Harnessing 2D Autoregressive Techniques for Enhanced Vision-Language Intelligence
- Innovative Architecture: The DnD Transformer addresses the information loss issues associated with vector-quantization (VQ) autoregressive image generation by introducing new autoregression directions and enhancing model depth.
- Enhanced Image Quality: Compared to traditional 1D autoregressive models, the DnD Transformer can produce higher-quality images without increasing the model size or sequence length, showcasing its efficiency.
- Emerging Vision-Language Intelligence: This new model demonstrates the ability to generate images with rich text and graphics in a self-supervised manner, hinting at the model’s understanding of combined visual and textual modalities.
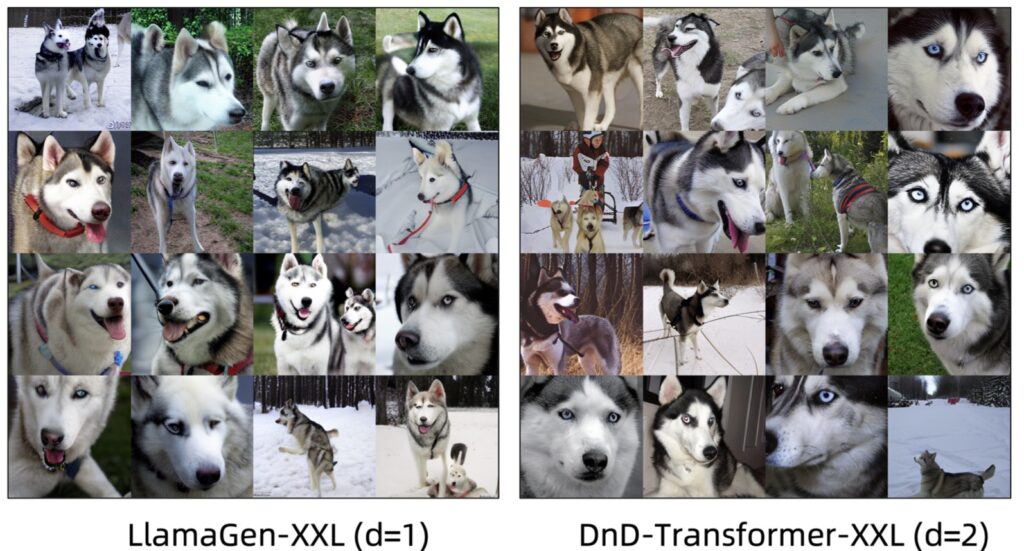
The landscape of autoregressive (AR) image generation is undergoing a significant transformation, propelled by advancements in large language models (LLMs) and innovative techniques. At the forefront of this evolution is the newly introduced DnD Transformer, which redefines the traditional methods of image generation by tackling longstanding issues related to information loss and computational efficiency. By introducing a 2D autoregressive framework, this groundbreaking model offers a fresh perspective on optimizing the image generation process.
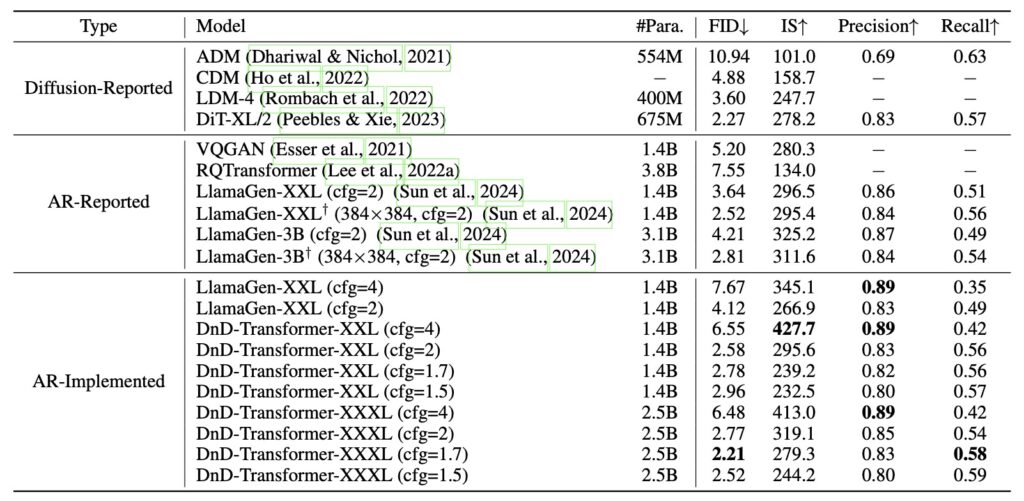
One of the primary challenges faced by previous AR image generation models has been the limitations of vector quantization (VQ). While VQ has paved the way for successful models like DALL·E and VQGAN, it has also introduced challenges in balancing reconstruction fidelity with prediction complexity. The DnD Transformer mitigates these limitations by leveraging both depth dimension autoregression and spatial dimension processing, significantly enhancing the quality of generated images without the need for increased model size or sequence length.
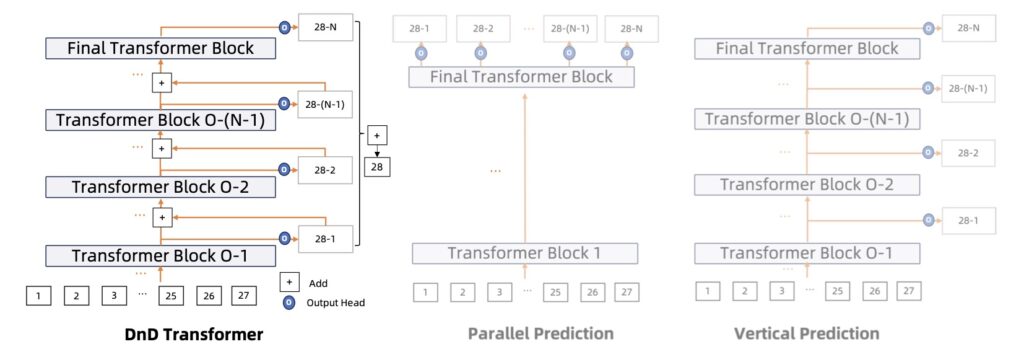
The experiments conducted using the DnD Transformer indicate impressive improvements in image quality, outpacing strong baselines such as LlamaGen. The model’s design allows it to predict more codes for an image efficiently, resulting in a superior output that resonates with the intricacies of visual data. This ability to generate high-fidelity images demonstrates the transformative potential of the DnD Transformer in the realm of AI-generated content.
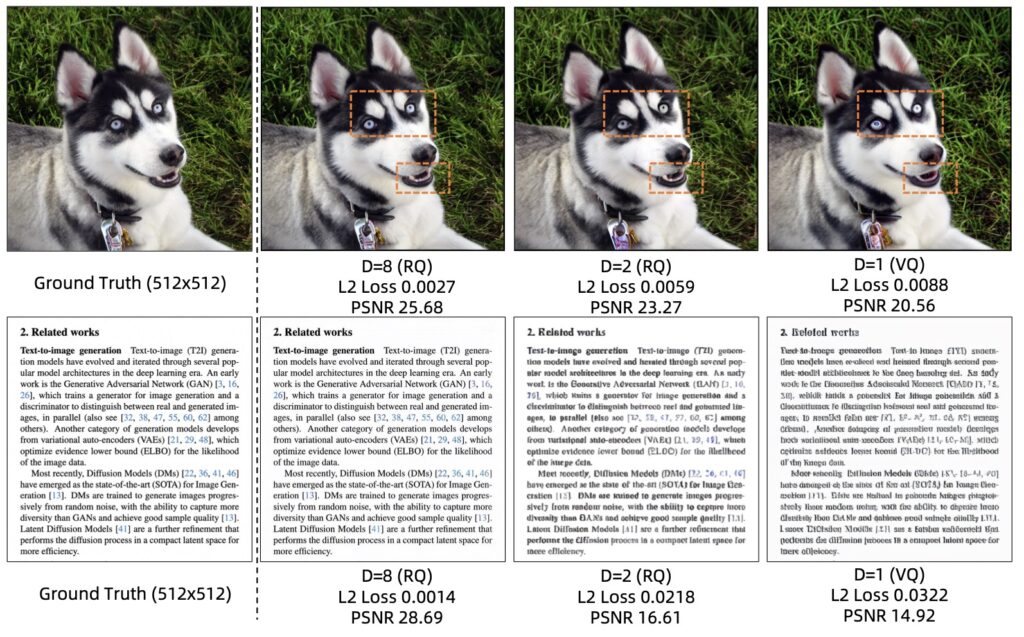
A particularly noteworthy aspect of the DnD Transformer is its emergent vision-language intelligence, which enables it to produce images rich in textual elements and graphical features without relying on explicit conditioning. This capability showcases the model’s understanding of multimodal information—a significant advancement not commonly found in traditional diffusion models. The potential applications for this technology are vast, ranging from creative industries to educational tools, where the ability to generate contextually relevant imagery is invaluable.
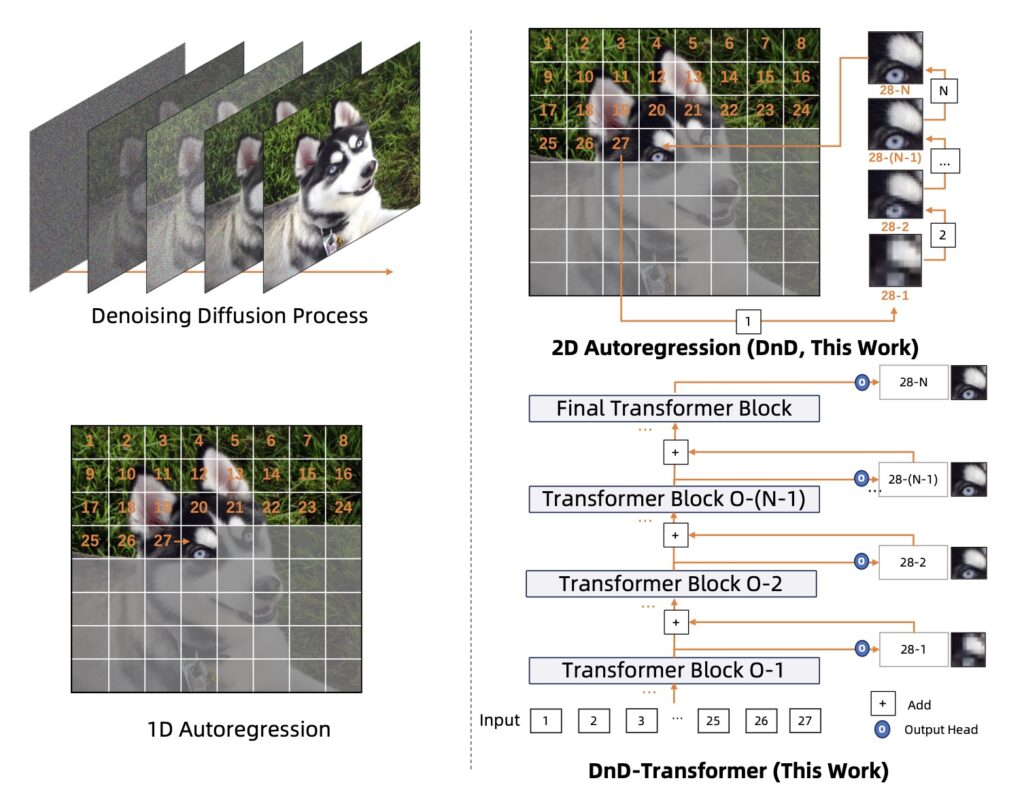
Looking ahead, the DnD Transformer’s innovative approach signifies a promising future for multimodal foundation models that can seamlessly integrate visual and textual information. As AI continues to evolve, the development of models like the DnD Transformer underscores the importance of creating efficient, high-quality generation techniques that address the complexities of modern data processing. By pushing the boundaries of what is possible in autoregressive image generation, researchers are paving the way for a new era of creativity and functionality in AI-driven applications.
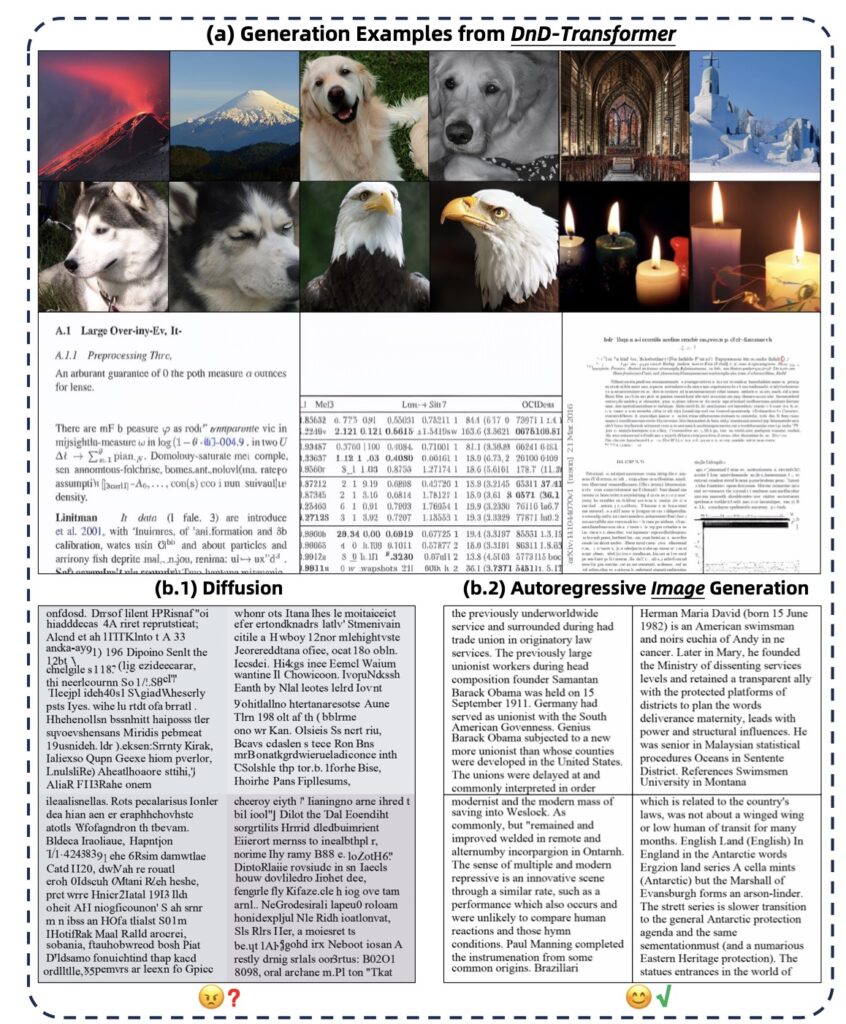
The introduction of the 2-Dimensional Autoregression Transformer marks a significant milestone in the quest for efficient and high-quality image generation. By effectively addressing the challenges of traditional autoregressive models and showcasing emergent vision-language intelligence, the DnD Transformer promises to be a game changer in the field of artificial intelligence. As we embrace this new era, the possibilities for innovative applications and enhanced user experiences are truly limitless.
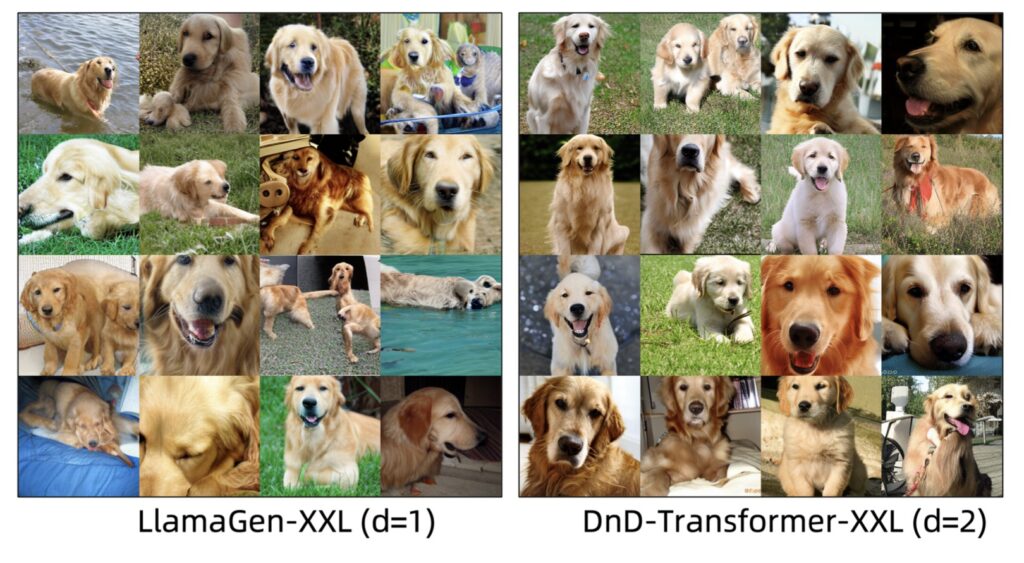