A Breakthrough Framework for Realistic and Controllable 3D Motion Synthesis
- Moving Beyond 2D Limitations:Â 3DTrajMaster introduces 3D motion control for multi-entity dynamics, surpassing traditional 2D control techniques.
- Innovative Techniques:Â A plug-and-play 3D-motion injector and domain adaptation strategies ensure high-quality, generalizable video synthesis.
- A Versatile Dataset: The 360°-Motion Dataset, featuring diverse 3D trajectories, sets a new standard for training and evaluation in this field.
Traditional video generation methods have relied heavily on 2D control signals such as sketches and bounding boxes. While effective, these approaches struggle to capture the complexities of 3D motion, which is crucial for realistic video synthesis. Recognizing this limitation, researchers have developed 3DTrajMaster, a groundbreaking framework designed to regulate multi-entity motions in 3D space with unparalleled precision.
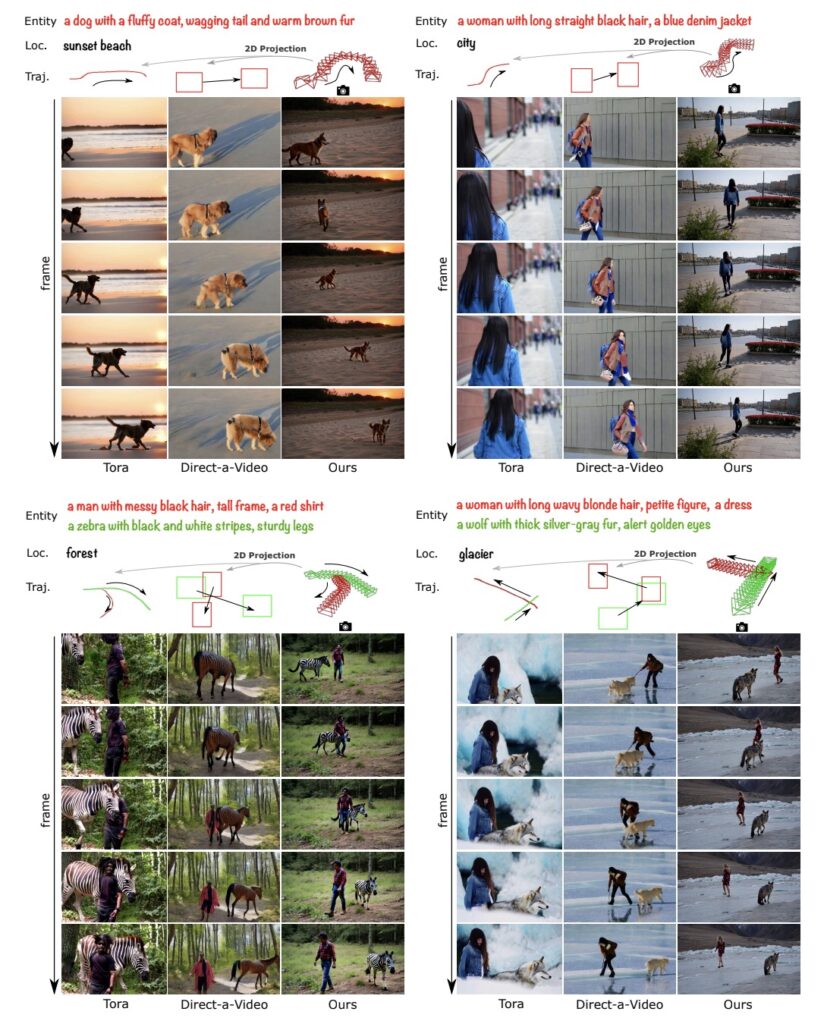
Introducing 3DTrajMaster
At the heart of 3DTrajMaster lies a robust 3D-motion grounded object injector. This innovative component aligns input entities with their respective 3D trajectories using a gated self-attention mechanism. By preserving the video diffusion prior, the injector ensures high-quality outputs while maintaining the generalization ability critical for diverse applications. Additionally, a domain adaptor mitigates video quality degradation during training, while an annealed sampling strategy enhances inference performance.
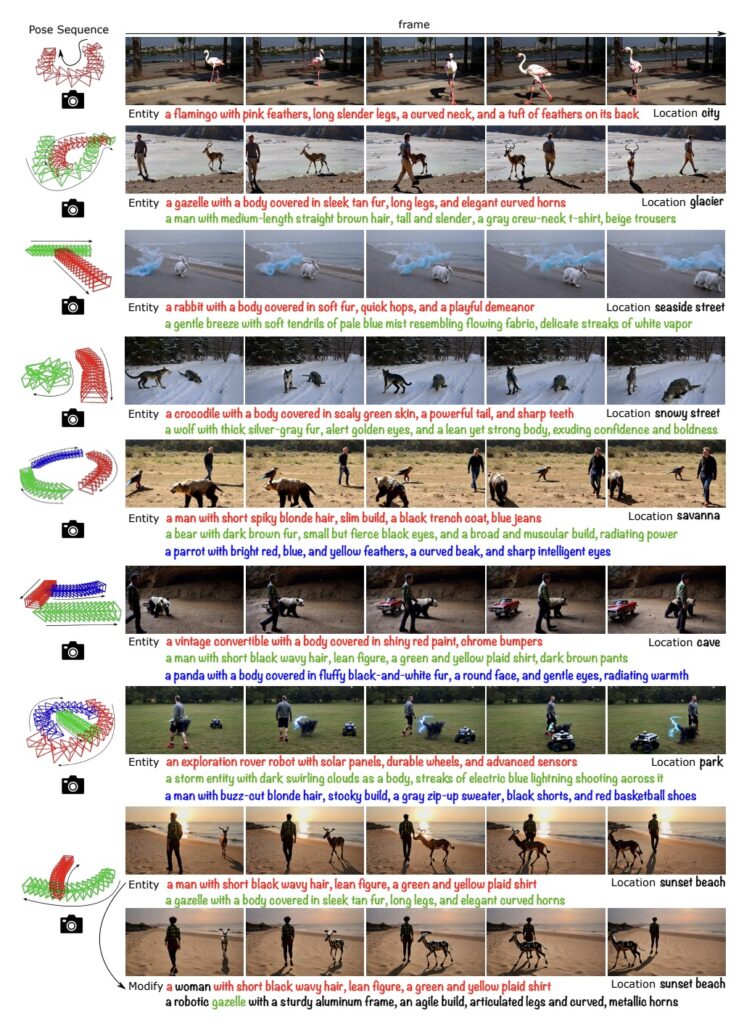
The Role of the 360°-Motion Dataset
A key enabler of 3DTrajMaster’s success is the 360°-Motion Dataset. This comprehensive dataset combines 3D human and animal assets with GPT-generated trajectories, captured using a 12-camera setup on Unreal Engine platforms. The dataset’s diversity and richness provide a solid foundation for training models capable of controlling multi-entity motions with accuracy and realism.
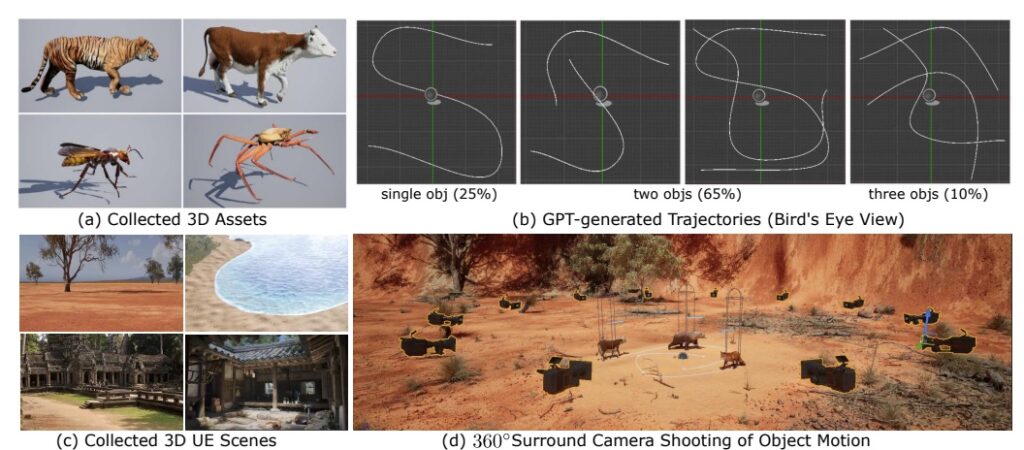
Applications and Future Directions
3DTrajMaster opens the door to numerous applications, from virtual cinematography in the film industry to interactive gaming and embodied AI systems. However, the current model has limitations, including restricted granularity in editing animal entities and generating complex local motions. Expanding the dataset and incorporating more powerful video foundation models could address these challenges, enabling finer control over intricate motions and interactions.
Extensive experiments demonstrate that 3DTrajMaster sets a new benchmark for accuracy and generalization in multi-entity 3D motion control. By bridging the gap between 2D control limitations and real-world 3D dynamics, this framework represents a significant step forward in video generation. As the technology evolves, 3DTrajMaster has the potential to transform the way we create and interact with dynamic, lifelike videos.
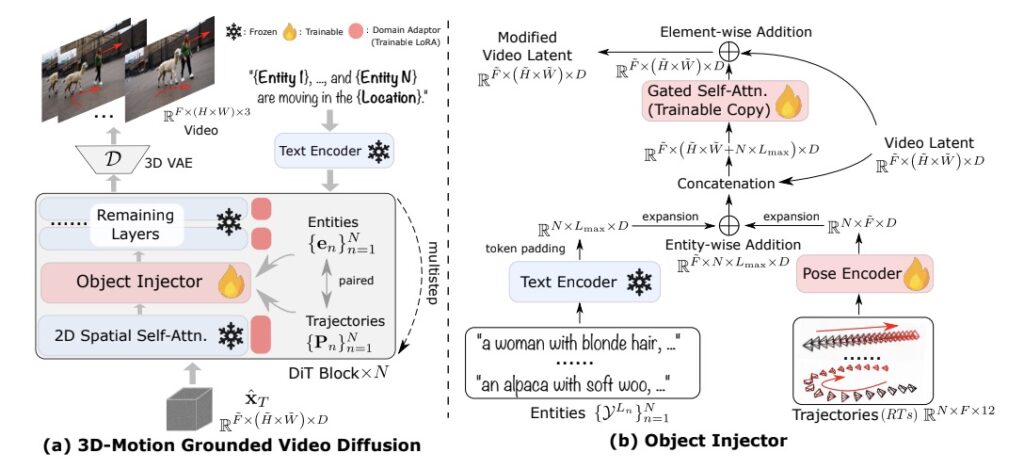