Enhancing Pretrained ControlNets for Seamless Integration with Diffusion Models
- Efficiency and Versatility: CTRL-Adapter enhances existing ControlNets to work with any diffusion model without the need for retraining, significantly reducing computational costs.
- Temporal Consistency: The framework includes specialized modules that ensure the temporal alignment of features across video frames, crucial for maintaining the continuity of generated content.
- Multi-Condition Control: Supports versatile scenario applications by managing multiple control conditions simultaneously, providing a robust solution for complex image and video generation tasks.
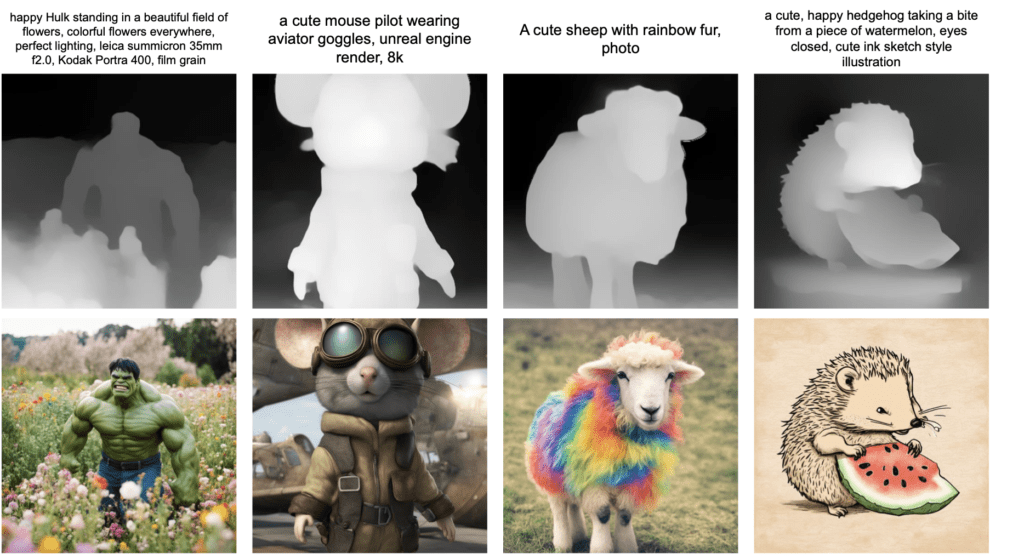
The recent surge in using generative models for creating dynamic and controlled visual content has posed new challenges, particularly in the seamless integration of control mechanisms with advanced diffusion models. To address these, the innovative CTRL-Adapter framework emerges as a transformative solution, designed to adapt existing ControlNets for use with any image or video diffusion models effectively.
At its core, CTRL-Adapter integrates adapter layers that bridge the feature space between pretrained ControlNets and new diffusion models, allowing for a smooth transfer of control capabilities without altering the underlying parameters of either system. This approach not only preserves the integrity of the original models but also offers a cost-effective alternative to developing new ControlNets from scratch.
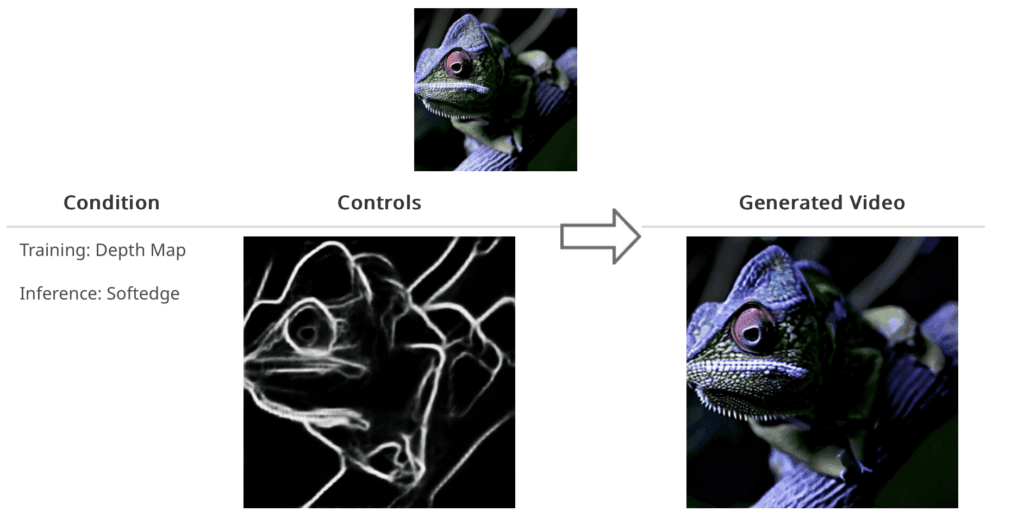
One of the standout features of CTRL-Adapter is its ability to manage temporal consistency within video sequences. Through a combination of temporal and spatial modules, the framework ensures that objects remain consistent across frames, addressing a common issue in controlled video generation where the temporal alignment of objects can often be disrupted.
Moreover, CTRL-Adapter’s versatility extends to handling multiple control conditions simultaneously. Whether it’s depth maps, canny edges, or human poses, the framework can integrate various data types to enrich the generative process. This multi-condition capability is particularly beneficial for complex scenarios where different types of control inputs are necessary to achieve desired outcomes.
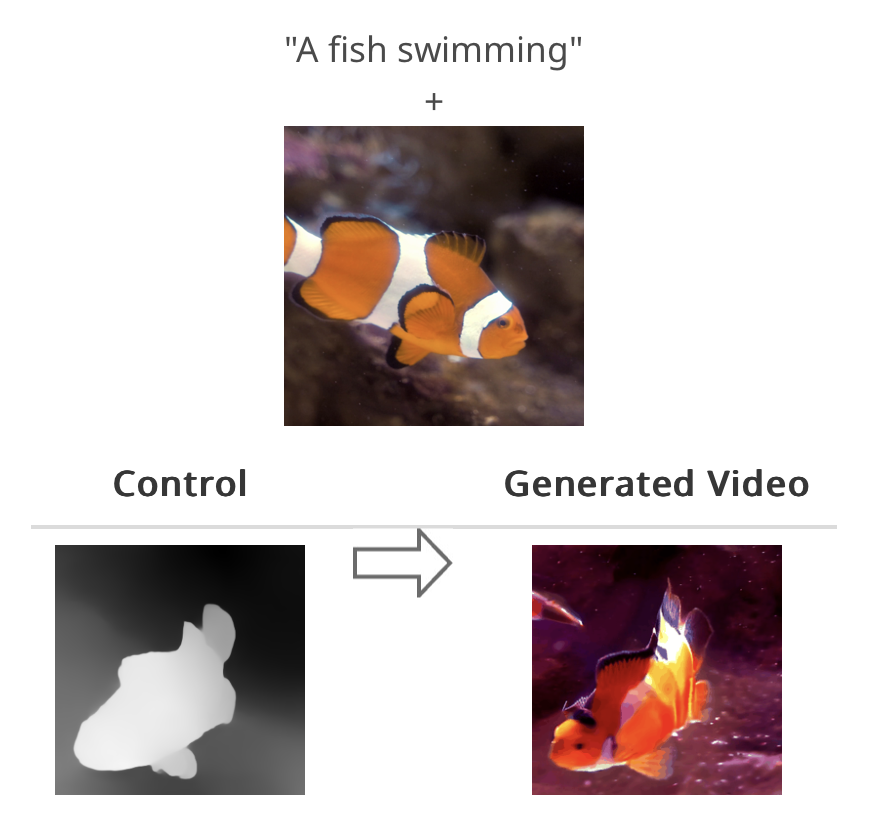
In practice, CTRL-Adapter has demonstrated its prowess by matching, and in some cases surpassing, the performance of ControlNets on rigorous benchmarks like the COCO dataset for image control and the DAVIS 2017 dataset for video control. These achievements highlight the framework’s capacity to deliver high-quality controlled media with reduced computational demands.
The development of CTRL-Adapter marks a significant advancement in the field of generative media, offering a scalable, efficient solution for creators and technologists looking to explore the boundaries of image and video generation. As this technology continues to evolve, it promises to unlock new creative potentials and applications, further enhancing the capability of generative AI in media production.