Researchers investigate the vulnerability of AI-generated text detectors and call for a more honest conversation about the ethical use of large language models.
The rapid development of large language models (LLMs) has led to impressive advancements in natural language processing tasks, such as document completion and question answering. However, the unregulated use of LLMs could potentially lead to harmful consequences, including plagiarism, fake news generation, and spamming. Consequently, there is a growing need for reliable AI-generated text detection methods to ensure the responsible use of LLMs.
In a recent paper, researchers Vinu Sankar Sadasivan, Aounon Kumar, Sriram Balasubramanian, Wenxiao Wang, and Soheil Feizi analyzed the reliability of current AI-generated text detectors. They demonstrated both empirically and theoretically that these detectors are not reliable in practical scenarios. The researchers found that paraphrasing attacks can effectively evade a range of detectors, including watermarking schemes, neural network-based detectors, and zero-shot classifiers.
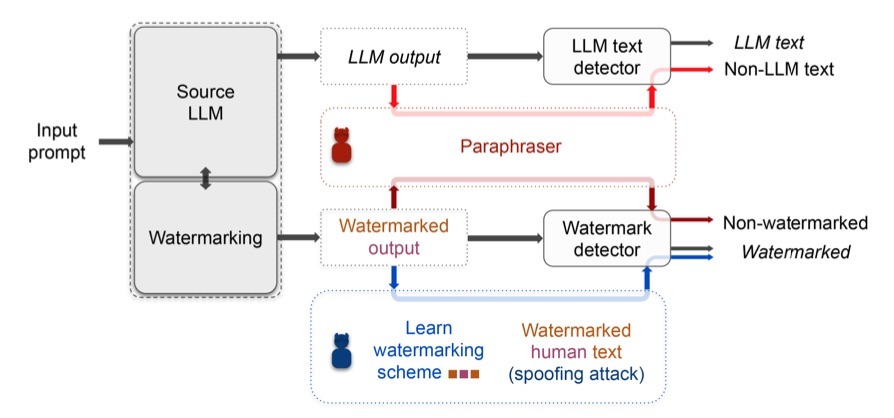
The study also provides a theoretical impossibility result indicating that for a sufficiently good language model, even the best possible detector can only perform marginally better than a random classifier. Additionally, the researchers showed that LLMs protected by watermarking schemes can be vulnerable to spoofing attacks, where adversarial humans can infer hidden watermarking signatures and add them to their generated text, potentially causing reputational damage to LLM developers.
These findings highlight the need for more robust detection methods to prevent the misuse of LLMs. The researchers suggest that future research on AI text detectors should carefully consider potential vulnerabilities and design more secure methods to counter emerging threats.
The authors of the paper hope that their results will encourage an open and honest discussion within the AI community about the ethical and trustworthy applications of generative LLMs. As AI-generated text becomes increasingly prevalent, it is crucial to strike a balance between leveraging LLMs’ capabilities and mitigating their potential risks.