Balancing Identity Preservation and Personalized Editing in 2D Generative Models
- FlexIP introduces a groundbreaking framework that decouples identity preservation and stylistic manipulation in 2D image generation, addressing the long-standing trade-offs in existing methods.
- Through its dual-adapter architecture—comprising a Personalization Adapter and a Preservation Adapter—FlexIP ensures high-fidelity subject identity while enabling diverse, user-controlled editing.
- Experimental results showcase FlexIP’s superior performance, offering a flexible, parameterized control mechanism that transforms image synthesis for artistic and commercial applications.
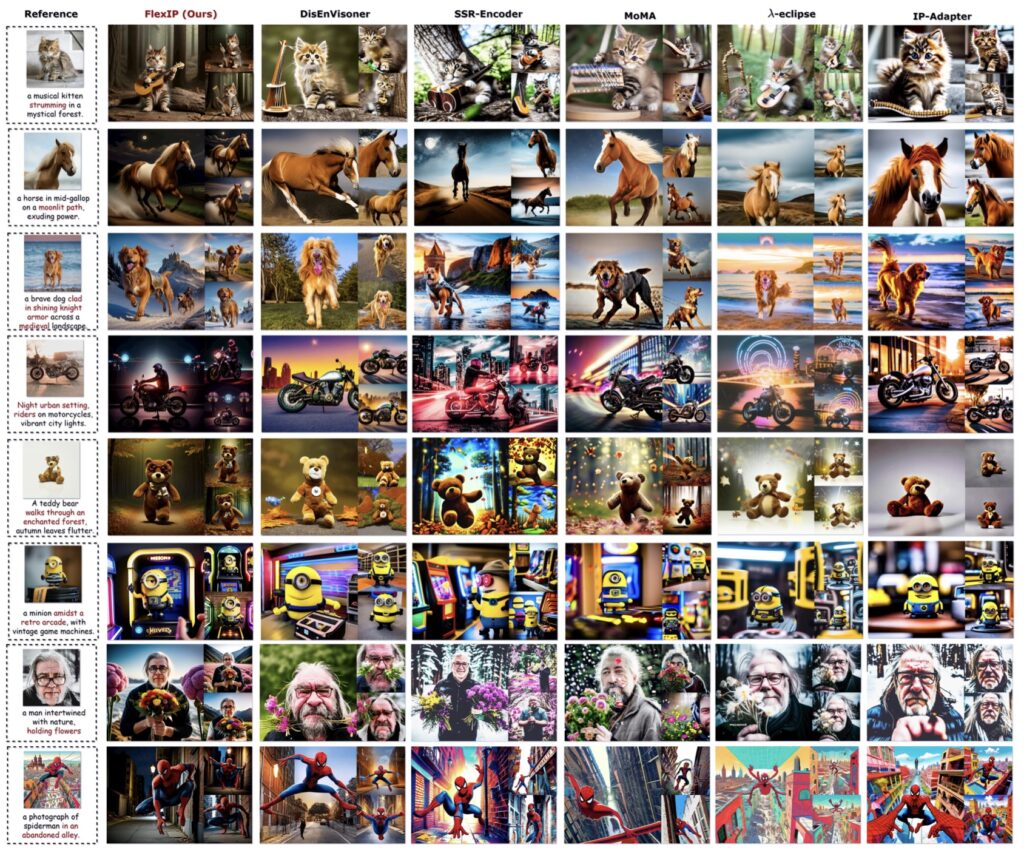
The world of 2D generative models has seen remarkable strides in recent years, with diffusion models leading the charge in image synthesis and editing technologies. These advancements have unlocked unprecedented potential in fields like artistic creation and advertising design, where high-quality, diverse visual content is paramount. However, a persistent challenge has plagued subject-driven image generation: the inherent conflict between preserving a subject’s identity and enabling personalized, flexible editing. Traditional approaches often force a compromise, sacrificing one for the other. Enter FlexIP, a novel framework that redefines the landscape by decoupling these objectives and offering dynamic, user-controlled solutions for customized image generation.
At the heart of FlexIP lies its innovative dual-adapter architecture, which tackles the core limitations of prior methods. Current research in subject-driven image generation typically follows two paradigms: inference-time fine-tuning and zero-shot image-based customization. Fine-tuning methods learn pseudo-words as compact subject representations through per-subject optimization, achieving high-fidelity reconstruction but often overfitting to narrow feature manifolds, thus limiting editing flexibility. On the other hand, zero-shot methods leverage cross-modal alignment modules without subject-specific fine-tuning, providing greater editing freedom but frequently failing to maintain identity integrity. FlexIP transcends these constraints by introducing two dedicated components: a Personalization Adapter for stylistic manipulation and a Preservation Adapter for identity maintenance. This separation allows the framework to address each objective independently, ensuring that neither is sacrificed at the expense of the other.
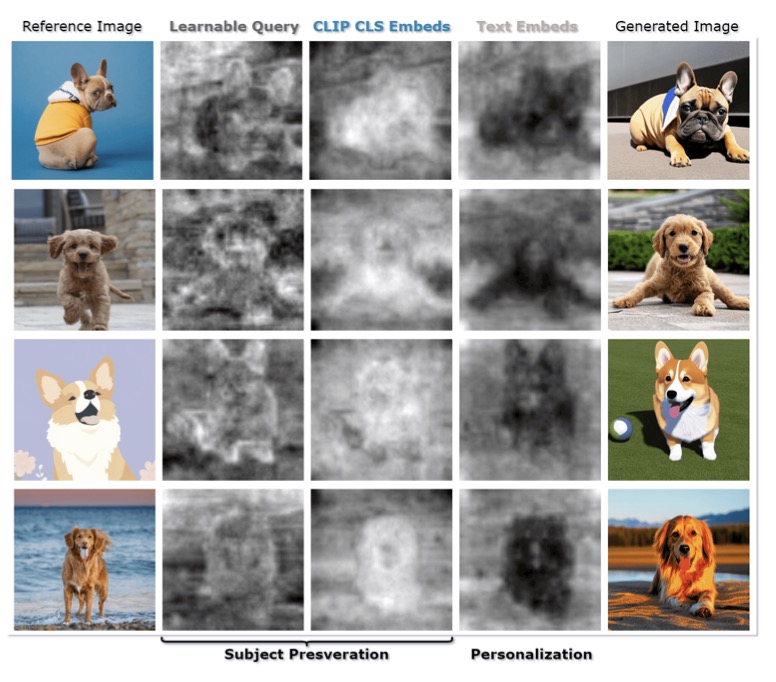
What sets FlexIP apart is its ability to transform the binary trade-off between preservation and personalization into a continuous, parameterized control surface. Through a dynamic weight gating mechanism, users can fine-tune the balance between identity preservation and stylistic editing during inference. This means that whether the goal is to maintain the intricate details of a subject’s identity—using high-level semantic concepts and low-level spatial details—or to explore bold, creative manipulations, FlexIP offers unparalleled flexibility. The framework explicitly injects both control mechanisms into the generative model, empowering users to navigate the spectrum of possibilities with ease. This dynamic tuning capability marks a significant departure from conventional methods, which often lock users into predefined trade-offs.
The implications of FlexIP’s approach are profound, particularly in practical applications. In artistic creation, for instance, designers can preserve the essence of a character or subject while experimenting with diverse styles and aesthetics, ensuring that the final output remains true to the original vision. In advertising design, where brand identity and creative innovation must coexist, FlexIP enables the generation of visually striking content that retains recognizable elements of a product or spokesperson. Experimental results further validate the framework’s effectiveness, demonstrating that it not only breaks through the performance limitations of existing methods but also achieves superior identity preservation alongside expansive personalized generation capabilities. This balance positions FlexIP as a robust and versatile solution for subject-driven image synthesis.
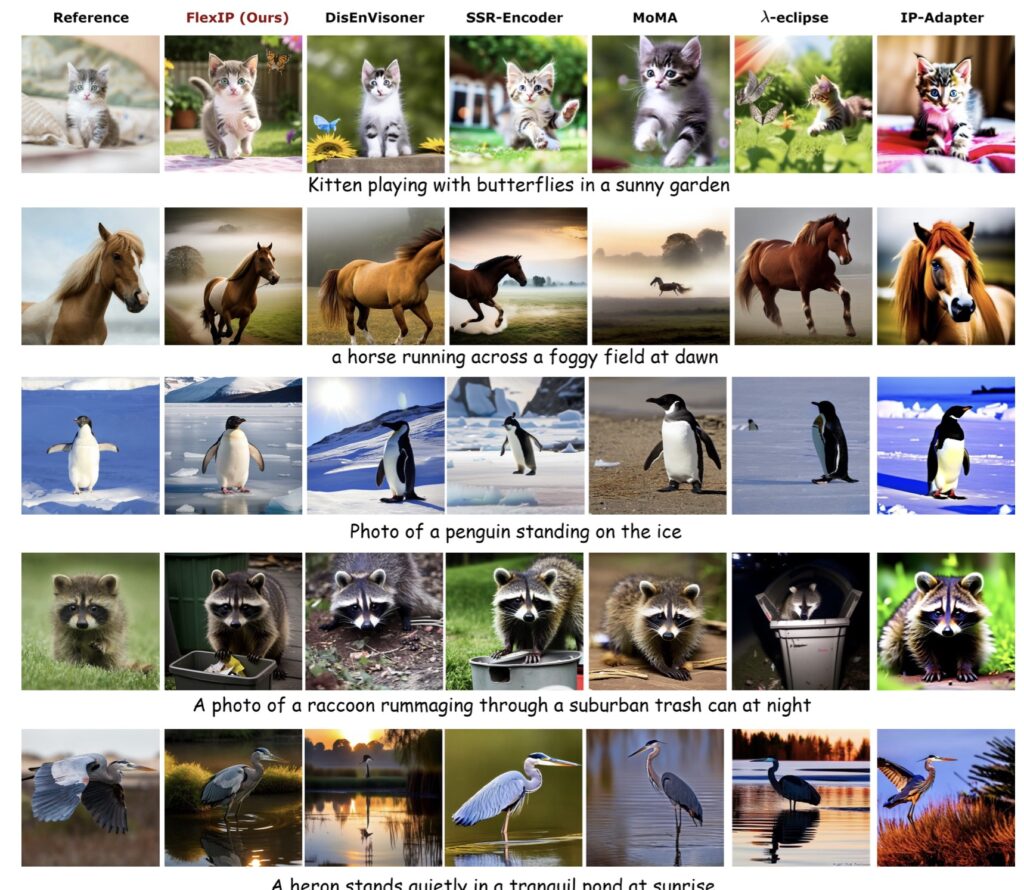
Looking at the broader perspective, FlexIP represents a pivotal step forward in the evolution of 2D generative models. The rapid progress of diffusion models has already reshaped how we approach visual content creation, but challenges like the identity-editing trade-off have hindered their full potential. By decoupling these competing objectives and introducing a flexible control mechanism, FlexIP paves the way for more intuitive and powerful tools in the hands of creators and innovators. It shifts the paradigm from a rigid, either-or scenario to a fluid, user-defined experience, where the boundaries of creativity are limited only by imagination. As generative technologies continue to advance, frameworks like FlexIP will likely serve as the foundation for future innovations, driving the next wave of breakthroughs in image synthesis and beyond.
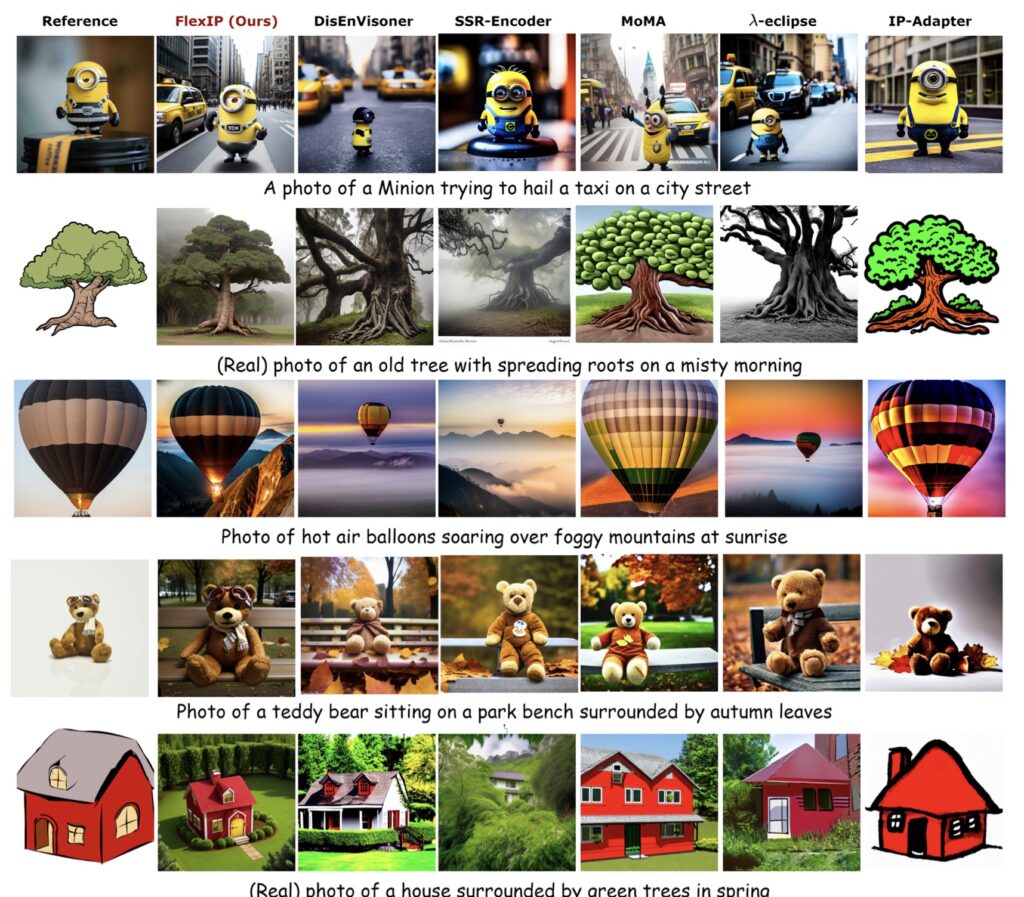
In conclusion, FlexIP stands as a beacon of progress in the realm of customized image generation. Its dual-adapter architecture, dynamic control mechanisms, and ability to balance identity preservation with personalized editing make it a game-changer for both artistic and commercial applications. By overcoming the inherent limitations of traditional methods, FlexIP not only enhances the quality and diversity of generated content but also empowers users with unprecedented control over the creative process. As we move forward in the era of generative AI, FlexIP offers a glimpse into a future where technology and creativity converge seamlessly, unlocking new possibilities for visual storytelling and design.