A New Benchmark for Assessing AI Agents’ Performance in Real-World ML Tasks
OpenAI has unveiled MLE-Bench, a groundbreaking benchmark designed to evaluate the performance of AI agents in machine learning engineering tasks.
- Diverse Challenges: MLE-Bench curates 75 Kaggle competitions, creating a comprehensive set of tasks that mimic real-world machine learning engineering scenarios, such as model training and dataset preparation.
- Human Baselines Established: The benchmark establishes human performance baselines using publicly available Kaggle leaderboards, allowing for direct comparisons between AI agents and human competitors.
- Promising Results: The combination of OpenAI’s o1-preview model with AIDE scaffolding shows that AI agents can achieve performance levels comparable to a Kaggle bronze medal in 16.9% of competitions.
In the rapidly evolving field of artificial intelligence, accurately assessing the capabilities of AI agents in machine learning (ML) engineering is crucial. To address this need, OpenAI has introduced MLE-Bench, a novel benchmark that provides a structured framework for evaluating AI performance across various ML engineering tasks. By leveraging the rich data from Kaggle competitions, MLE-Bench simulates real-world scenarios and offers a comprehensive tool for measuring the effectiveness of AI in executing complex engineering tasks.
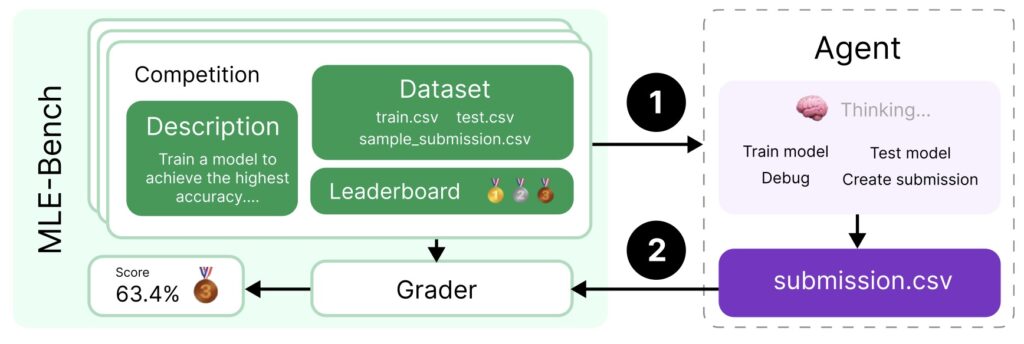
The selection of 75 Kaggle competitions as the foundation for MLE-Bench ensures that the benchmark covers a diverse range of challenges. These competitions include tasks such as training models, preparing datasets, and running experiments, all of which are essential skills for successful ML engineering. By mimicking the competitive environment of Kaggle, MLE-Bench provides a realistic context for evaluating AI agents and allows for direct comparisons with human competitors who have participated in these competitions.

One of the notable features of MLE-Bench is the establishment of human baselines. By using publicly available leaderboards from Kaggle, the benchmark enables researchers to measure AI performance against human standards, providing a clearer picture of how well AI agents can execute tasks traditionally performed by skilled ML engineers. This approach not only facilitates meaningful comparisons but also helps identify areas where AI agents can improve their capabilities.
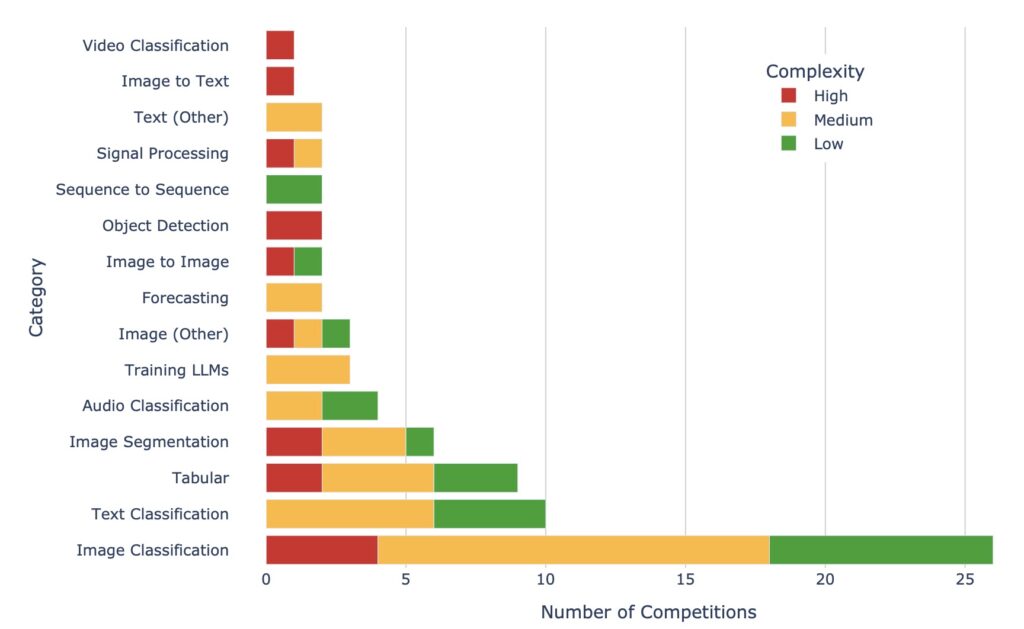
Preliminary results from experiments conducted using MLE-Bench are promising. The best-performing setup, which combines OpenAI’s o1-preview model with AIDE scaffolding, achieved at least a bronze medal level in 16.9% of the competitions evaluated. This achievement highlights the potential of advanced AI models to perform competitively in ML engineering tasks, marking a significant step forward in the quest for more capable autonomous systems.
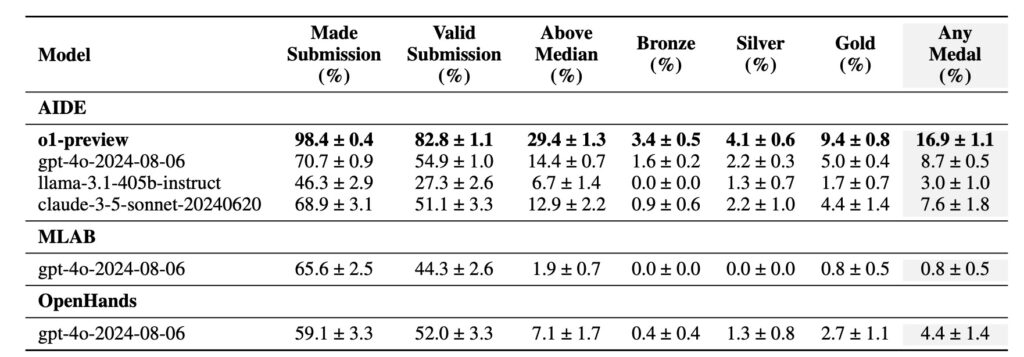
By open-sourcing MLE-Bench, OpenAI aims to foster further research into the evaluation of AI agents’ ML engineering capabilities. As the deployment of more powerful AI models becomes increasingly common, understanding their abilities to autonomously execute ML engineering tasks is essential for ensuring their safe and effective use. MLE-Bench serves as a valuable resource for researchers and developers alike, paving the way for advancements in the field of AI and machine learning.
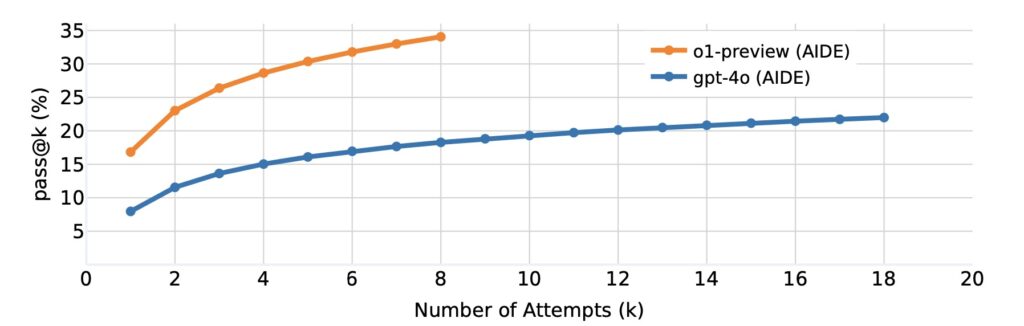
MLE-Bench represents a significant advancement in the evaluation of AI agents within the realm of machine learning engineering. By establishing a structured benchmark based on real-world competitions and enabling comparisons with human performance, OpenAI has created a vital tool that will facilitate research and innovation in the development of more capable AI systems. As the field continues to evolve, MLE-Bench will play a crucial role in understanding and enhancing the engineering capabilities of AI agents, ultimately contributing to the responsible deployment of these technologies.
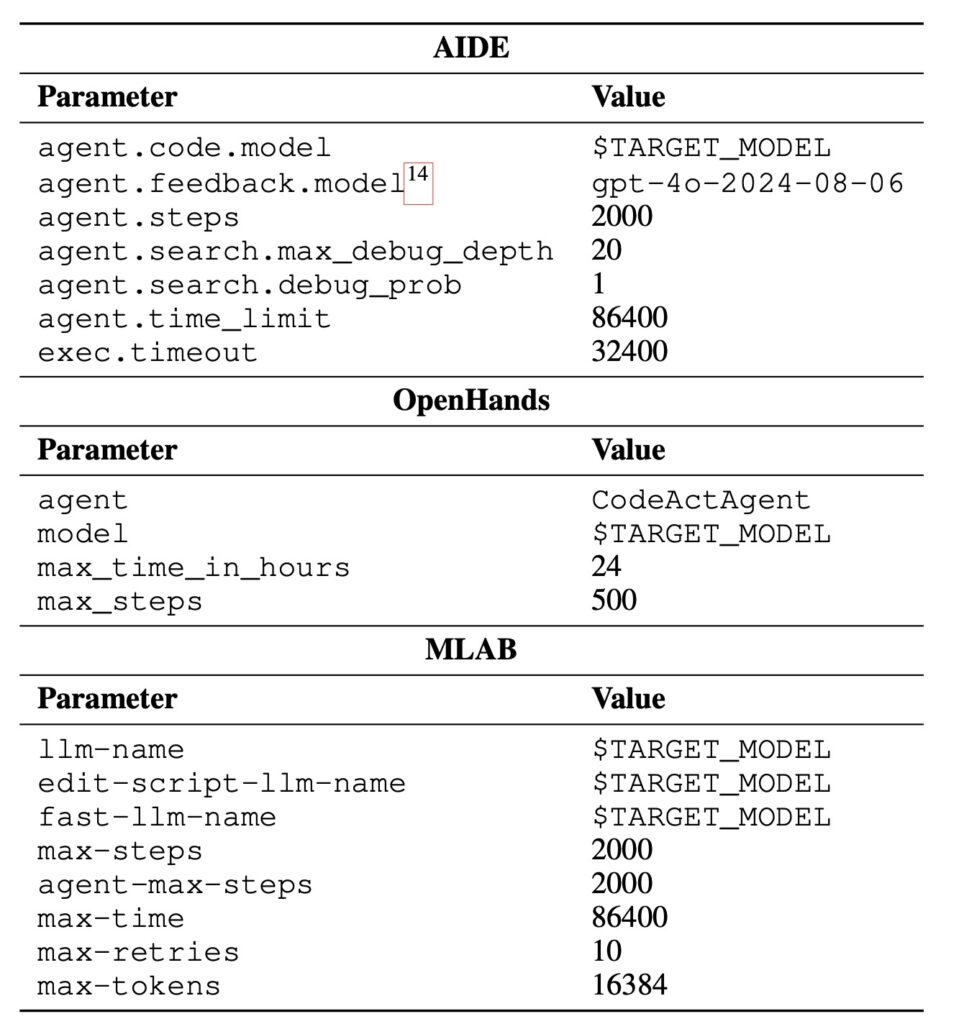