Harnessing Video Diffusion for Temporally Consistent Surface Normals
- NormalCrafter introduces a groundbreaking approach to surface normal estimation in videos, leveraging video diffusion priors to ensure temporal consistency across sequences.
- The method employs Semantic Feature Regularization (SFR) and a two-stage training protocol to enhance spatial accuracy and preserve intricate details in diverse, unconstrained videos.
- Extensive evaluations showcase NormalCrafter’s state-of-the-art performance in zero-shot settings, paving the way for advancements in 3D reconstruction, relighting, and mixed reality applications.

Surface normal estimation, a fundamental task in computer vision, acts as a building block for understanding 3D scene geometry. From enabling precise 3D reconstructions to powering immersive mixed reality experiences, surface normals are indispensable in applications like video editing and relighting. However, while static image-based normal estimation has seen significant progress, achieving temporal coherence in video sequences remains a daunting hurdle. Variations in scene layouts, lighting conditions, camera movements, and dynamic elements often disrupt consistency across frames, leading to inaccuracies. Enter NormalCrafter, a pioneering solution that redefines video normal estimation by tapping into the inherent strengths of video diffusion models.
Unlike traditional approaches that merely adapt static methods with temporal add-ons, NormalCrafter takes a bold step forward by integrating temporal priors from video diffusion models. This innovative framework ensures that normal sequences remain consistent over time, even in the face of complex, open-world videos. By focusing on the intrinsic temporal patterns embedded in video data, NormalCrafter addresses the core challenge of maintaining coherence across frames, delivering results that are not only accurate but also visually seamless. This approach marks a significant departure from conventional methods, offering a fresh perspective on how temporal dynamics can be harnessed for better outcomes in computer vision tasks.
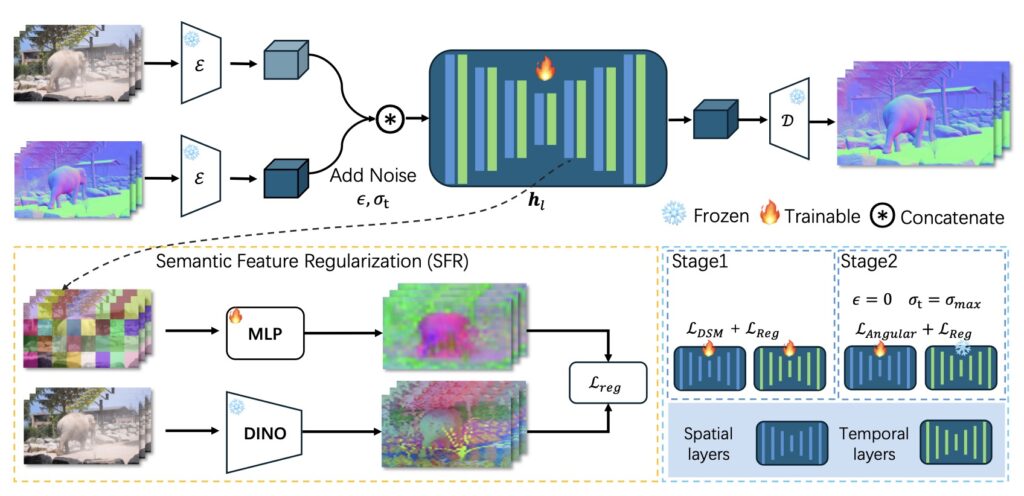
A key pillar of NormalCrafter’s success lies in its use of Semantic Feature Regularization (SFR). This technique aligns diffusion features with semantic cues, guiding the model to focus on the underlying semantics of a scene rather than getting lost in superficial variations. Whether it’s a bustling urban street or a serene natural landscape, SFR ensures that the model captures the essence of the environment, resulting in high-fidelity normal estimations that retain fine-grained details. This semantic alignment is particularly crucial for handling the diversity of real-world videos, where unpredictable changes in illumination or motion can easily throw off less sophisticated systems.
Complementing SFR is NormalCrafter’s two-stage training protocol, a meticulously designed strategy that balances spatial accuracy with long-term temporal context. In the first stage, the model learns in latent space, grasping the broader patterns and relationships within the video data. The second stage shifts to pixel space, refining the details to ensure precision at a granular level. This dual approach allows NormalCrafter to excel in both capturing the overarching flow of a sequence and preserving the minute intricacies that make each frame unique. The result is a normal estimation system that performs robustly across a wide range of scenarios, from subtle indoor settings to dynamic outdoor environments.
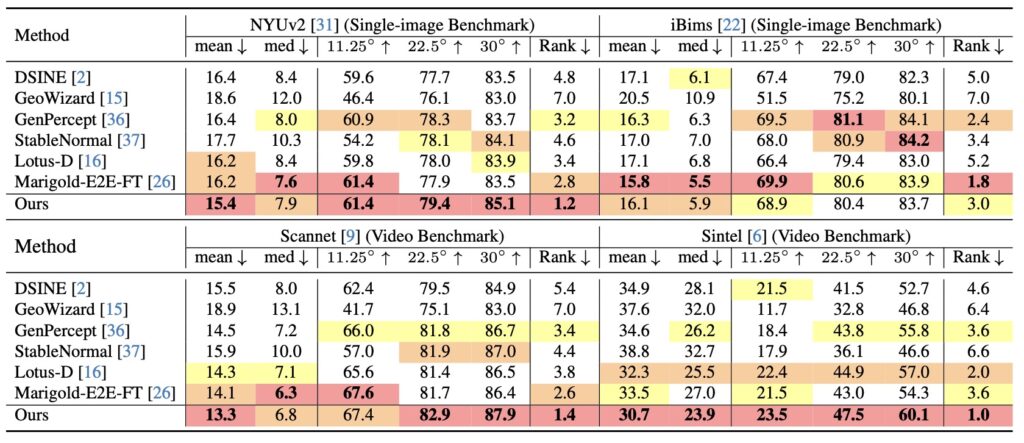
The implications of NormalCrafter’s capabilities are far-reaching. In 3D reconstruction, for instance, temporally consistent normals can significantly improve the quality of models, making them more realistic and usable for practical applications. In relighting and video editing, the fine details preserved by NormalCrafter enable more natural and visually appealing modifications, enhancing creative workflows. Mixed reality, too, stands to benefit, as accurate surface normals are critical for seamlessly blending virtual and real-world elements. By tackling the challenges of unconstrained, open-world videos, NormalCrafter opens up new possibilities for these fields, pushing the boundaries of what’s achievable with video-based computer vision.
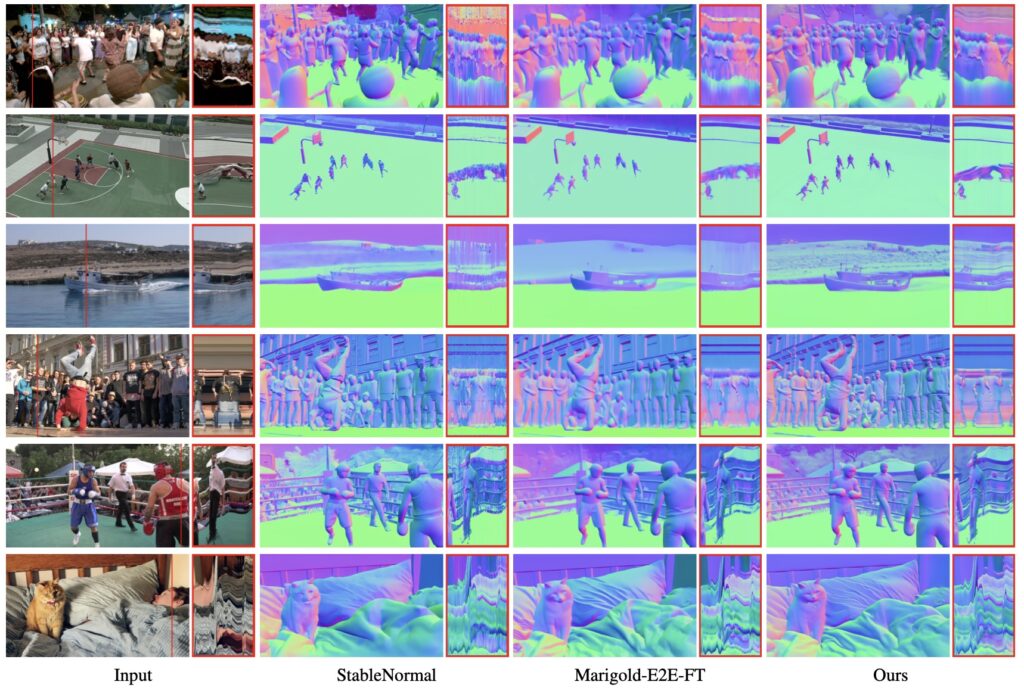
Extensive evaluations underscore the transformative potential of NormalCrafter, demonstrating its superior performance in zero-shot settings. This means the model can handle entirely new, unseen videos without requiring additional training, a testament to its robustness and adaptability. Compared to existing methods, NormalCrafter stands out for its ability to generate normal sequences that are not only temporally consistent but also rich in detail, even under the most challenging conditions. These results highlight the method’s state-of-the-art standing in the realm of video normal estimation, setting a new benchmark for future research and development.
Looking ahead, NormalCrafter serves as an inspiring foundation for further exploration in this domain. Its innovative use of video diffusion priors and strategic training protocols offers valuable insights into how temporal and spatial challenges can be addressed in tandem. As computer vision continues to evolve, solutions like NormalCrafter will play a pivotal role in bridging the gap between static and dynamic scene understanding, unlocking new levels of precision and creativity. For researchers and practitioners alike, this work is a call to rethink traditional approaches and embrace the untapped potential of video data in shaping the future of 3D vision technologies.
NormalCrafter is more than just a tool for normal estimation; it’s a visionary step toward mastering the complexities of video-based 3D scene analysis. By achieving temporal consistency and spatial accuracy in equal measure, it addresses longstanding challenges with elegance and efficacy. As we continue to explore the vast possibilities of computer vision, NormalCrafter stands as a beacon of innovation, illuminating the path toward more immersive, accurate, and dynamic digital experiences.