New Simulation Platform Enhances Robot Training and Performance in Diverse Real-World Scenario
- Advanced Simulation for Robots: GRUtopia offers a simulated 3D society with diverse, interactive environments for training various robots.
- Comprehensive Data and NPC Integration: Features a vast dataset (GRScenes) and NPC system (GRResidents) for realistic task simulation.
- Benchmarking and Evaluation: Introduces GRBench to assess robots’ capabilities in navigation, social interaction, and manipulation.
The realm of Embodied AI is set to experience a paradigm shift with the introduction of GRUtopia, a groundbreaking project designed to enhance the training and performance of robots in a simulated city-scale environment. Developed to overcome the limitations of existing platforms, GRUtopia promises to revolutionize how robots are trained, evaluated, and ultimately deployed in real-world scenarios.
Advanced Simulation for Robots
GRUtopia is poised to address the critical need for scalable and realistic training environments for robots. Traditional approaches to Embodied AI have been hampered by the high costs and logistical challenges associated with collecting real-world data. GRUtopia leverages the Simulation-to-Real (Sim2Real) paradigm, offering a comprehensive solution through its large-scale, interactive 3D environments. These environments, known as GRScenes, include 100,000 finely annotated scenes covering a wide range of settings from supermarkets and hospitals to amusement parks and libraries. This diversity allows robots to be trained for a multitude of service-oriented tasks beyond the confines of home environments.
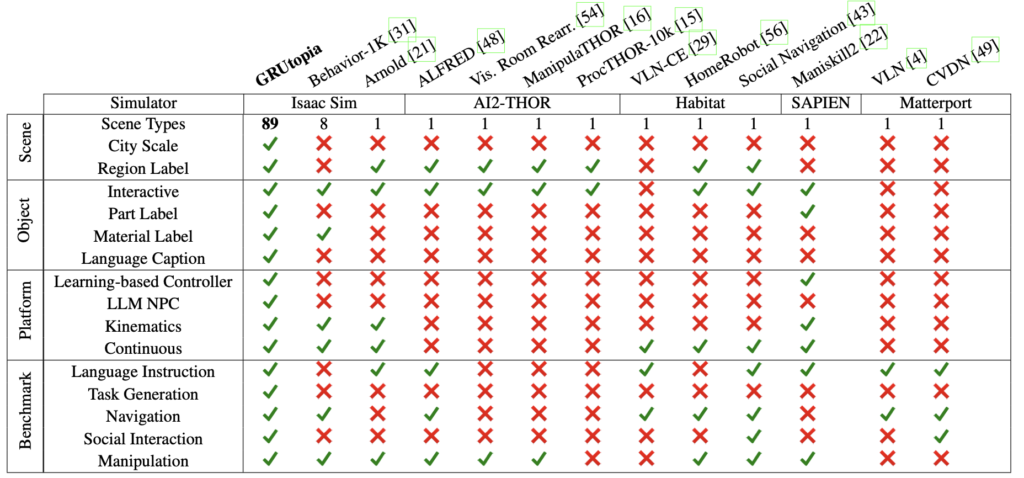
Comprehensive Data and NPC Integration
A standout feature of GRUtopia is its integration of Non-Player Characters (NPCs) driven by Large Language Models (LLMs). The NPC system, named GRResidents, facilitates social interactions, task generation, and task assignment, providing a realistic social context for training robots. This enables the simulation of complex social scenarios that robots are likely to encounter in real-world applications. By leveraging robust DINO features, GRUtopia ensures that the interactions are both dynamic and contextually relevant.
Benchmarking and Evaluation
To measure the effectiveness of robot training, GRUtopia introduces GRBench, a benchmark specifically designed for evaluating robots’ performance across various tasks. GRBench includes three primary benchmarks: Object Loco-Navigation, Social Loco-Navigation, and Loco-Manipulation. These benchmarks are moderately challenging, reflecting the current capabilities of state-of-the-art algorithms, and provide a robust framework for assessing robots’ abilities in navigation, social interaction, and manipulation.
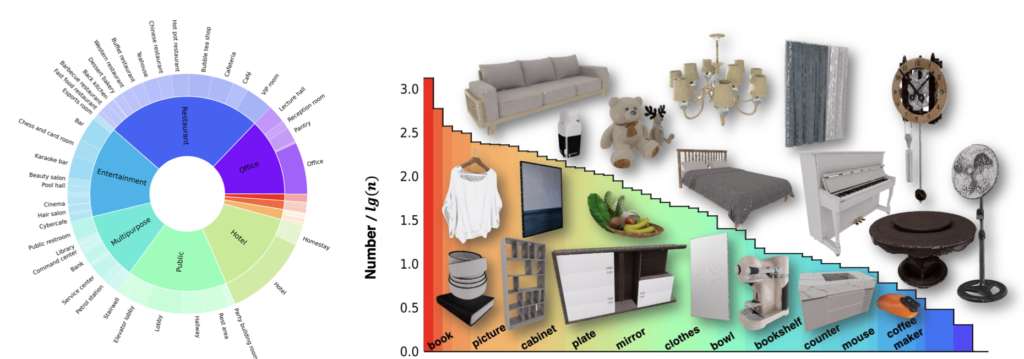
Future Directions and Enhancements
GRUtopia is an ongoing project with continuous enhancements planned. The initial version includes 100 annotated indoor scenes and a city block, with future updates set to expand the 3D scene assets, control policies, task generation mechanisms, and NPC systems. The aim is to create a comprehensive platform that not only simulates realistic environments but also facilitates the scaling up of embodied learning for diverse robotic applications.
Ideas for Further Exploration
- Integration with Real-World Data: Exploring ways to incorporate real-world data into the simulation to enhance the realism and applicability of the training environments.
- Advanced Interaction Models: Developing more sophisticated NPC interactions that include physically realistic contact and more complex social dynamics.
- Customizable Training Scenarios: Allowing users to create and customize training scenarios specific to their needs, enabling targeted skill development for robots.
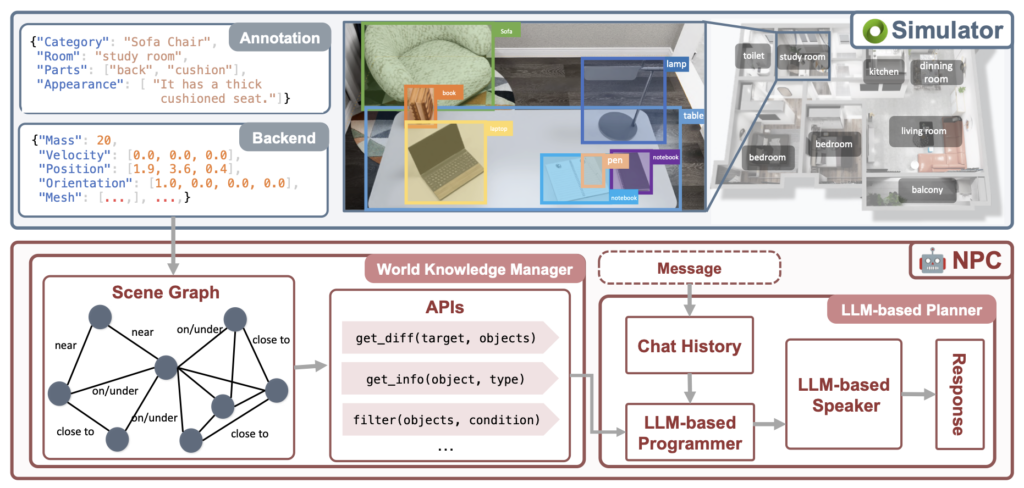
GRUtopia represents a significant advancement in the field of Embodied AI, providing a versatile and scalable platform for training and evaluating robots in diverse, realistic environments. By combining extensive data sets, advanced NPC systems, and comprehensive benchmarks, GRUtopia is set to accelerate the development of highly capable robots ready to perform complex tasks in real-world settings. This innovative approach not only enhances the training process but also opens up new possibilities for the practical deployment of AI-driven robots in various industries.