Researchers introduce a novel approach to 3D-aware image generation by leveraging 2D diffusion models and depth information from still images.
In a recent paper, researchers Jianfeng Xiang, Jiaolong Yang, Binbin Huang, and Xin Tong have introduced an innovative 3D-aware image generation method that harnesses the power of 2D diffusion models. The team formulated the 3D-aware image generation task as a sequential unconditional-conditional multiview image generation process, enabling the use of 2D diffusion models to enhance the generative modeling capabilities of their method.
A key aspect of this approach is the incorporation of depth information from monocular depth estimators. This enables the construction of training data for the conditional diffusion model using only still images. The method was trained on a large-scale dataset, ImageNet, which has not been tackled by previous methods in this field.
The results of this research show significant improvements over prior methods, producing high-quality images that demonstrate the method’s ability to generate instances with large view angles. This is particularly noteworthy given that the training images used were diverse, unaligned, and gathered from real-world “in-the-wild” environments.
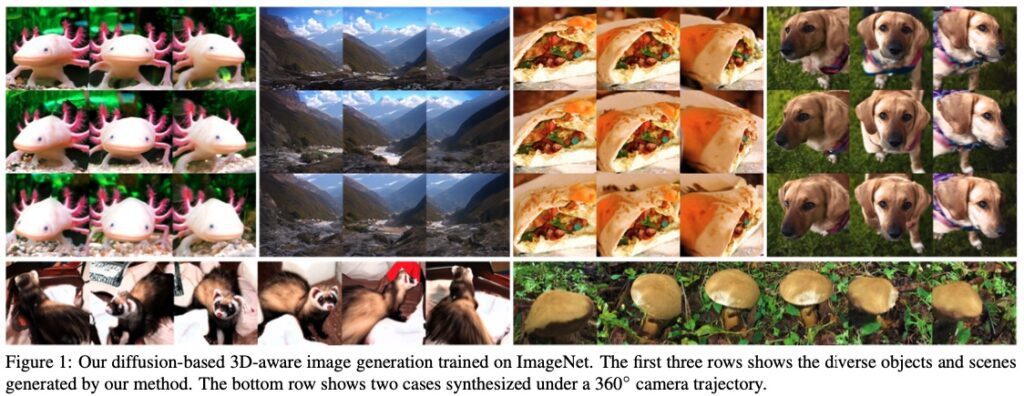
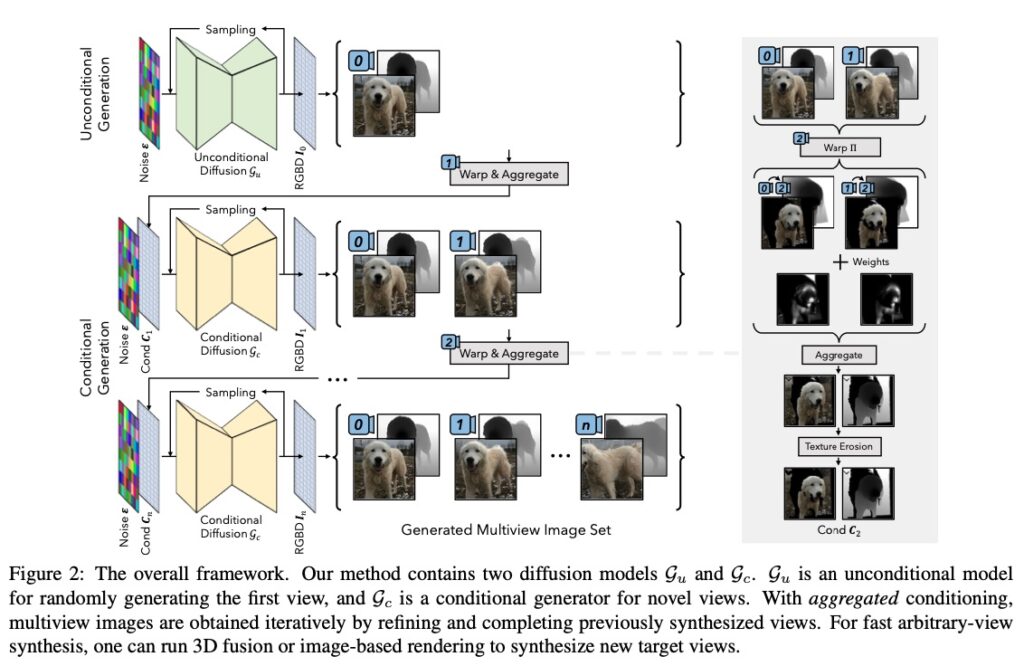
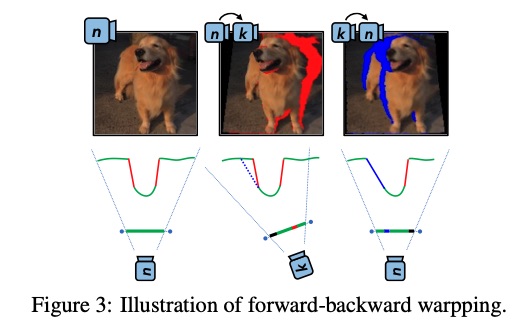
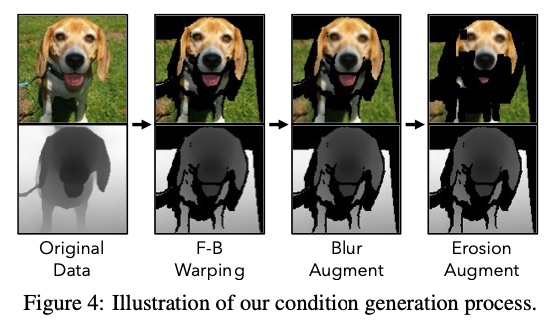
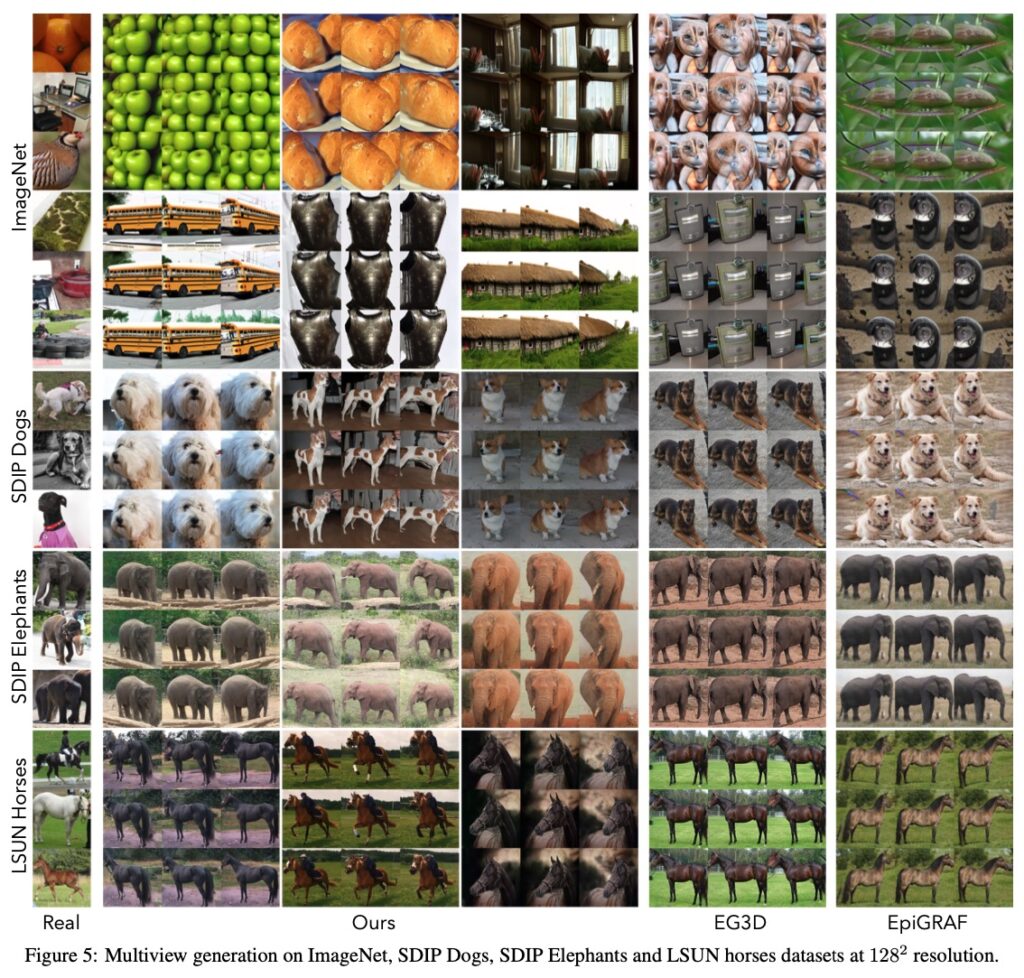
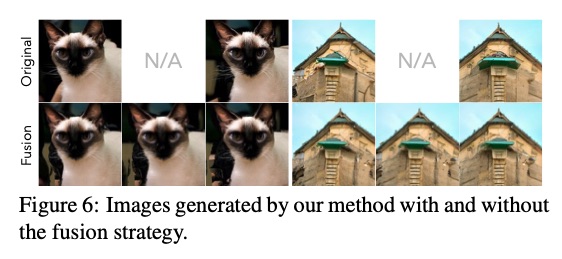
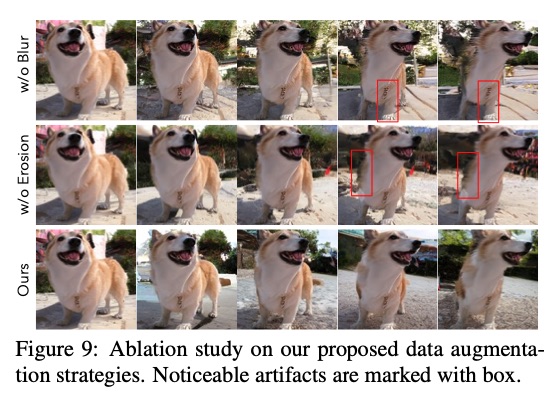
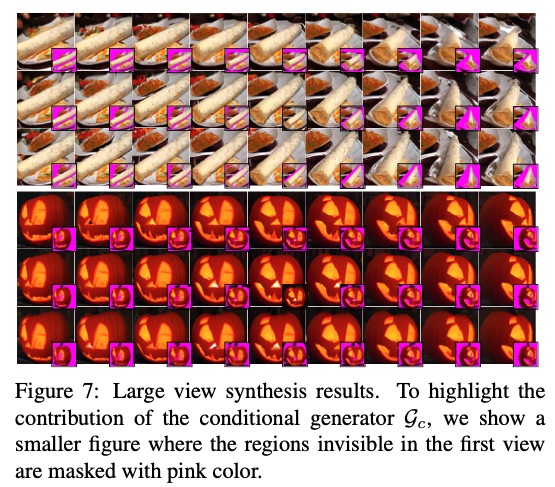
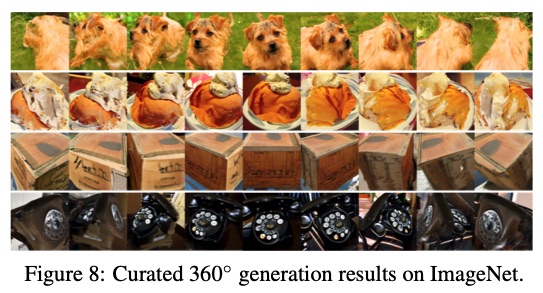
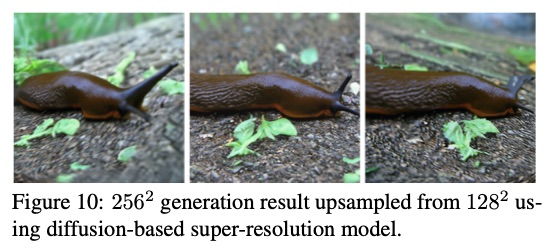
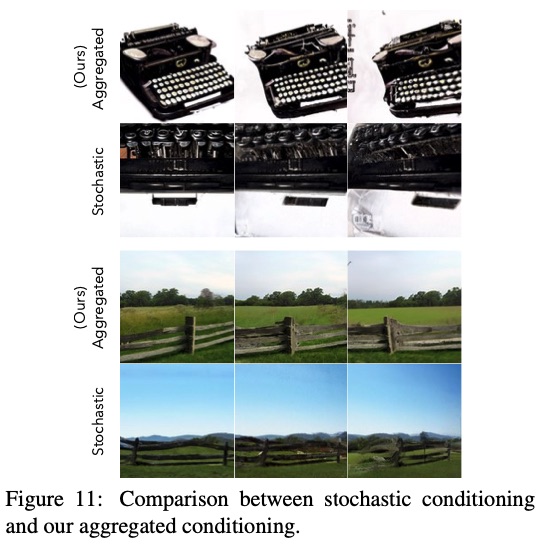
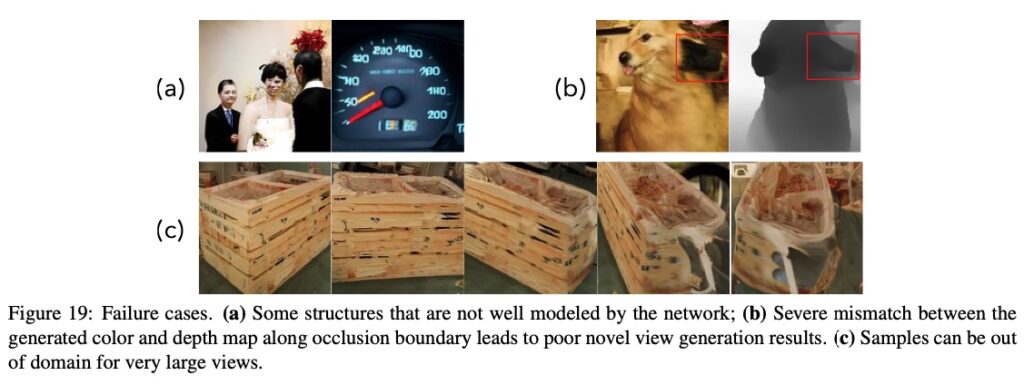
The researchers have presented a groundbreaking method for 3D-aware image generative modeling that successfully combines depth information with 2D diffusion models. The promising results on both large-scale multi-class datasets, such as ImageNet, and complex single-category datasets showcase the robust generative modeling power of the proposed method. This research could have far-reaching implications for future advancements in 3D-aware image generation and related applications.
Paper: https://arxiv.org/abs/2303.17905
Official Website: https://jeffreyxiang.github.io/ivid/