Solving Object Repetition in High-Resolution Image Generation
- AccDiffusion addresses the issue of object repetition in patch-wise higher-resolution image generation.
- The method uses patch-content-aware prompts and dilated sampling for better global consistency.
- AccDiffusion achieves high-resolution image generation without additional training.
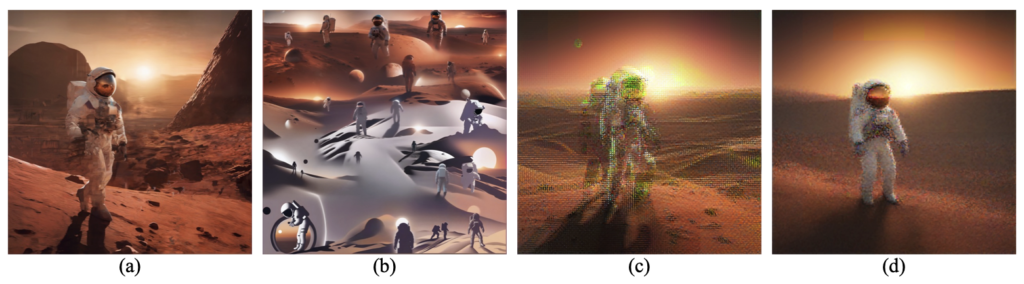
Generating high-resolution images using diffusion models has become increasingly popular, thanks to their exceptional generative abilities and wide range of applications. However, a persistent challenge in this field is the repetition of objects when generating images patch-wise, which compromises the quality and realism of the output. AccDiffusion, a new method proposed in recent research, tackles this issue effectively, offering a more accurate approach to high-resolution image generation without the need for additional training.
The Challenge of Object Repetition
Diffusion models like DDPM, DDIM, ADM, and LDMs have made significant strides in generative capabilities, yet they often require extensive computational resources and lengthy training periods. Stable diffusion models, for instance, demand substantial training time and resources, making it common to limit the resolution during training. However, the need for high-resolution images in real-world applications, such as advertising, remains high. This demand clashes with the limitations imposed by high training costs and computational demands.
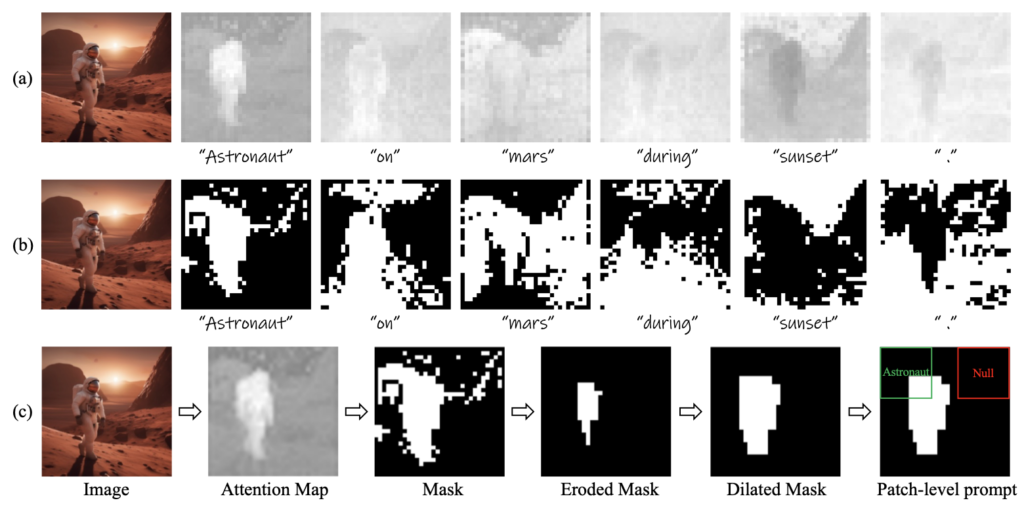
One of the critical issues in high-resolution image generation is the repetition of objects within patches when using identical text prompts. This repetition can severely detract from the overall quality and coherence of the generated image.
Introducing AccDiffusion
AccDiffusion presents a novel solution to the object repetition problem. Instead of using a single, uniform text prompt for the entire image, AccDiffusion decouples the image-content-aware prompt into a set of patch-content-aware prompts. Each of these prompts provides a precise description tailored to a specific image patch, thereby preventing repeated object generation and enhancing the detail and accuracy of each patch.
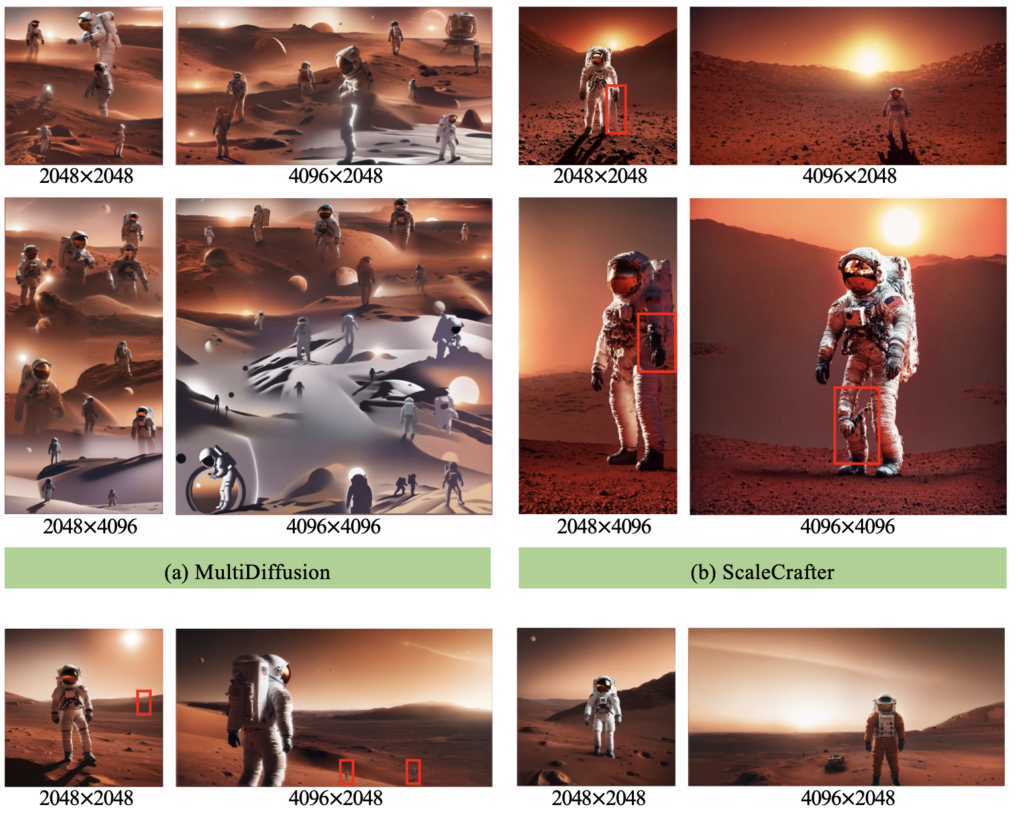
Additionally, AccDiffusion incorporates dilated sampling with window interaction. This technique improves global consistency across the entire image, ensuring that the high-resolution output is cohesive and free from disjointed patches.
Methodology and Innovations
- Patch-Content-Aware Prompts: By breaking down the general text prompt into more specific prompts for each patch, AccDiffusion avoids the pitfall of repeated objects. This approach allows for more accurate patch-wise denoising, resulting in higher fidelity images.
- Dilated Sampling with Window Interaction: This innovative sampling method enhances the global consistency of the generated image. It ensures that the patches interact with each other more effectively, creating a seamless and coherent high-resolution image.
- No Additional Training Required: One of the standout features of AccDiffusion is its ability to generate high-resolution images without the need for further training. This is particularly advantageous in reducing computational costs and time.
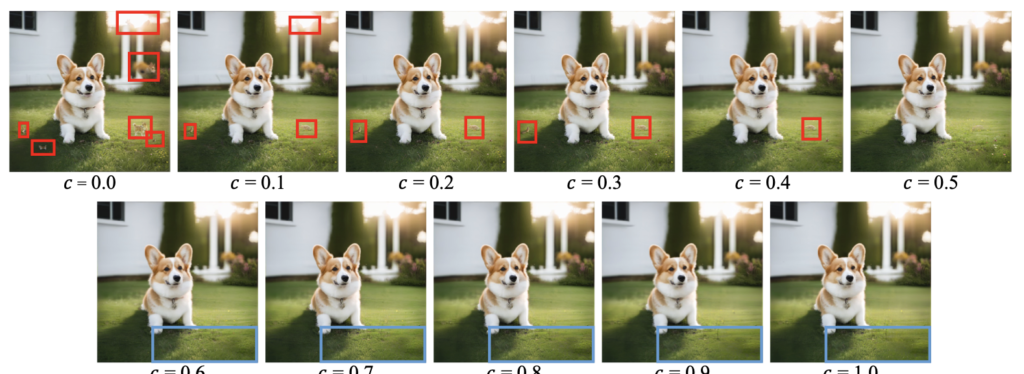
Experimental Results
Extensive experiments conducted on AccDiffusion demonstrate its effectiveness in generating high-resolution images. Both qualitative and quantitative results show that AccDiffusion successfully mitigates the issue of object repetition, producing images that are consistent and detailed across patches.
Comparison with Existing Methods:
- Success Rate: AccDiffusion achieves a higher success rate in maintaining background consistency and spatial appropriateness compared to traditional methods.
- Quality: The quality of images generated by AccDiffusion is superior, with fewer artifacts and better adherence to the desired visual attributes.
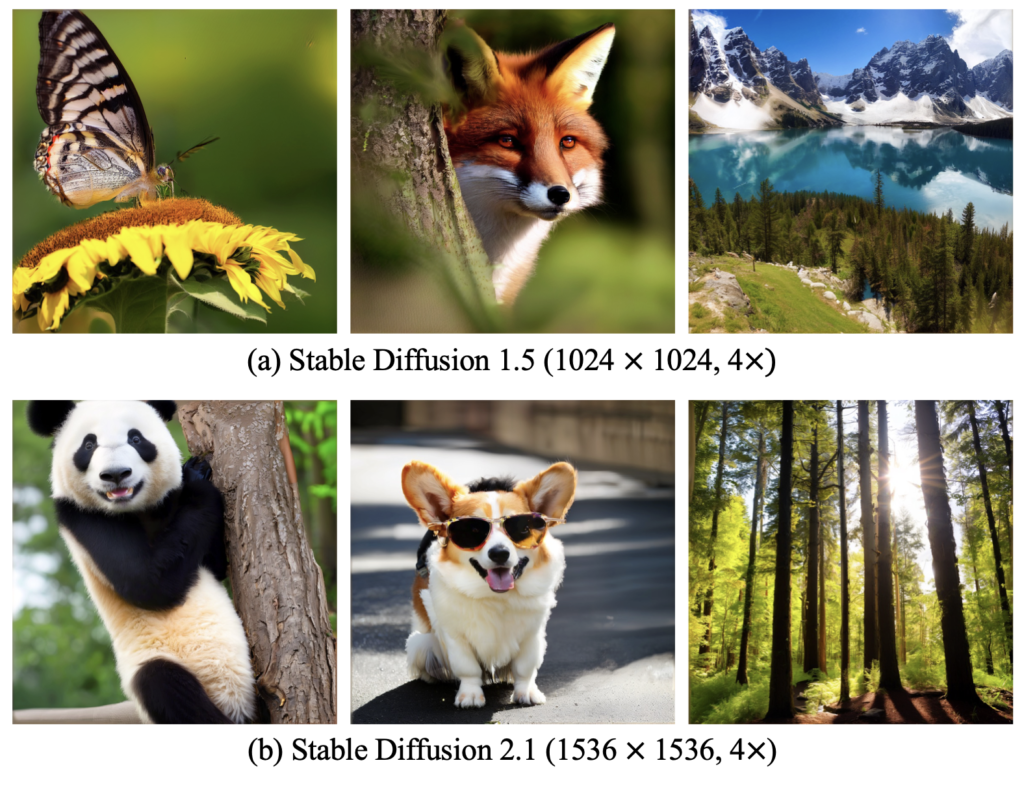
Limitations and Future Directions
While AccDiffusion represents a significant advancement in high-resolution image generation, it does have some limitations. For instance, it follows the DemoFusion pipeline, which can introduce inference latency due to progressive upscaling and overlapped patch-wise denoising. Additionally, the method’s reliance on pre-trained diffusion models means that the fidelity of the high-resolution images depends on these models’ prior knowledge.
Future Research:
- Non-Overlapped Patch-Wise Denoising: Exploring non-overlapped techniques could further improve efficiency and quality.
- Enhanced LDMs: Developing better latent diffusion models could mitigate the production of local irrational content in close-up images.
- Broader Applications: Future studies could investigate the application of AccDiffusion in various real-life scenarios, expanding its utility and impact.
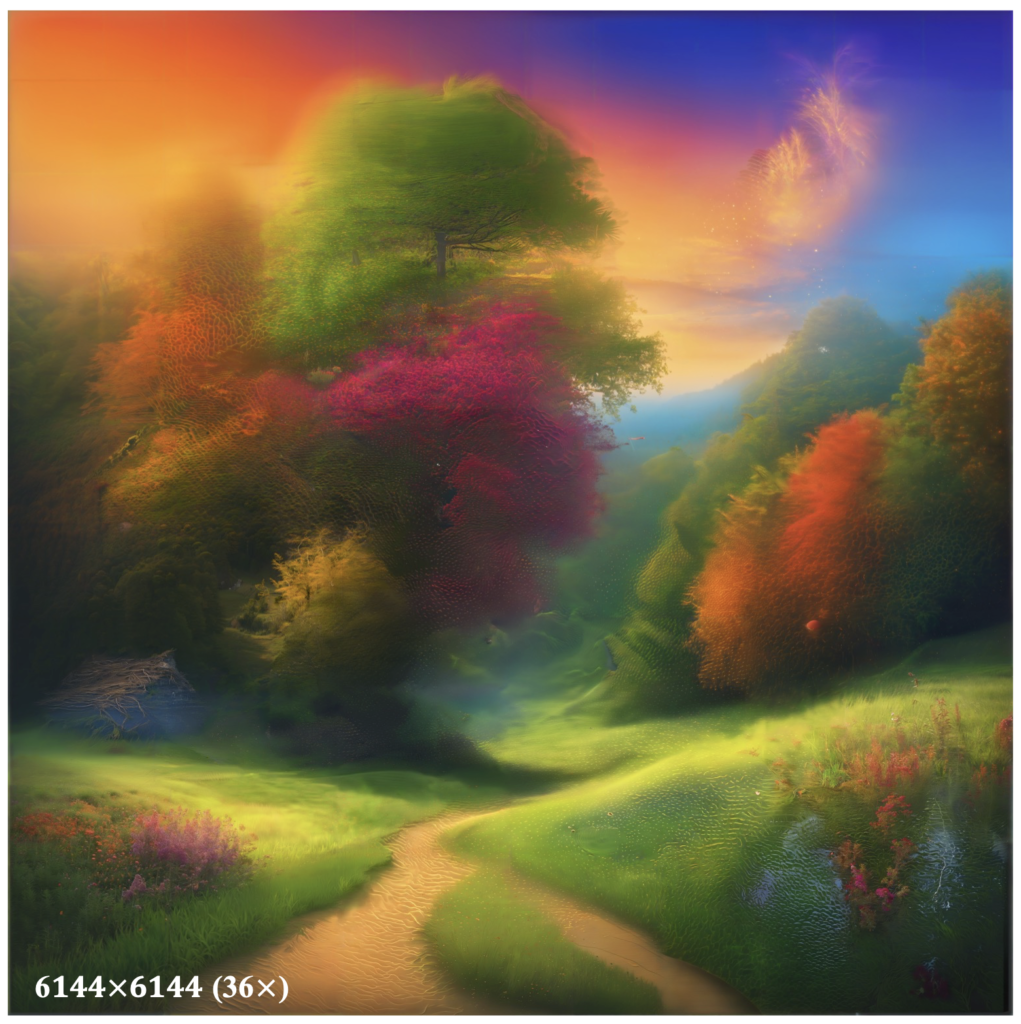
AccDiffusion offers a groundbreaking approach to high-resolution image generation, addressing the critical issue of object repetition in patch-wise generation. By utilizing patch-content-aware prompts and dilated sampling, it achieves superior global consistency and image quality without the need for additional training. As this method continues to evolve, it holds the promise of transforming high-resolution image generation, making it more accurate, efficient, and accessible for a wide range of applications.