectively. This enables pretraining on a dataset of only 15 million samples, four times smaller than comparable models, while maintaining competitive or superior performance.
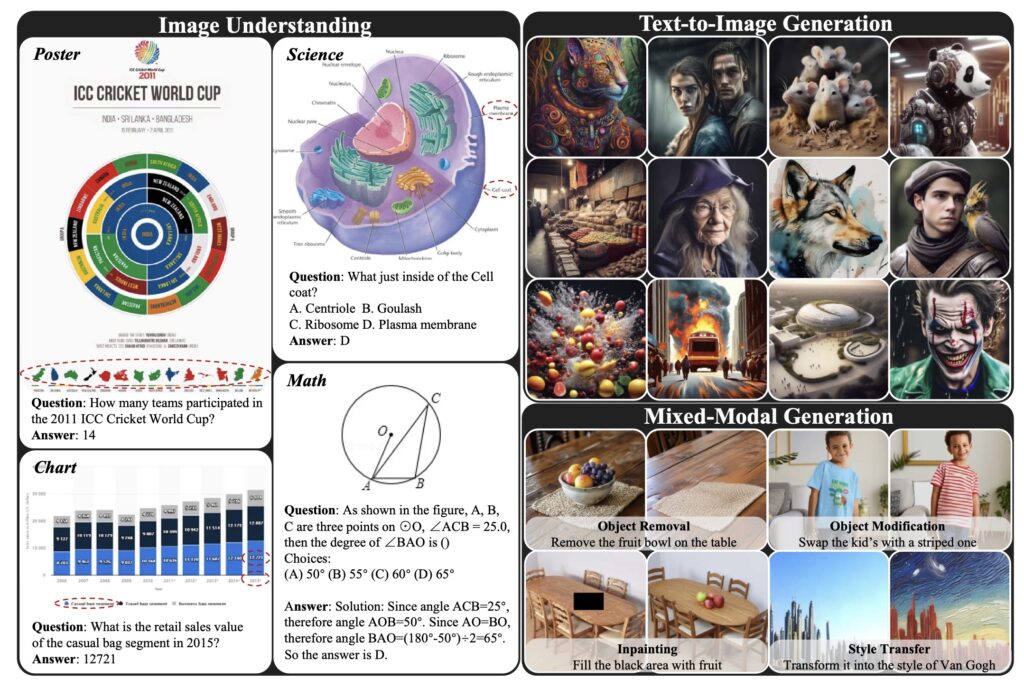
Self-Enhancing Multimodal Alignment
ILLUME introduces a novel self-enhancing alignment scheme. The model evaluates the consistency between text descriptions and its own generated images, enabling it to self-correct and improve its understanding of multimodal inputs. This results in more accurate interpretations and realistic outputs.
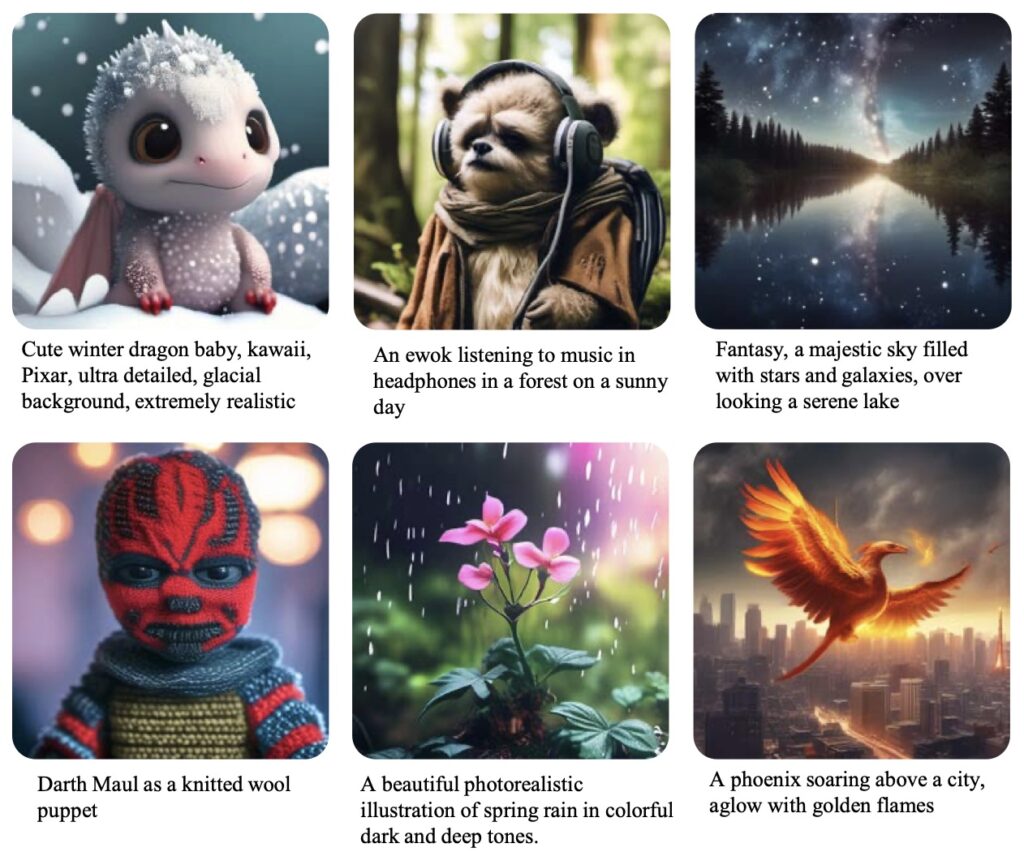
Multimodal Excellence
Extensive experiments demonstrate that ILLUME competes with and even surpasses leading models like Janus across benchmarks for multimodal understanding, generation, and editing. Its balanced architecture ensures top-tier performance in both specialized and unified MLLM tasks.
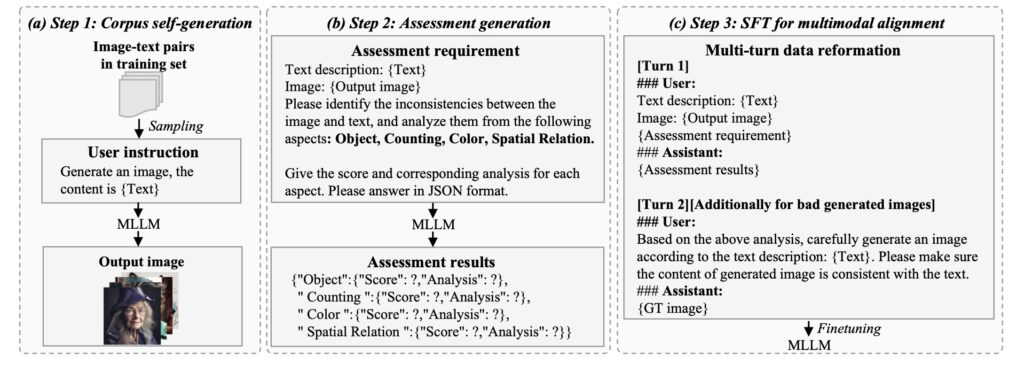
Future Directions for ILLUME
Huawei plans to expand ILLUME’s capabilities in several key areas:
- Broader Modality Support:Â Extend functionality to include video, audio, and 3D data, making it applicable across diverse domains such as entertainment, healthcare, and industrial design.
- Enhanced Vision Tokenizer:Â Develop a versatile tokenizer capable of supporting both images and videos while further integrating semantic insights.
- Advanced Self-Enhancing Techniques: Incorporate criteria like aesthetic quality to align outputs more closely with human preferences, improving both understanding and generation.
These advancements aim to transform ILLUME into a universal, highly efficient, multimodal AI system for any task and any modality.
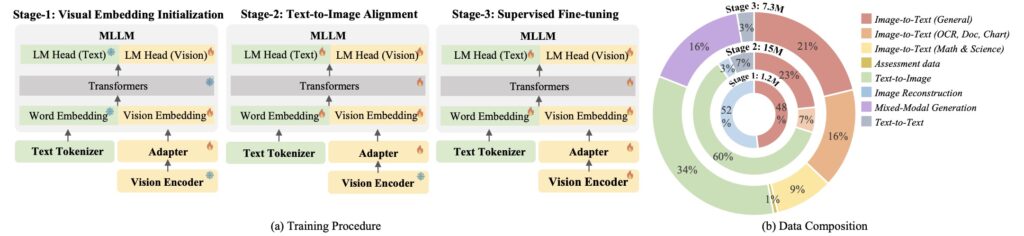
Multimodal AI with ILLUME
ILLUME sets a new standard for multimodal AI, combining cutting-edge efficiency with powerful self-enhancing capabilities. Its ability to perform across a variety of tasks while reducing data requirements marks a significant leap forward for MLLMs.
As Huawei continues to refine and expand ILLUME’s features, the model promises to drive innovation across industries, providing tools for more intuitive and accurate multimodal interactions. With its groundbreaking architecture and forward-looking roadmap, ILLUME illuminates the future of unified multimodal AI.
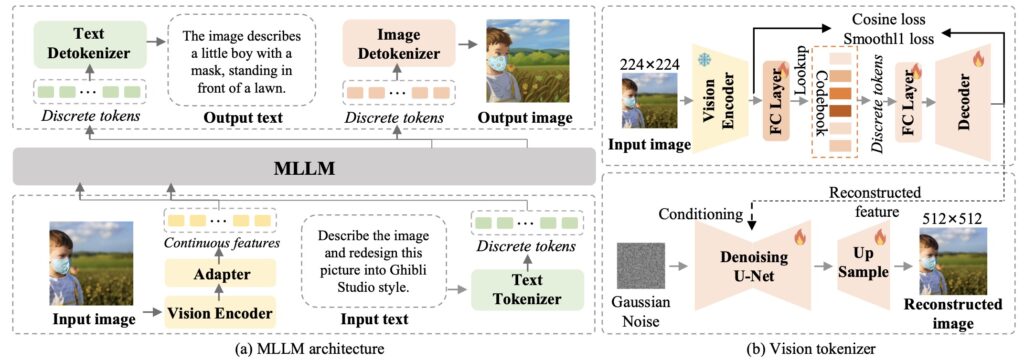
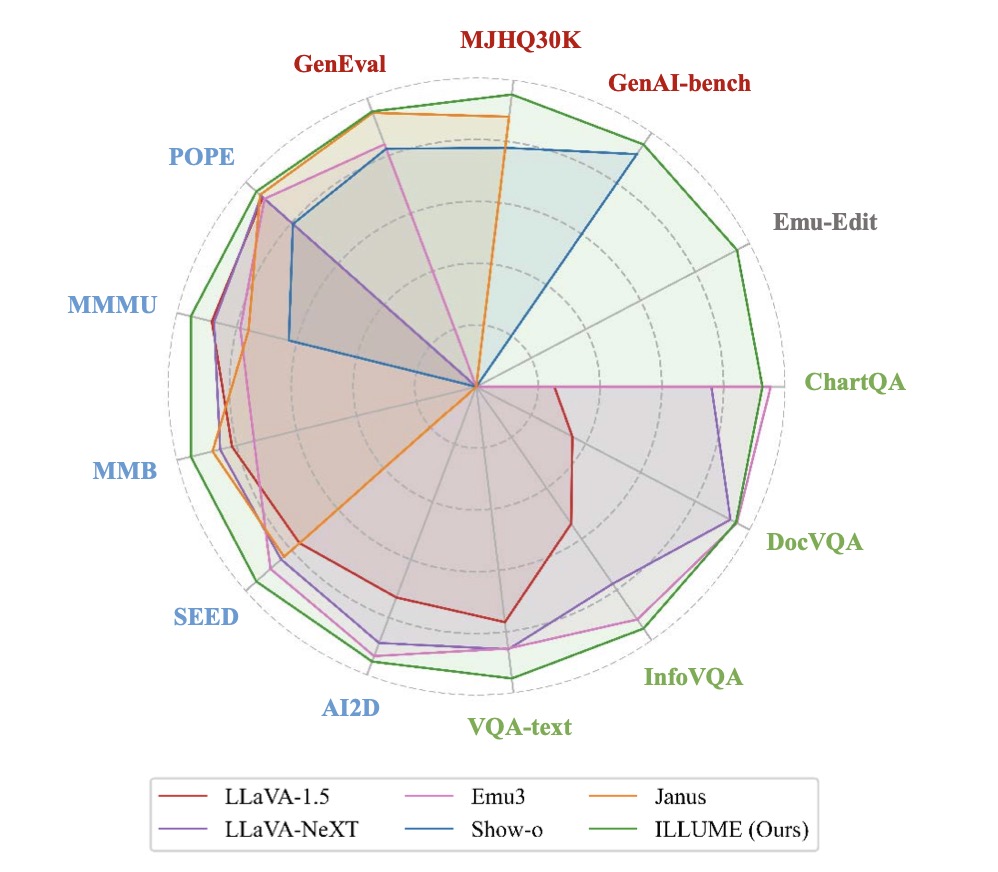