Revolutionizing AI with a Mixture-of-Experts Approach
- DeepSeek-V3, a 671B parameter Mixture-of-Experts (MoE) model, outperforms open-source models and rivals closed-source giants like GPT-4o and Claude-3.5-Sonnet.
- Innovative architectures and training strategies, including Multi-head Latent Attention (MLA) and an auxiliary-loss-free load balancing approach, enable efficient inference and cost-effective training.
- Pre-trained on 14.8 trillion tokens and enhanced through Supervised Fine-Tuning and Reinforcement Learning, DeepSeek-V3 sets new standards in language model performance across diverse tasks.
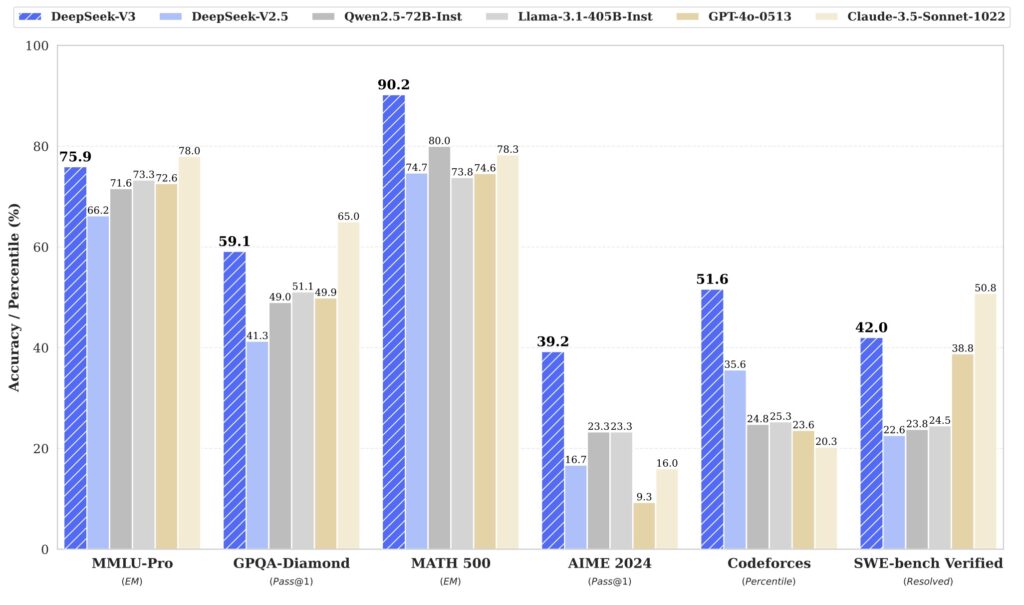
DeepSeek-V3 represents a significant leap forward in the field of artificial intelligence, introducing a powerful Mixture-of-Experts (MoE) language model that combines efficiency with exceptional performance. With a total of 671 billion parameters and 37 billion activated for each token, DeepSeek-V3 has been meticulously designed to push the boundaries of what language models can achieve.
The model’s architecture builds upon the successes of its predecessor, DeepSeek-V2, incorporating Multi-head Latent Attention (MLA) and the DeepSeekMoE framework. These innovations have been thoroughly validated and refined, ensuring that DeepSeek-V3 can handle complex tasks with ease. Moreover, the model pioneers an auxiliary-loss-free strategy for load balancing, minimizing performance degradation while maintaining efficient inference. The introduction of a multi-token prediction training objective further enhances the model’s capabilities, enabling stronger performance across a wide range of applications.
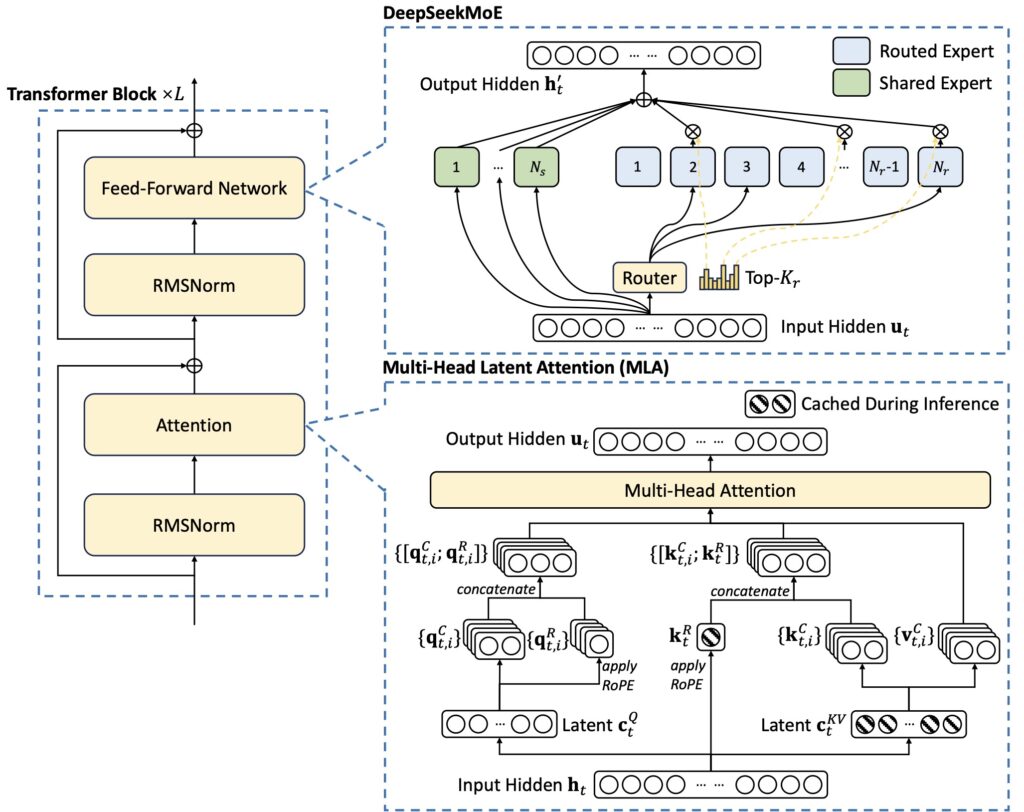
To achieve such impressive results, DeepSeek-V3 was pre-trained on an astonishing 14.8 trillion diverse and high-quality tokens. This extensive pre-training, combined with subsequent Supervised Fine-Tuning and Reinforcement Learning stages, allows the model to fully harness its potential. The result is a language model that not only outperforms other open-source models but also achieves performance comparable to leading closed-source models like GPT-4o and Claude-3.5-Sonnet.
Despite its remarkable performance, DeepSeek-V3 remains cost-effective, requiring only 2.788 million H800 GPU hours for its full training. This efficiency is made possible through the adoption of an FP8 mixed precision training framework and meticulous engineering optimizations. The training process itself is remarkably stable, with no irrecoverable loss spikes or rollbacks experienced throughout the entire journey.
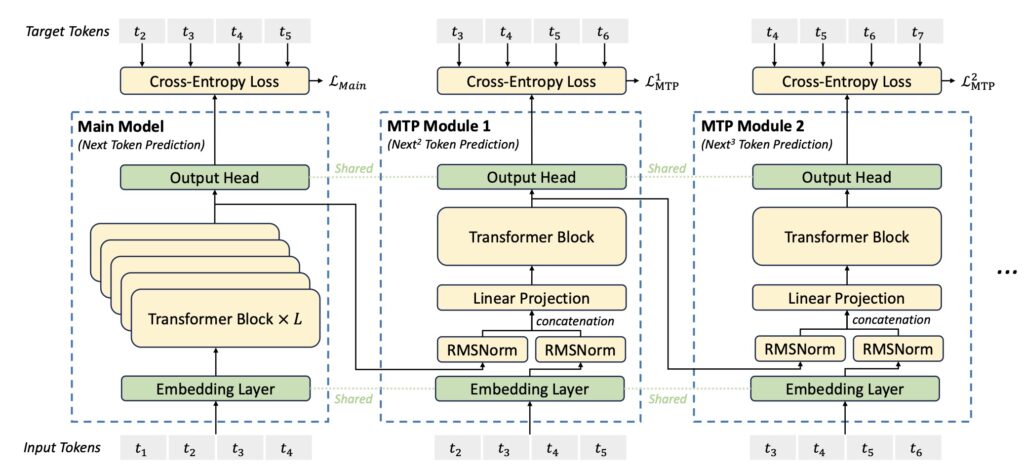
In the post-training phase, DeepSeek-V3 benefits from an innovative knowledge distillation methodology, drawing reasoning capabilities from the DeepSeek-R1 series of models. This approach elegantly incorporates the verification and reflection patterns of R1 into DeepSeek-V3, significantly improving its reasoning performance while maintaining control over output style and length.
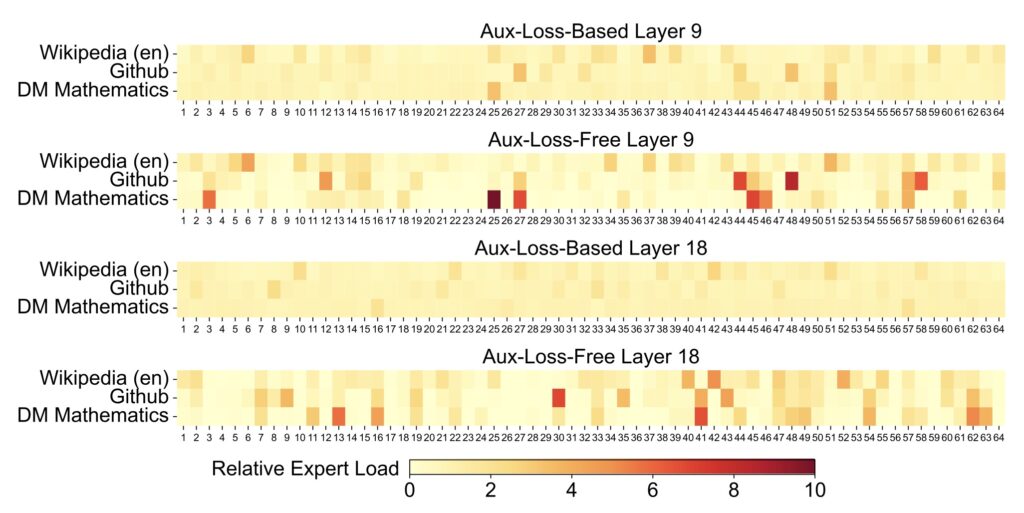
Comprehensive evaluations across various benchmarks demonstrate DeepSeek-V3’s superiority, particularly in math and code tasks. The model consistently achieves top scores, showcasing its versatility and adaptability. For those interested in exploring the model further, DeepSeek-V3 is available for download on Hugging Face, with a total size of 685 billion parameters, including the Main Model weights and the Multi-Token Prediction (MTP) Module weights.

While DeepSeek-V3 represents a significant advancement in language modeling, it is not without its limitations. The recommended deployment unit for efficient inference is relatively large, which may pose challenges for smaller teams. Additionally, although the deployment strategy has achieved an end-to-end generation speed more than twice that of DeepSeek-V2, there remains room for further enhancement. However, these limitations are expected to be addressed naturally as hardware technology continues to advance.
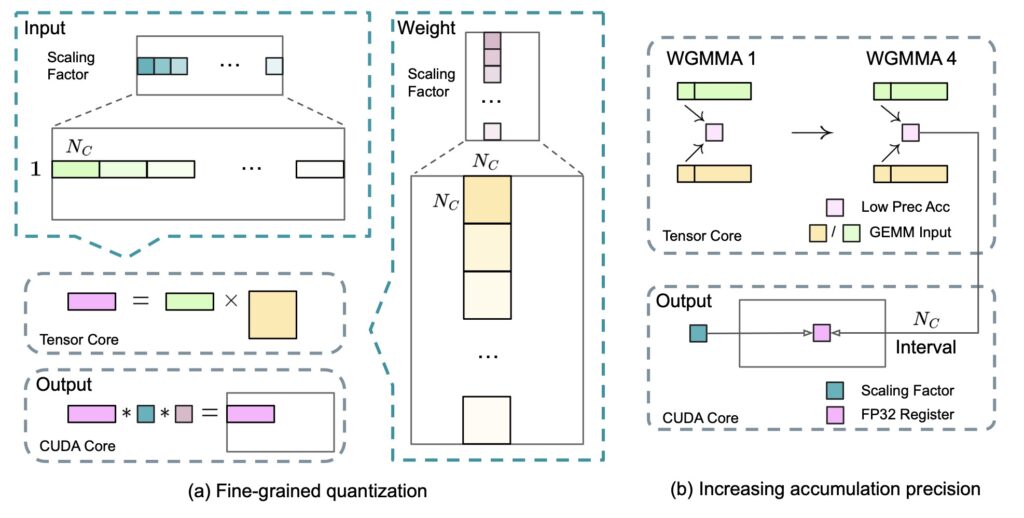
Looking to the future, DeepSeek remains committed to the path of open-source models with a long-term vision of achieving Artificial General Intelligence (AGI). The team plans to invest in research across several key areas, including refining model architectures, improving training and inference efficiency, expanding the quantity and quality of training data, enhancing deep thinking capabilities, and developing more comprehensive evaluation methods. By consistently pushing the boundaries of language modeling, DeepSeek aims to steadily approach the ultimate goal of AGI, revolutionizing the way we interact with and understand the world around us.
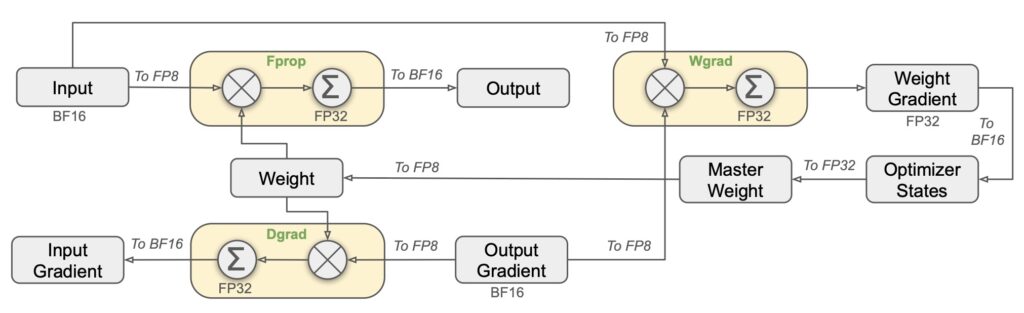
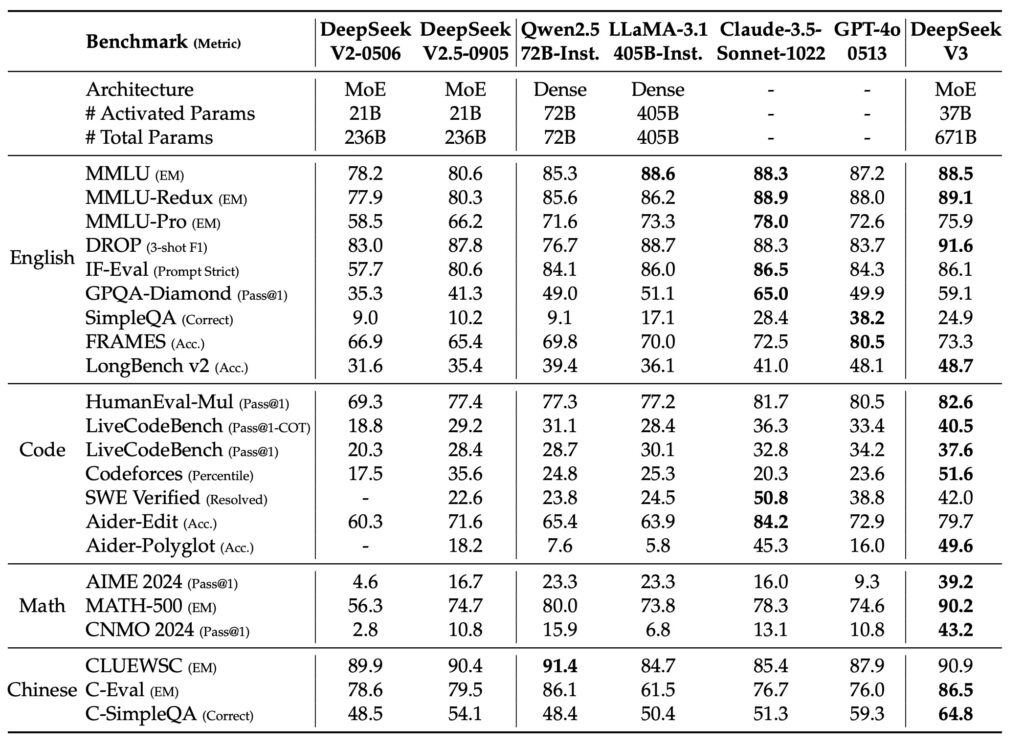