How a Multi-Agent System is Automating the Future of Therapeutic Innovation
- Robin, the first multi-agent AI system, fully automates the scientific discovery process by integrating literature search and data analysis to generate hypotheses, propose experiments, and interpret results.
- Applied to dry age-related macular degeneration (dAMD), Robin identified a novel treatment strategy using ripasudil, a clinically approved drug, marking a significant breakthrough in therapeutic discovery.
- Beyond dAMD, Robin’s scalable framework promises to accelerate drug development across multiple diseases, addressing the stagnation in novel FDA approvals with a semi-autonomous, lab-in-the-loop approach.
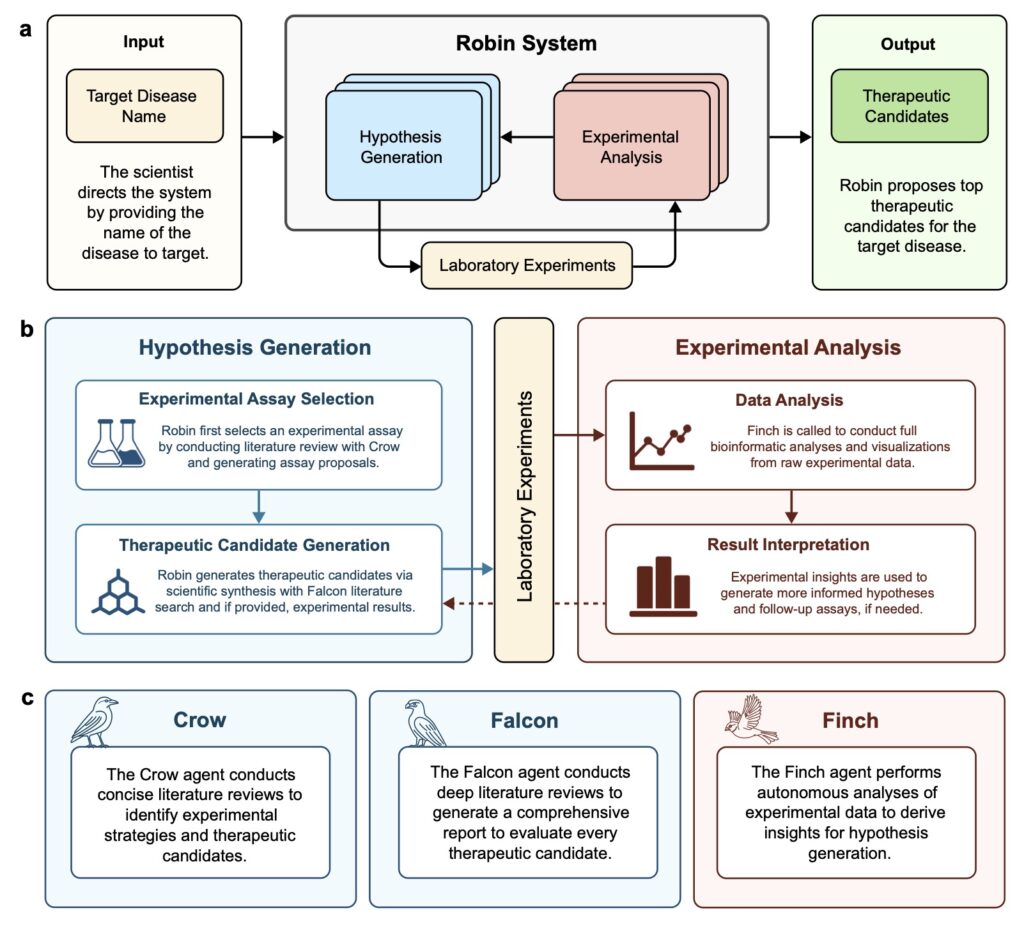
The landscape of scientific discovery is undergoing a profound transformation, driven by the integration of artificial intelligence (AI) into the traditionally human-centric process of research. At the forefront of this revolution is Robin, a pioneering multi-agent system designed to automate the iterative stages of scientific inquiry—background research, hypothesis generation, experimentation, and data analysis. Unlike previous AI tools that tackled isolated aspects of research, Robin offers a comprehensive workflow, seamlessly connecting these stages to create a semi-autonomous engine of discovery. This article explores how Robin is reshaping the future of science, with a particular focus on its groundbreaking application in identifying a novel treatment for dry age-related macular degeneration (dAMD), a leading cause of blindness in the developed world.
At its core, Robin addresses a critical gap in scientific progress: the lag in technologies to interpret, synthesize, and generate hypotheses from the ever-expanding body of knowledge. While advancements in measuring, perturbing, and modeling biological systems have fueled rapid growth in data, the ability to distill actionable insights has struggled to keep pace. Robin leverages large language models (LLMs) to bridge this gap, integrating specialized agents like Crow and Falcon for literature searches and Finch for data analysis. Crow and Falcon, built on the expert-level PaperQA2 framework, conduct concise and in-depth summaries of scientific literature, clinical trials, and platforms like Open Targets. Meanwhile, Finch tackles the complex task of analyzing experimental data from assays such as RNA-seq and flow cytometry, ensuring that insights are both reproducible and interpretable. Together, these agents enable Robin to drive scientific discovery with unprecedented efficiency.
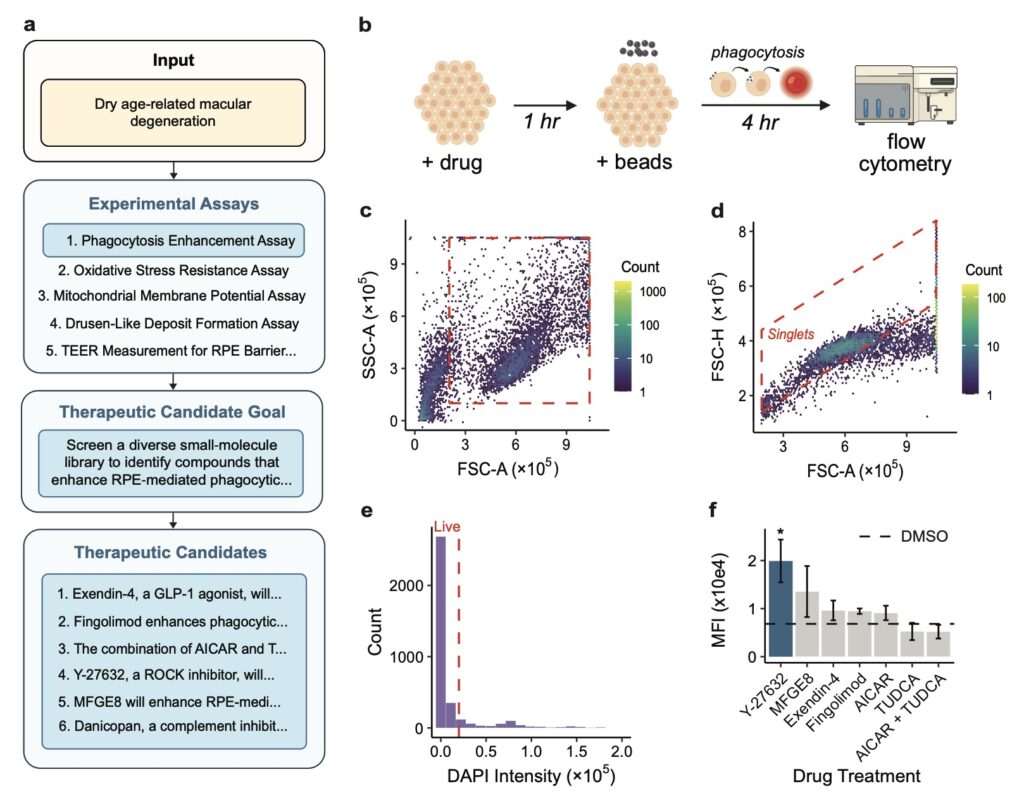
One of Robin’s most remarkable achievements is its application to dAMD, a condition with limited therapeutic options despite its devastating impact. Tasked with identifying novel treatments, Robin proposed enhancing retinal pigment epithelium (RPE) phagocytosis as a therapeutic strategy—a mechanism critical to retinal health but impaired in dAMD patients. Through its literature synthesis, Robin pinpointed ripasudil, a clinically approved rho kinase (ROCK) inhibitor, as a promising candidate to boost phagocytosis. Notably, while ROCK inhibitors have been explored for wet AMD and other retinal conditions, Robin’s hypothesis marked the first time their potential for dAMD was suggested, highlighting the system’s ability to uncover novel applications from existing knowledge. Subsequent experiments validated ripasudil’s efficacy, and Robin proposed a follow-up RNA-seq analysis that revealed upregulation of ABCA1, a lipid efflux pump, as a possible novel target. This iterative, lab-in-the-loop cycle—where hypotheses are tested, results analyzed, and new experiments proposed—demonstrates Robin’s capacity to not only generate ideas but also refine them through data-driven insights.
The process behind Robin’s success is as innovative as its outcomes. When given a disease name, Robin begins by formulating general questions about pathology, deploying Crow to provide detailed answers. It then identifies potential causal mechanisms, ranks them using an LLM judge for pairwise comparisons, and selects the top in vitro model to guide experimental strategy. Once experiments are conducted, scientists upload raw data, and Finch performs analyses across multiple trajectories to account for the inherent ambiguity in biological data interpretation. For instance, choices in gating for flow cytometry or filters in RNA-seq can vary, so Robin runs ten independent Finch analyses, synthesizing results into a consensus conclusion. This approach balances diversity in analysis with consistency in findings, ensuring robust scientific insights. Finally, Robin distills these results into actionable recommendations and proposes follow-up assays, perpetuating a cycle of discovery until a satisfactory therapeutic candidate emerges.
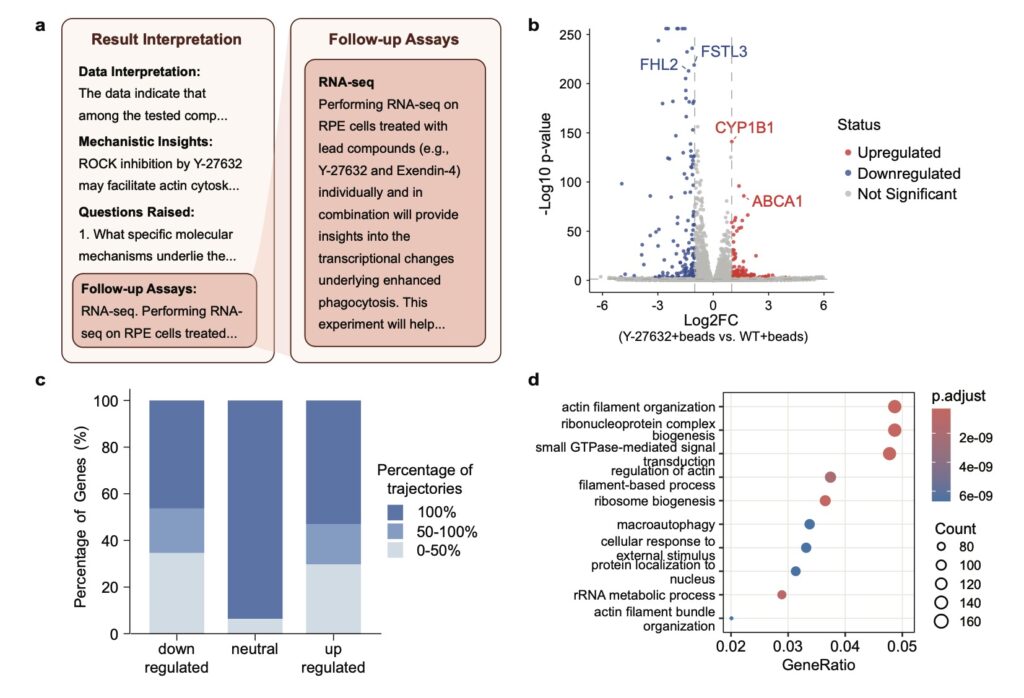
Beyond its specific triumph with dAMD, Robin’s implications for biomedical research are vast. Drug development, a field constrained by the slow synthesis of biological, clinical, and pharmaceutical expertise, stands to benefit immensely from such automation. With FDA approvals for novel drugs stagnating at around 50 per year over the past decade, the need for scalable discovery platforms is urgent. Robin’s ability to automate hypothesis generation grounded in literature and pair it with experimental data analysis positions it as a game-changer. Its broader applicability is evident in its generation of hypotheses for ten additional diseases with pressing therapeutic needs, showcasing a versatility that could transform how we approach drug discovery across diverse medical challenges.
Robin is not without room for growth. In its current form, it generates experimental outlines but lacks the precision to produce executable lab protocols, an area targeted for future development to minimize human intervention. The Finch data analysis agent also relies heavily on expert prompt engineering, suggesting a need for greater autonomy in adapting to specific data types. Additionally, while Robin’s LLM-judged tournament for hypothesis ranking is innovative, aligning its evaluations more closely with human scientific judgment could enhance reliability. These opportunities for refinement underscore that Robin, while groundbreaking, is still an evolving tool—one with the potential to become even more integral to scientific progress.
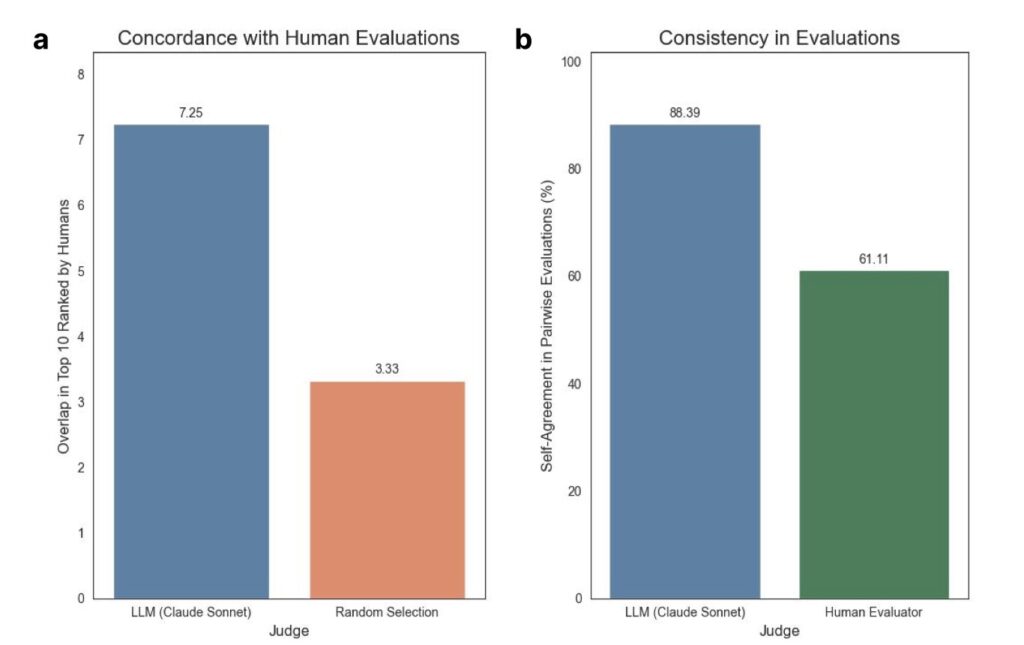
Robin represents a paradigm shift in AI-driven scientific discovery, offering the first fully integrated system to automate the intellectual steps of research. Its success in identifying ripasudil as a novel treatment for dAMD is a testament to its ability to synthesize existing knowledge into innovative solutions, while its scalable framework promises to accelerate therapeutic development across a spectrum of diseases. As Robin continues to evolve, addressing current limitations and enhancing its autonomy, it stands poised to redefine the pace and possibilities of science. In a world where the complexity of biological data grows daily, Robin offers a glimpse into a future where AI not only assists but actively drives the quest for knowledge and healing.
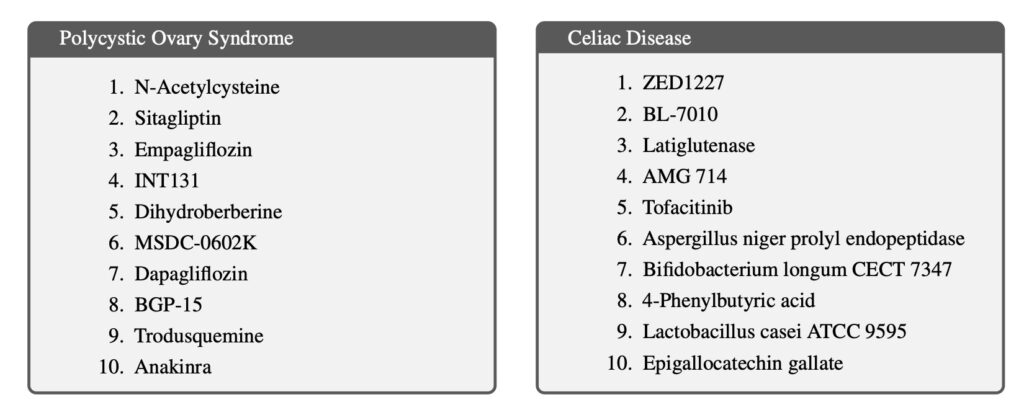