Human-in-the-Loop Reinforcement Learning Revolutionizes Robotic Skills Acquisition
The quest for precise and dexterous robotic manipulation has reached new heights with the introduction of a human-in-the-loop reinforcement learning (RL) system. This innovative approach not only enhances the capabilities of robots but also transforms how they learn complex tasks.
- Enhanced Learning Efficiency: The human-in-the-loop RL system enables robots to achieve near-perfect success rates and rapid cycle times in just 1 to 2.5 hours of training across various dexterous tasks, significantly outperforming traditional methods.
- Broad Applicability: This framework is poised to revolutionize industries that require high-mix low-volume production, such as electronics and automotive manufacturing, by allowing robots to adapt to varied tasks efficiently.
- Future Opportunities: The method lays the groundwork for generating high-quality data for training robot foundation models and reducing training times through value function pretraining, paving the way for broader applications in robotic manipulation.
The challenges associated with teaching robots complex manipulation skills have long hindered advancements in automation. Traditional reinforcement learning approaches often struggle to learn effectively in real-world settings, where unpredictability and task complexity prevail. The newly introduced human-in-the-loop RL system addresses these issues head-on by integrating human demonstrations and corrections into the training process.
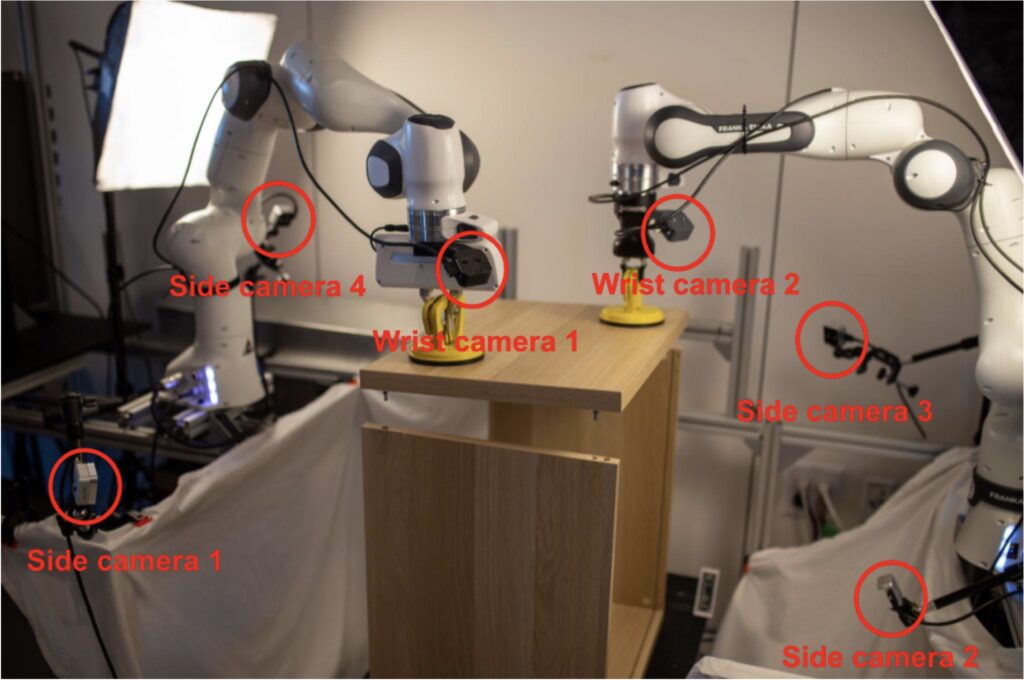
By allowing human operators to guide robots through challenging tasks, this approach accelerates learning and significantly enhances the robot’s ability to adapt to dynamic environments. The results demonstrate that with the right design choices, RL can effectively acquire complex manipulation skills in a fraction of the time previously required.
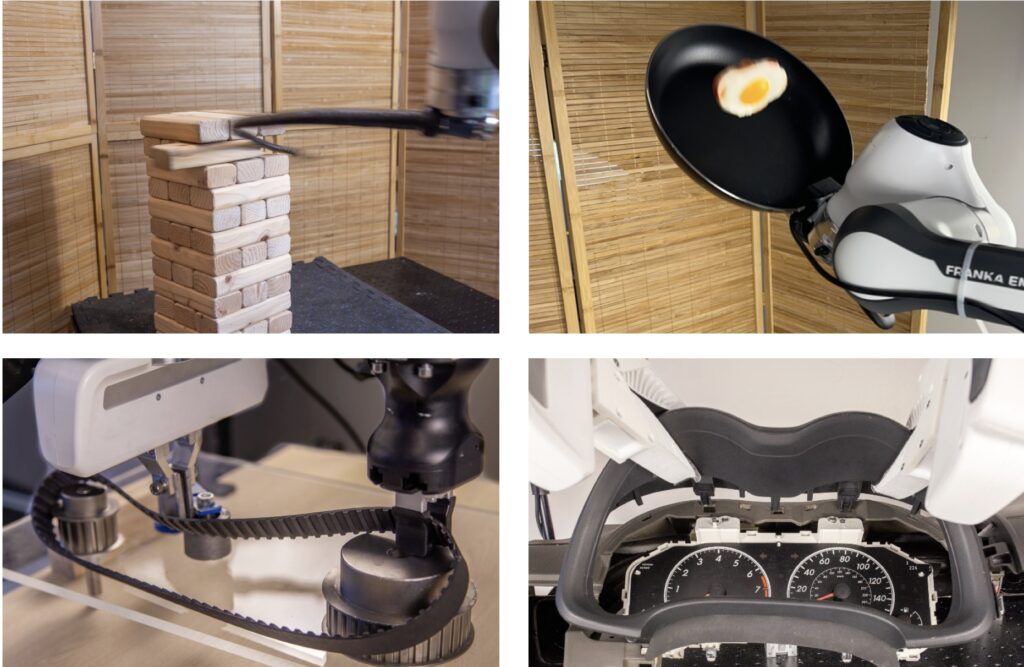
Achieving Dexterity through Collaboration
The core innovation of this system lies in its dual-agent framework, which consists of human input and AI-driven algorithms. The human operator can provide demonstrations and corrections, which are then incorporated into the learning process. This collaborative approach fosters a more robust understanding of tasks, allowing the robot to engage in dynamic manipulation, precision assembly, and dual-arm coordination.
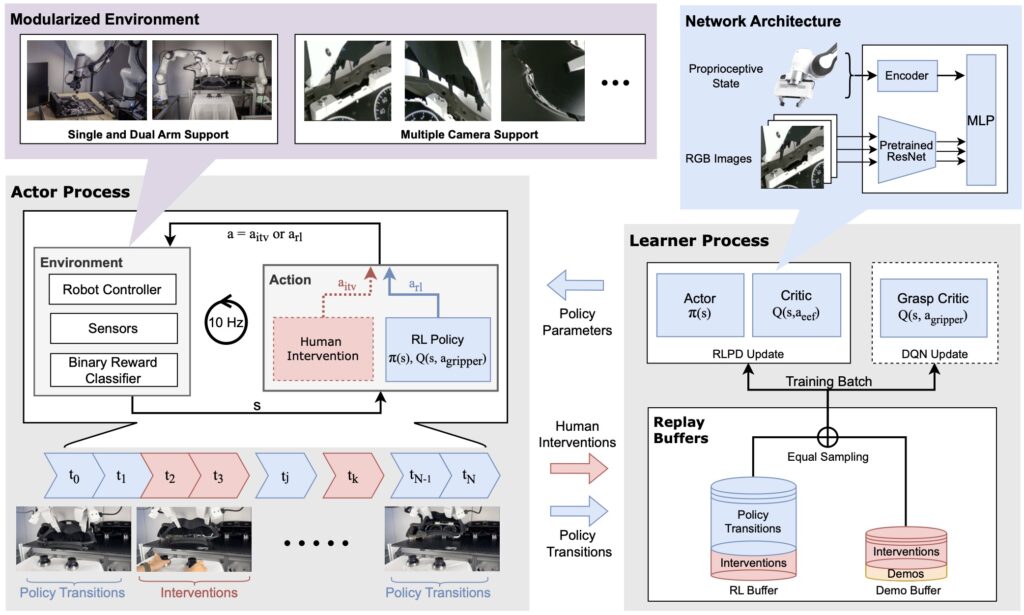
Through extensive experimentation, the system has shown an impressive average 2x improvement in success rates and 1.8x faster execution compared to traditional imitation learning baselines. The ability to create adaptive policies for both reactive and predictive control strategies ensures that robots can handle a wide range of manipulation challenges effectively.
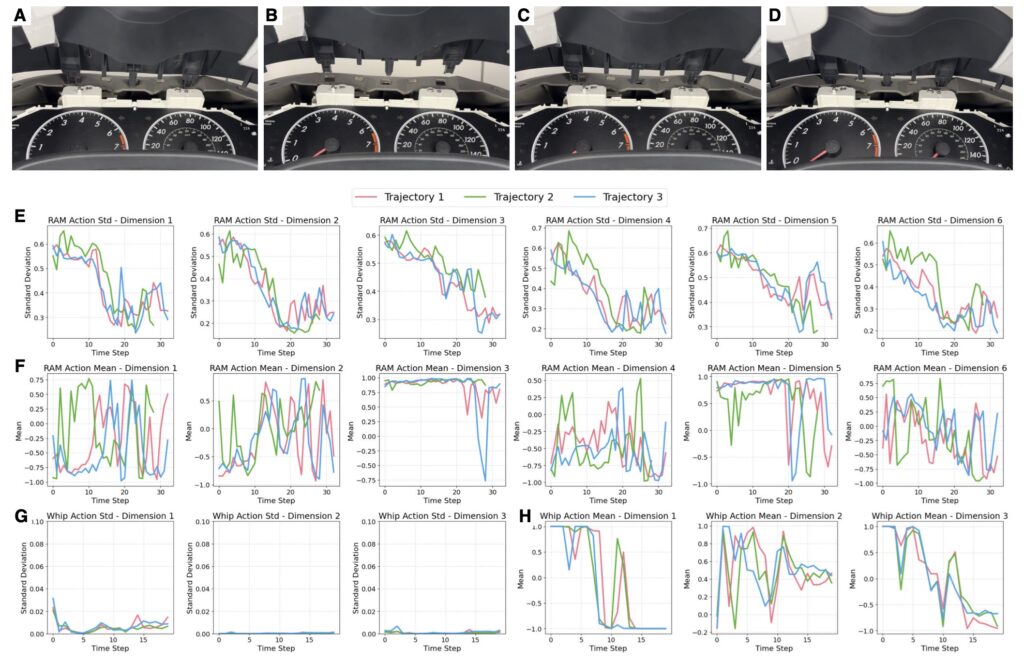
Real-World Applications and Industry Impact
The implications of this research extend beyond academic interest; they have the potential to reshape industries that rely on automated production. High-mix low-volume manufacturing environments, which are increasingly common in sectors such as electronics, semiconductors, and automotive production, demand agility and flexibility. Co-STORM can adapt to various tasks, providing customized solutions that can enhance productivity and reduce costs.
By implementing this technology, companies can expect shorter product life cycles and increased customization options, enabling them to respond more effectively to market demands. As the framework proves its efficacy in real-world applications, it is likely to be adopted widely, driving advancements in industrial automation.
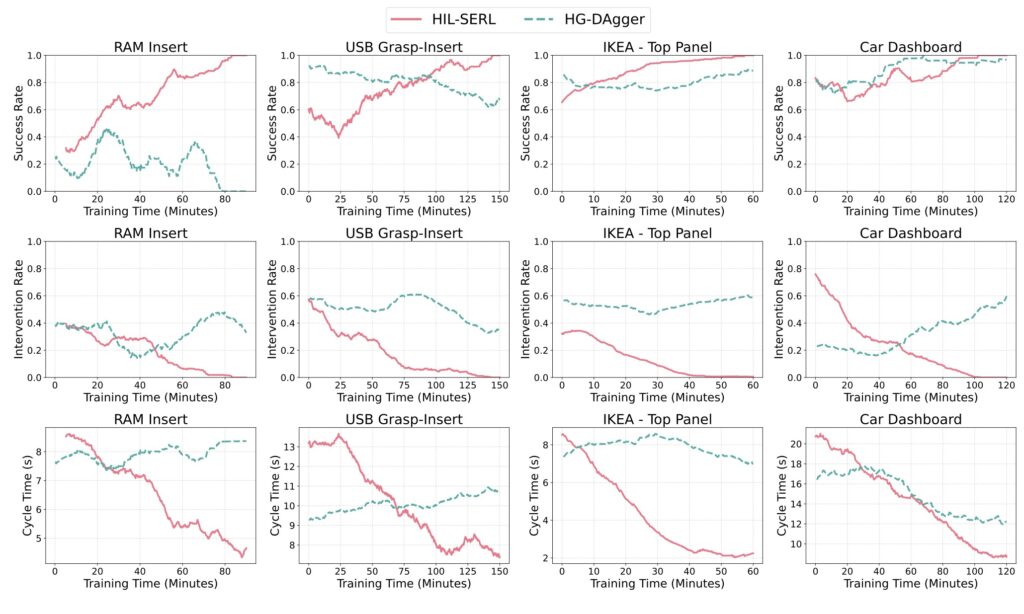
Exploring Future Possibilities
While the current system has demonstrated remarkable success, there are opportunities for further refinement and expansion. One potential avenue is the pretraining of a value function that encapsulates general manipulation capabilities, allowing for quicker fine-tuning to specific tasks. Additionally, there is scope for generating high-quality training data through the execution of converged policies, which can be distilled into generalist models.
Addressing the limitations of the current approach is also crucial. While the system excels at various tasks, the effectiveness in longer-horizon tasks remains uncertain. Future work could explore strategies for segmenting long-horizon tasks into manageable sub-tasks, potentially aided by vision-language models.
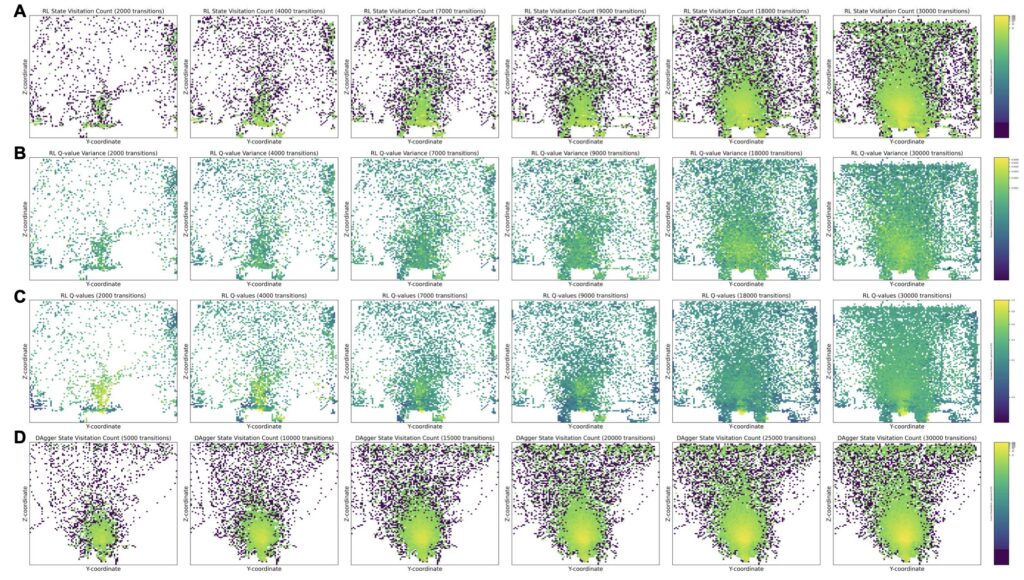
A Promising Future for Robotics
The introduction of the human-in-the-loop reinforcement learning system represents a significant advancement in robotic manipulation, combining human insight with machine learning capabilities. With its ability to learn complex skills efficiently and adapt to dynamic environments, this approach opens new avenues for industrial applications and research advancements.
As the field of robotics continues to evolve, integrating human expertise will play a vital role in overcoming challenges associated with automation. The promising results of this research not only demonstrate the feasibility of effective RL in real-world settings but also lay the foundation for a future where robots can seamlessly integrate into diverse industries, enhancing productivity and innovation. The journey toward more intelligent and capable robotic systems is just beginning, and with initiatives like this, the possibilities are endless.
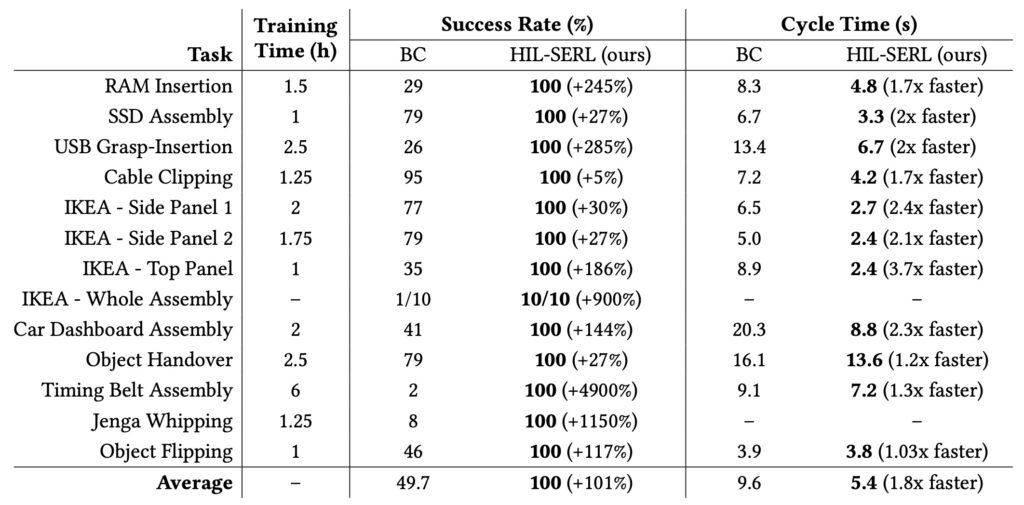