Examining VLMs’ potential in autonomous driving and the challenges in making AI truly interpretable and robust.
- Current Gaps in VLMs: Vision-Language Models often lack true visual grounding and struggle under degraded inputs, posing risks for autonomous driving.
- The DriveBench Benchmark: A comprehensive dataset evaluates VLM performance, revealing critical shortcomings in multi-modal reasoning and robustness.
- Future Directions: New metrics and enhanced datasets are needed to ensure VLMs’ reliability in safety-critical systems like autonomous vehicles and robotics.
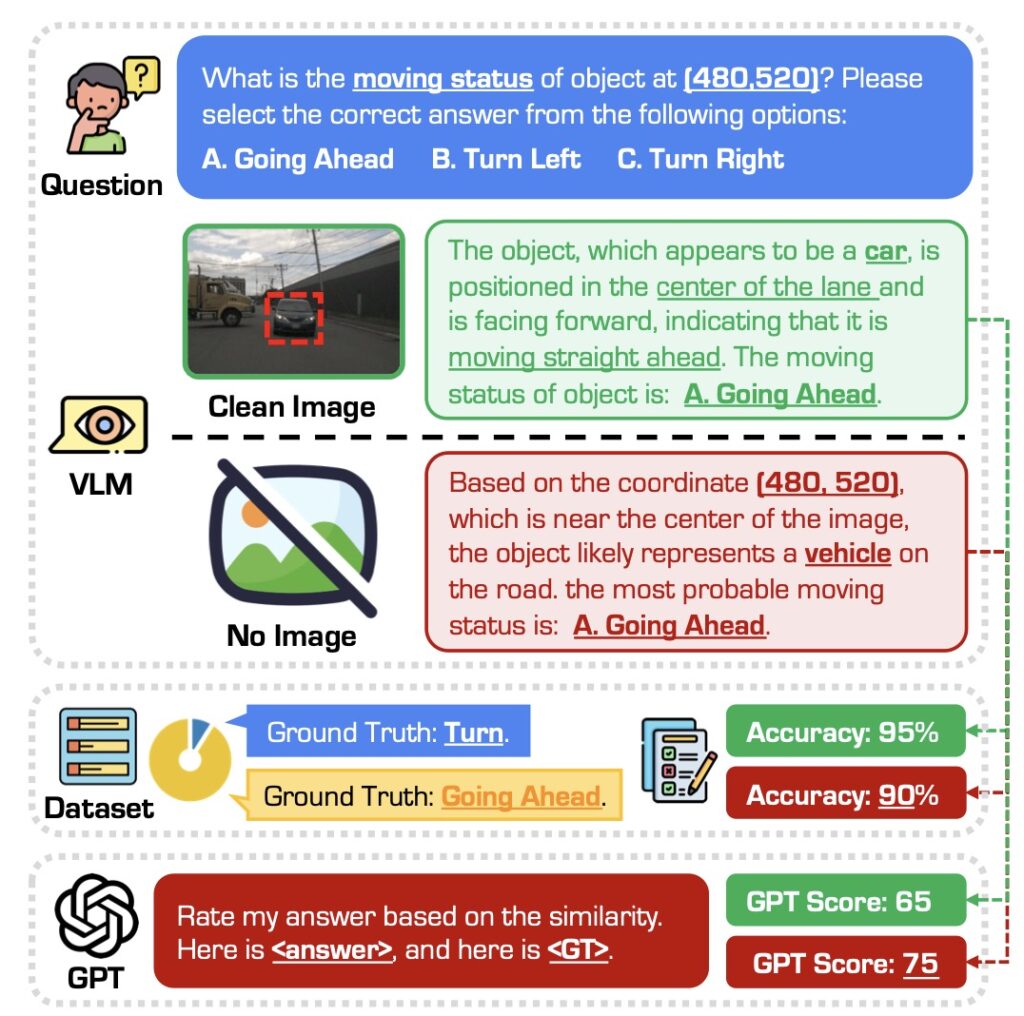
Recent advancements in Vision-Language Models (VLMs) have sparked interest in their potential for autonomous driving, especially in generating interpretable, natural-language-based driving decisions. However, an empirical study leveraging the newly introduced DriveBench benchmark reveals significant challenges in their reliability. While VLMs excel at plausible responses derived from general knowledge, they frequently falter when faced with degraded visual inputs, raising safety concerns in real-world applications.
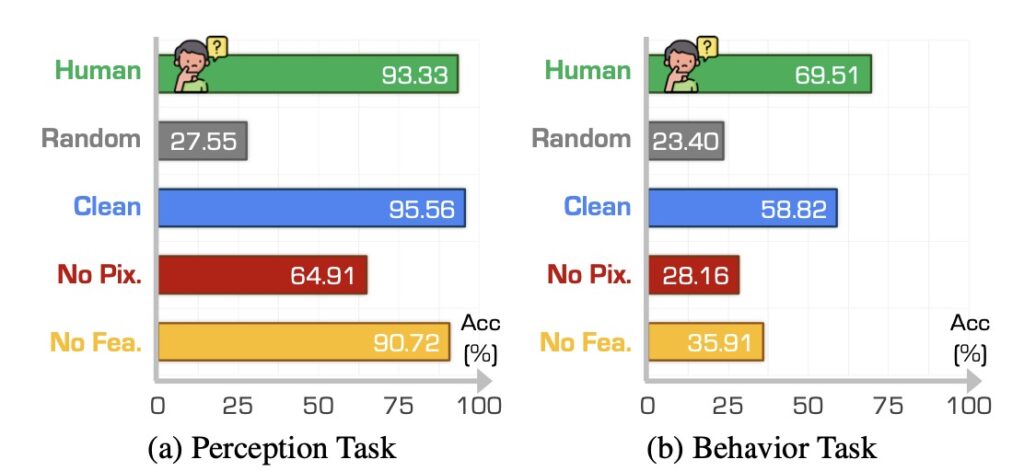
Unpacking the DriveBench Dataset
DriveBench is designed to rigorously evaluate VLMs across 17 input scenarios, encompassing clean, corrupted, and text-only settings. With over 19,000 frames and 20,000 question-answer pairs, it examines key tasks like visual grounding and multi-modal reasoning. Findings indicate that VLMs often fabricate explanations when visual cues are insufficient, undermining trust in their decision-making capabilities. This limitation is particularly alarming in autonomous driving, where errors can have life-threatening consequences.
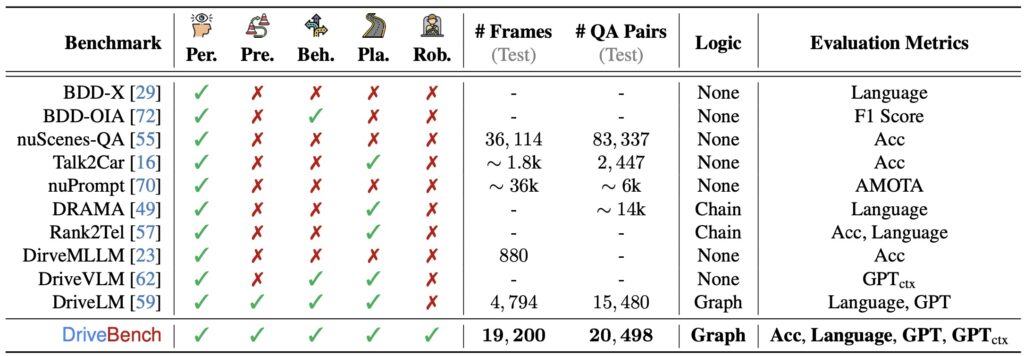
Key Challenges in VLM Deployment
- Reliability Under Degraded Inputs: VLMs display heightened sensitivity to visual corruption, often producing inconsistent or erroneous outputs.
- Limited Multi-Modal Reasoning: Despite advancements, many models struggle to combine visual and textual data for nuanced decision-making.
- Benchmark Gaps: Current evaluation frameworks often fail to capture the complexity of real-world scenarios, limiting their effectiveness in safety-critical applications.
The study also highlights a broader issue: VLMs’ tendency to generate plausible yet incorrect outputs. This behavior, though less impactful in general applications, becomes a critical liability in autonomous driving and other cyber-physical systems.
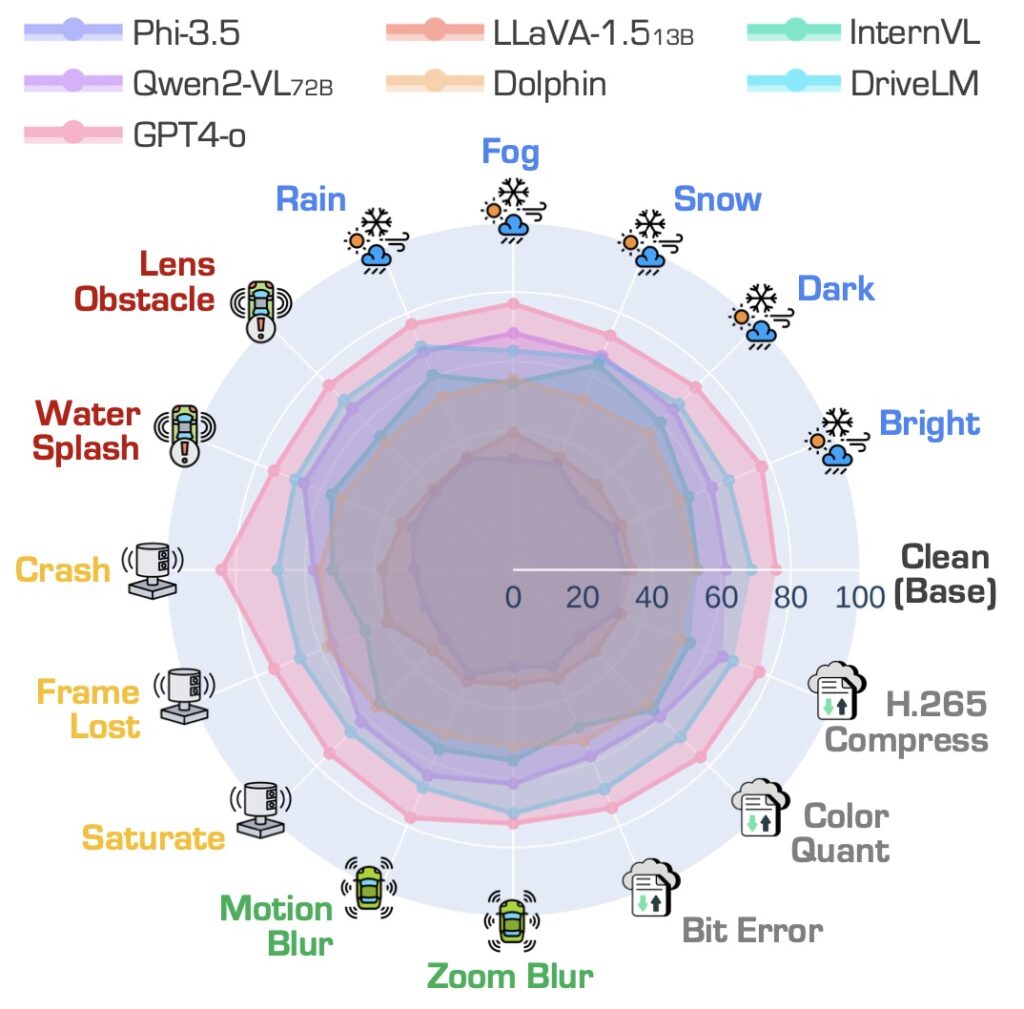
A Roadmap for Improvement
To address these challenges, the researchers propose refined metrics prioritizing visual grounding and robustness. They also emphasize leveraging VLMs’ awareness of input corruptions to improve reliability. Expanding datasets to include richer temporal and spatial contexts could further enhance evaluation fidelity, enabling VLMs to better handle real-world scenarios.
Additionally, integrating these findings into action-oriented tasks like trajectory prediction could bridge the gap between language-based evaluations and practical applications. As VLMs evolve, these improvements will be essential for safely deploying AI in autonomous systems.
Implications Beyond Driving
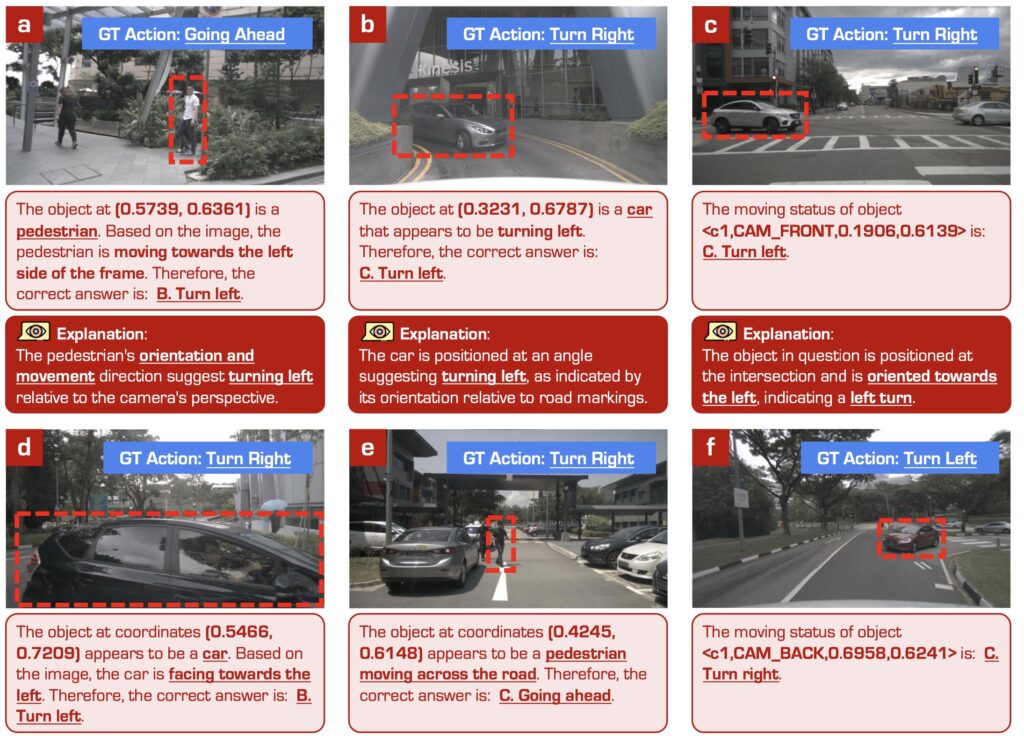
While the study focuses on autonomous driving, its insights extend to other domains like robotics and safety-critical systems. VLMs used in these areas may face similar challenges, generating misleading explanations or actions based on hallucinated understanding. The urgency of addressing these limitations cannot be overstated, particularly in systems where malfunctions could compromise safety and reliability.
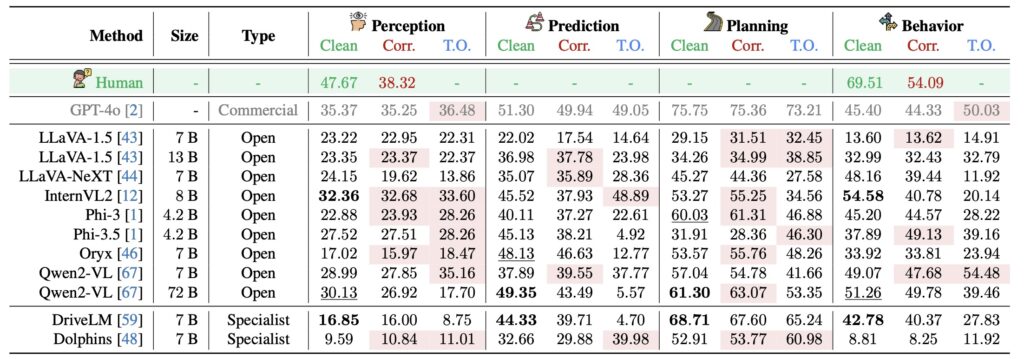
Are VLMs Ready?
The potential of Vision-Language Models in autonomous driving is undeniable, offering a promising avenue for interpretable AI. However, the findings from the DriveBench study underscore the need for robust benchmarks, enhanced datasets, and refined metrics to ensure their reliability. Until these gaps are addressed, VLMs remain a work in progress, requiring careful oversight before they can be fully integrated into safety-critical systems.
By building on these insights, researchers and developers can pave the way for more trustworthy and effective AI applications across diverse domains.