A Deep Dive into Performance, Architecture, and Limitations of OpenAI’s Breakthrough Model
- GPT-4o excels in image generation, editing, and knowledge-guided synthesis, outperforming existing models in quality and control.
- Its architecture likely combines auto-regressive (AR) planning with a diffusion-based decoder, challenging assumptions about VAR-like designs.
- While powerful, GPT-4o has detectable flaws, including synthetic artifacts and limitations in multi-round editing compared to rivals like Gemini 2.0 Flash.
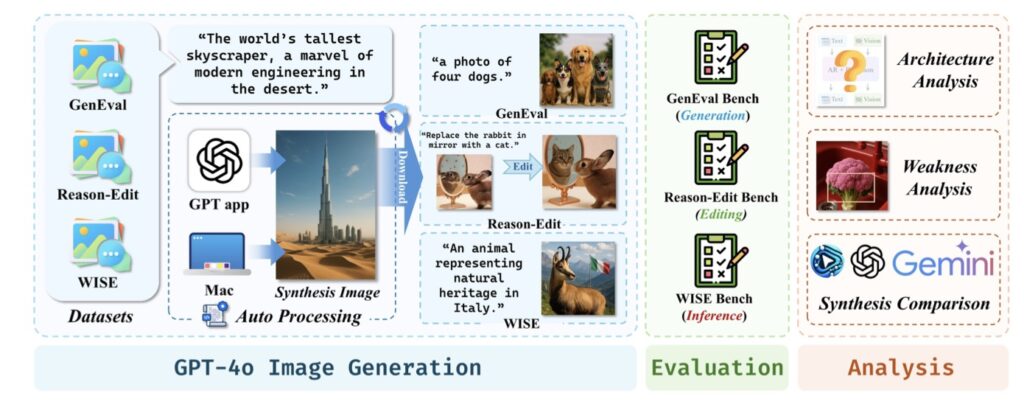
OpenAI’s GPT-4o has ignited a firestorm of excitement with its unprecedented ability to generate and refine images. But how does it really perform under the microscope? Enter GPT-ImgEval, the first comprehensive benchmark designed to dissect GPT-4o’s capabilities across three pillars: generation quality, editing proficiency, and knowledge-driven synthesis. This report not only quantifies its strengths but also decodes its hidden architecture, exposes its weaknesses, and pits it against competitors like Gemini 2.0 Flash. Let’s dive in.
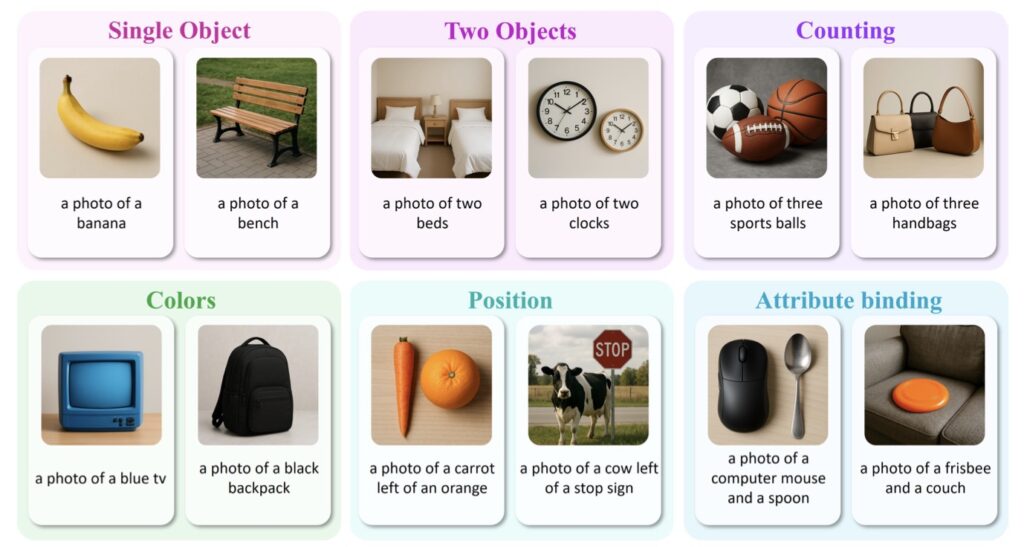
Generation Quality: Setting a New Gold Standard
Using the GenEval dataset, GPT-ImgEval evaluates GPT-4o’s ability to produce high-fidelity, coherent images. The results are staggering:
- Detail Accuracy: GPT-4o generates intricate textures and lifelike shadows, surpassing models like DALL-E 3 in photorealism.
- Prompt Adherence: Whether rendering “a Victorian library with floating books” or “a cyberpunk cityscape,” the model demonstrates near-flawless alignment with user instructions.
- Consistency: Multi-object scenes (e.g., “a wolf pack hunting in a snowstorm”) show minimal anatomical errors, a notorious weak spot for earlier AI systems.
This leap in quality suggests GPT-4o has mastered the interplay between semantic understanding and visual representation—a critical step toward human-like creative reasoning.
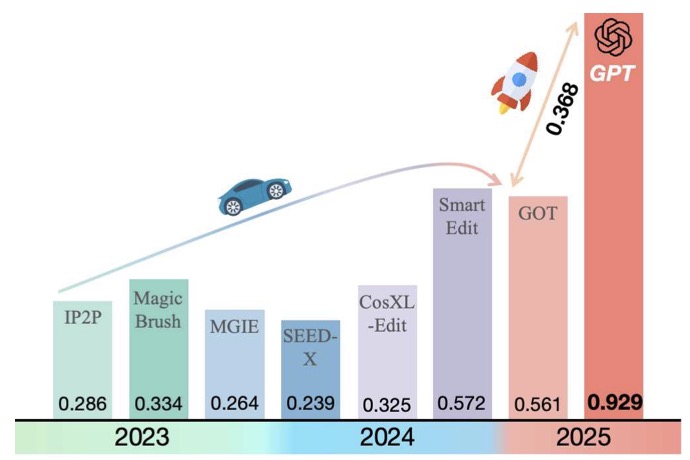
Precision Meets Creativity
The Reason-Edit dataset tests GPT-4o’s ability to modify images iteratively. From altering lighting conditions to swapping objects in complex scenes, the model shines:
- Context Preservation: When asked to “replace the medieval castle with a skyscraper,” it retains surrounding details like terrain and weather effects.
- Multi-Round Refinement: GPT-4o handles cascading edits (e.g., “make the dog larger, then add a collar”) with remarkable coherence—though Gemini 2.0 Flash edges ahead in maintaining long-term context over 5+ edits.
- Error Correction: It self-corrects artifacts (e.g., mismatched shadows) more effectively than diffusion-only models.
This proficiency hints at a hybrid architecture where AR-based planning guides iterative adjustments before a diffusion decoder executes them.
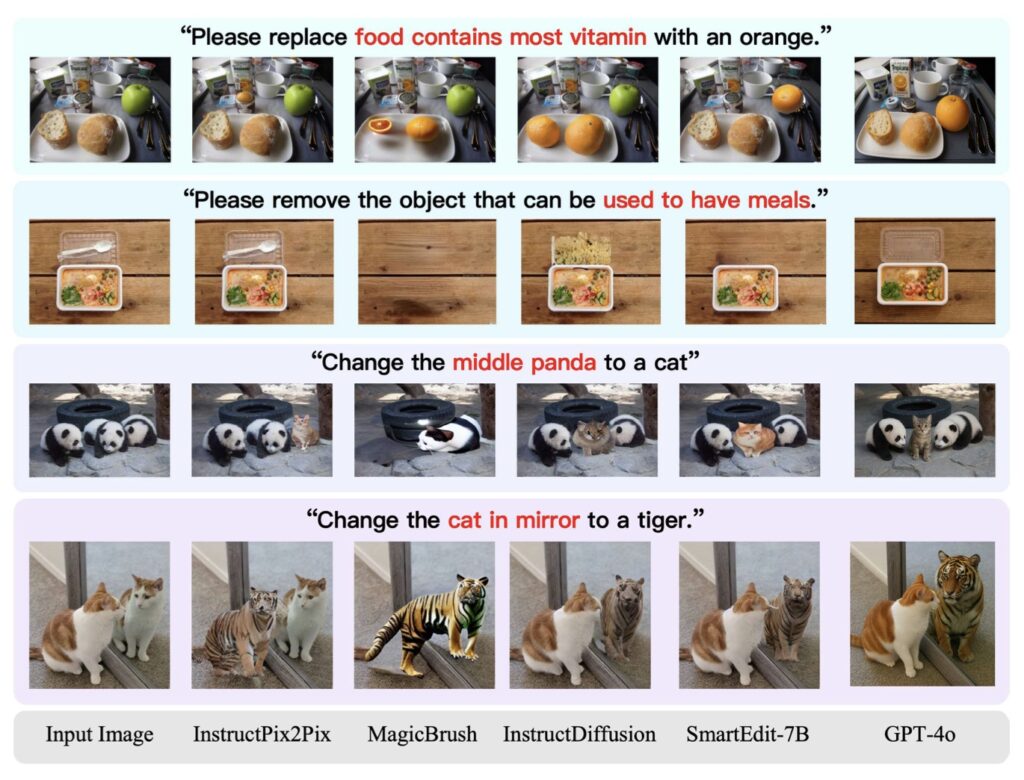
Knowledge-Guided Synthesis: Bridging Facts and Imagination
The WISE dataset evaluates GPT-4o’s ability to synthesize images informed by real-world knowledge:
- Cultural Nuance: Generating “a traditional Japanese tea ceremony” includes accurate utensils and clothing details.
- Scientific Accuracy: Depictions of “a Mars rover with solar panels” align with NASA design principles.
- Temporal Awareness: Scenes like “a 1920s jazz club” reflect period-appropriate fashion and architecture.
Such results imply GPT-4o’s training data includes cross-modal knowledge graphs, enabling it to fuse textual facts with visual creativity.
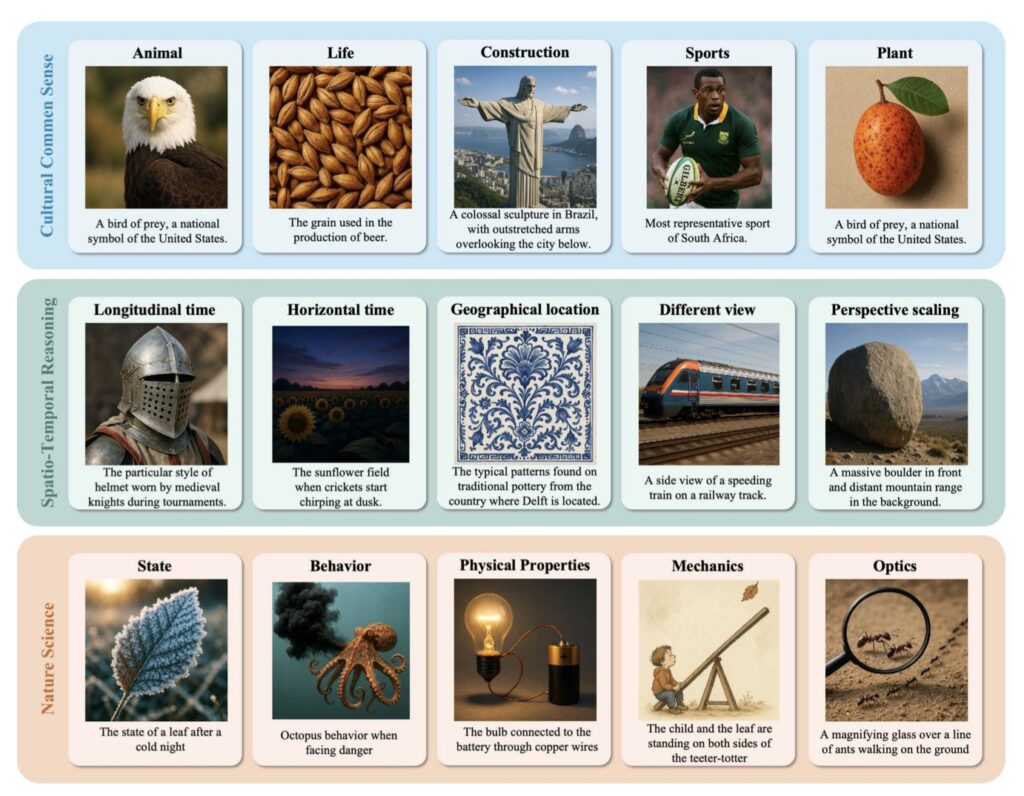
Under the Hood: Decoding GPT-4o’s Architecture
By analyzing GPT-4o’s outputs, researchers propose that its image generation pipeline combines:
- Auto-Regressive (AR) Component: Plans the image structure and semantic layout step-by-step.
- Diffusion-Based Decoder: Converts this “blueprint” into high-resolution pixels.
This hybrid design explains its superior control over purely diffusion-based models (e.g., Stable Diffusion) and VAR architectures. Empirical tests using classifier probes reveal AR-driven token prediction in early layers, followed by diffusion denoising—a structure optimized for both coherence and detail.
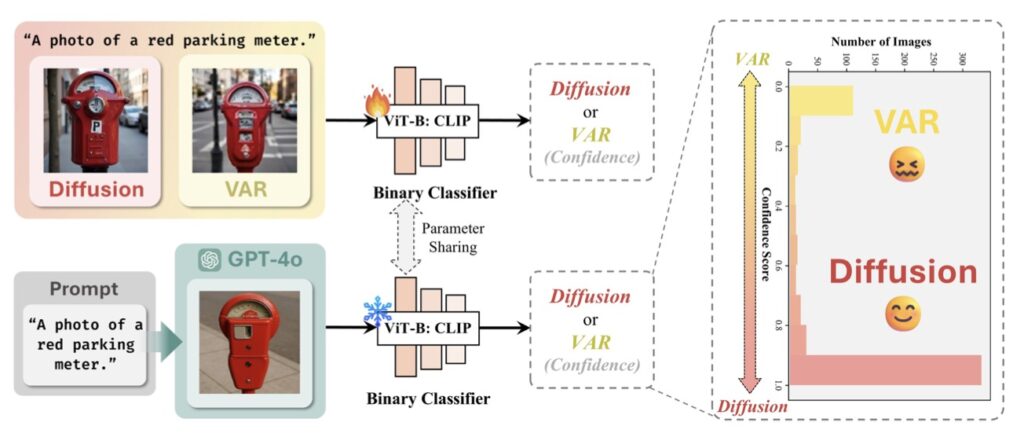
Cracks in the Armor: Limitations and Artifacts
Despite its prowess, GPT-ImgEval uncovers flaws:
- Synthetic Signatures: Over-smooth textures in organic materials (e.g., fur, wood grain) and inconsistent reflections in metallic surfaces.
- Conceptual Blind Spots: Struggles with rare combinations (e.g., “an octopus playing a piano”) or highly abstract prompts.
- Safety Risks: Over 30% of GPT-4o’s outputs evade detection by standard forensic tools, raising concerns about misuse.
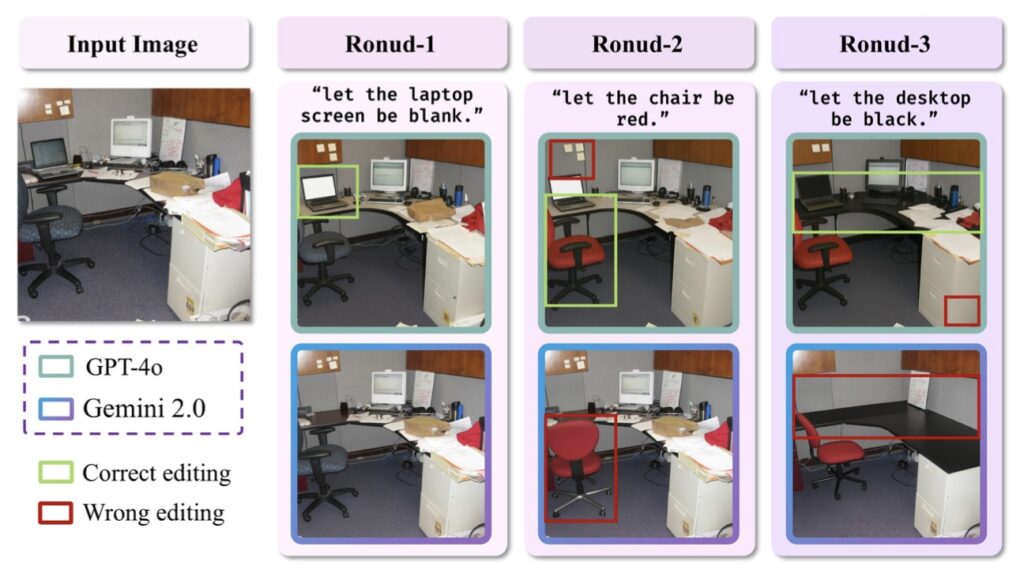
The Gemini Rivalry: Multi-Round Editing Showdown
In head-to-head tests with Gemini 2.0 Flash, GPT-4o trails slightly in editing stamina. While both models handle 3-4 iterative changes flawlessly, Gemini maintains context better beyond five edits, particularly in scenes requiring positional reasoning (e.g., moving objects in 3D space).
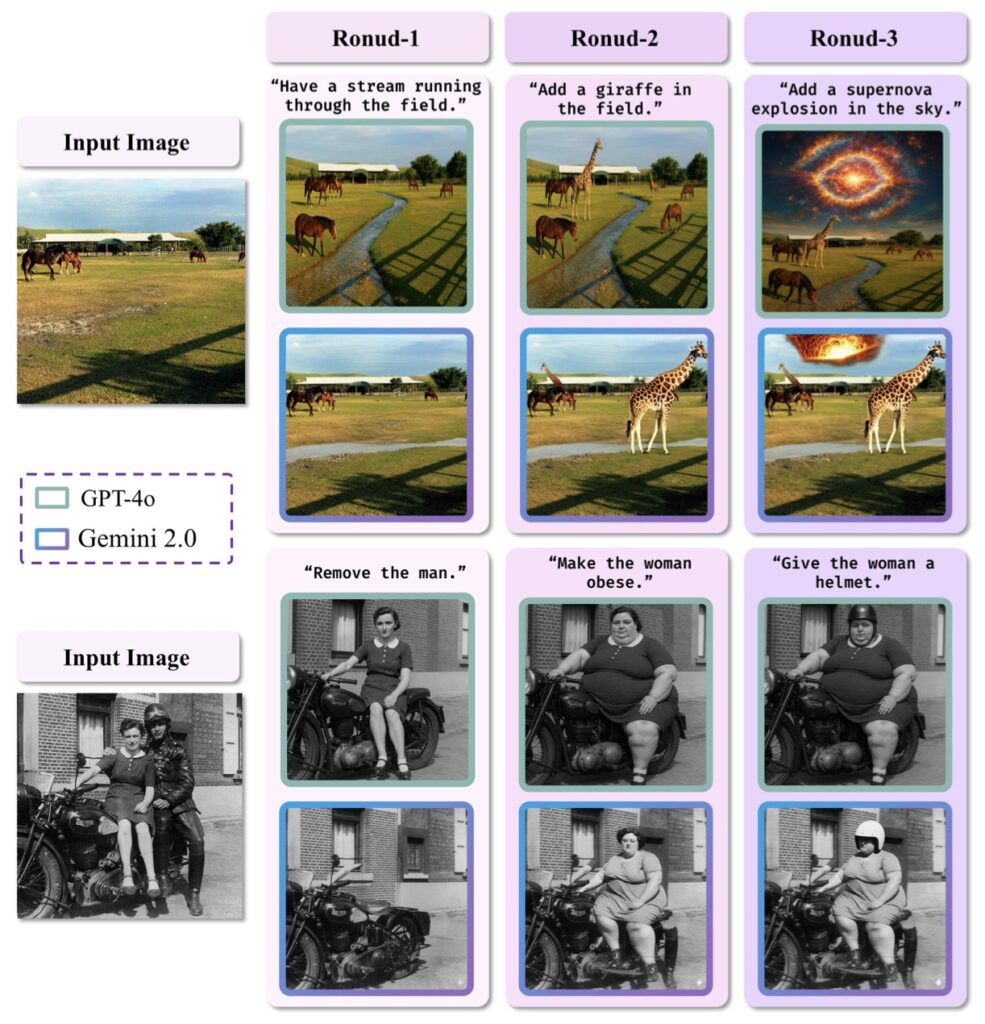
Charting the Future of AI-Generated Imagery
GPT-ImgEval isn’t just a report card—it’s a roadmap. By exposing GPT-4o’s strengths, architecture, and vulnerabilities, this benchmark empowers researchers to build safer, more transparent models. As AI art races toward indistinguishability from human creativity, tools like GPT-ImgEval will be vital in ensuring innovation doesn’t outpace accountability.