Refining Visual Processing in Large Language Models
- Enhanced Resolution Handling: Ferret-v2 introduces ‘any resolution grounding and referring,’ allowing for superior processing of high-resolution images, significantly enhancing detail recognition and understanding.
- Multi-Granularity Visual Encoding: Integration of the DINOv2 encoder enables Ferret-v2 to capture a broader spectrum of visual information, from global scenes to intricate details, enhancing the model’s contextual awareness.
- Innovative Three-Stage Training Paradigm: The new training approach includes stages for image-caption alignment and high-resolution dense alignment, culminating in final instruction tuning to refine the model’s performance further.
Apple’s latest iteration of its language model, Ferret-v2, marks a significant advancement in the field of machine learning, particularly in how large language models integrate and interpret visual data. Building on the foundation set by the original Ferret model, Ferret-v2 addresses and overcomes previous limitations with several key enhancements that promise to revolutionize the model’s utility and effectiveness.
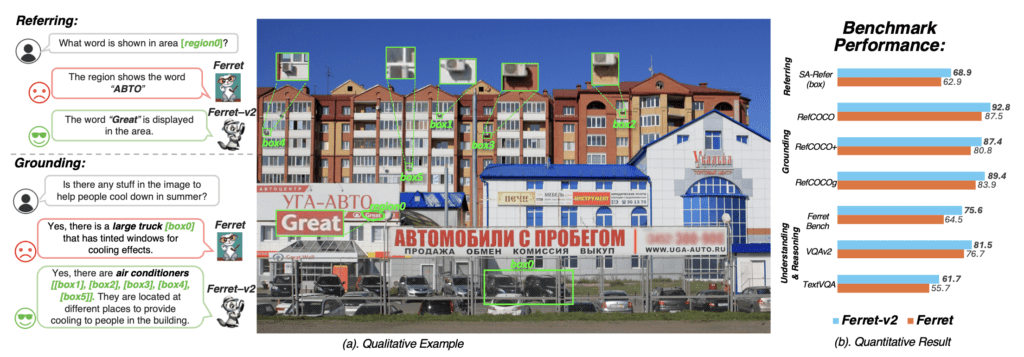
Core Innovations
Resolution Flexibility: One of the standout features of Ferret-v2 is its ability to handle images of any resolution. This flexibility is crucial as it allows the model to maintain high performance regardless of the image detail level, making it particularly useful in fields where precision in visual data is paramount, such as medical imaging or detailed geographic analysis.
Advanced Visual Encoding: By incorporating the DINOv2 encoder, Ferret-v2 can process visual information at multiple granularities. This capability allows it to understand both the overall context of an image and its finer details, providing a more nuanced interpretation of visual data. This multi-layered approach to visual encoding significantly broadens the model’s applicability across various domains where different scales of visual interpretation are required.
Three-Stage Training Paradigm: Ferret-v2’s training regimen is another area where significant innovations have been made. The model undergoes a three-stage training process, starting with basic image-caption alignment, advancing through high-resolution dense alignment, and finishing with specific instruction tuning. This structured approach ensures that the model not only aligns well with the visual content but also adheres closely to the instructions provided, improving both accuracy and relevancy of the output.
Implications and Potential Uses
The enhancements in Ferret-v2 are not just technical upgrades; they represent a shift towards more capable and versatile AI tools that can be tailored to a wide range of applications. From creative industries that require detailed visual understanding to scientific fields where precision is critical, Ferret-v2’s capabilities make it a valuable tool across many sectors.
Challenges and Ethical Considerations
Despite its advancements, Ferret-v2, like most machine learning models, faces challenges, particularly in generating responses that could be harmful or counterfactual. Apple acknowledges these potential issues and suggests that ongoing improvements and ethical considerations will be key components of Ferret-v2’s future development.
Ferret-v2 is a testament to Apple’s commitment to pushing the boundaries of what large language models can achieve in visual processing. By addressing previous limitations and introducing forward-thinking innovations, Ferret-v2 stands poised to set new standards in the integration of visual data within language models, offering more powerful and precise tools for interpreting complex visual information.