New Model Enhances Image Synthesis Speed and Quality
- Unified Model: MLCM offers a single model for various sampling steps, improving efficiency.
- Progressive Training: Enhances inter-segment consistency, boosting image quality with fewer steps.
- Versatile Applications: Supports controllable generation, image style transfer, and Chinese-to-image generation.
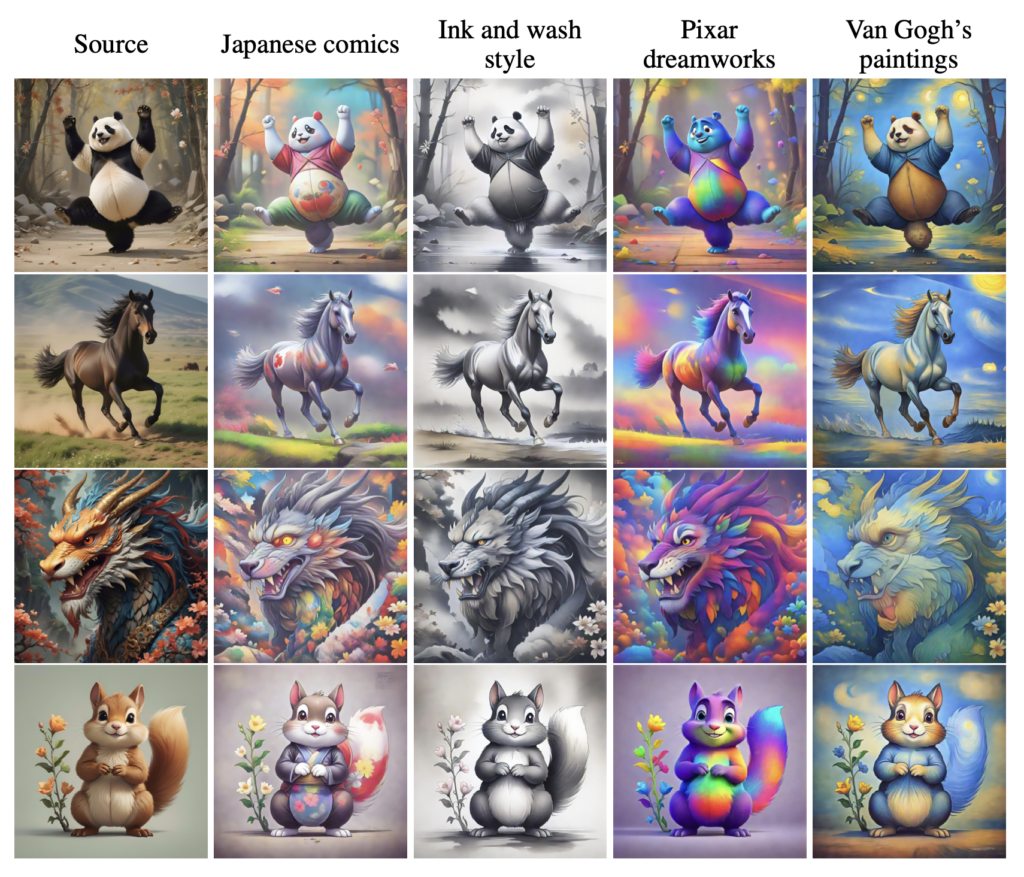
In the rapidly evolving field of generative modeling, diffusion models have become a cornerstone for creating high-fidelity images, videos, and audio. However, their computational demands, particularly during the sampling phase, have posed significant challenges. Enter the Multistep Latent Consistency Models (MLCMs), a groundbreaking approach designed to enhance both the speed and quality of image synthesis, setting new benchmarks in the industry.
Unified and Efficient Model
MLCMs are built on the innovative Multistep Consistency Distillation (MCD) strategy, which addresses the inefficiencies of previous models. Traditionally, large latent diffusion models (LDMs) required multiple denoising steps, making the process slow and resource-intensive. Existing methods often had to balance between needing multiple individual distilled models for different sampling budgets or compromising on image quality with limited sampling steps.
MLCMs eliminate these trade-offs by offering a unified model capable of performing across various sampling steps. This is achieved through a progressive training strategy that enhances inter-segment consistency, allowing the model to maintain high performance even with significantly reduced computational overhead. As a result, MLCMs can generate high-quality images with just 2-8 sampling steps, providing a substantial speedup compared to traditional methods.
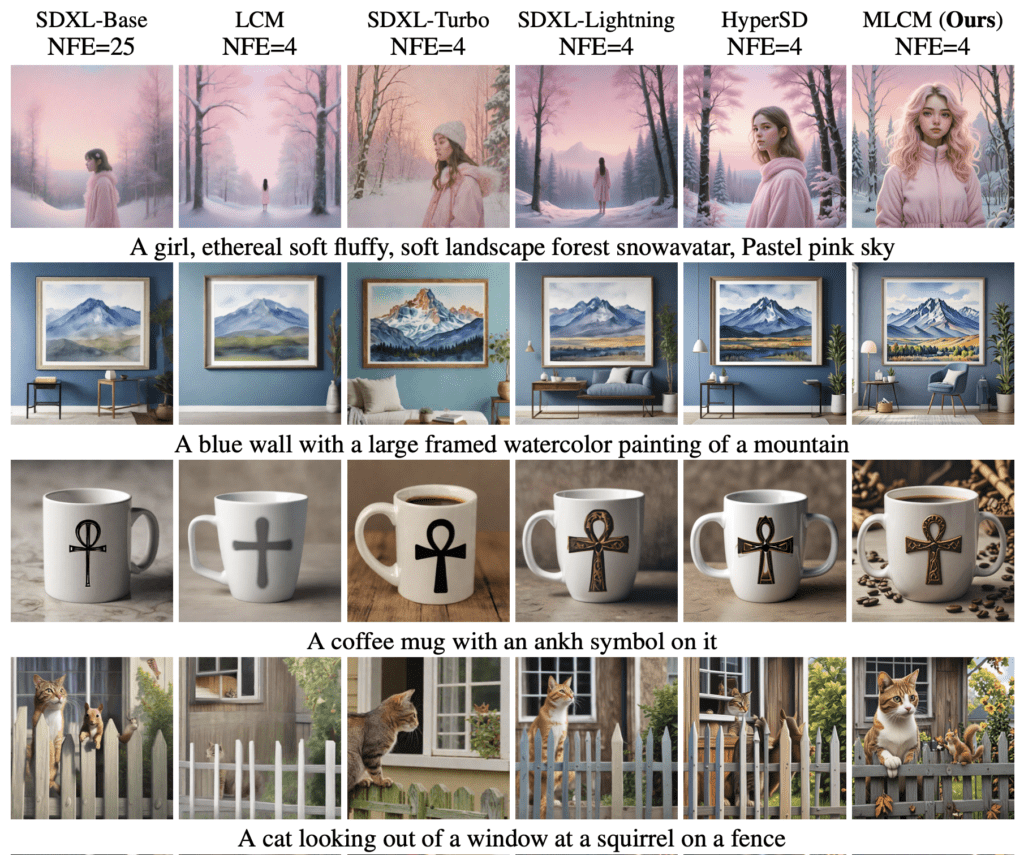
Enhanced Training Strategy
The core innovation in MLCMs lies in their training approach. By dividing the latent-space ordinary differential equation (ODE) trajectory into multiple segments, the model enforces consistency within each segment. This progressive training strategy ensures that the quality of the generated images remains high, even when fewer sampling steps are used.
Moreover, MLCMs leverage the states from the sampling trajectories of a teacher model as training data. This approach eliminates the need for high-quality text-image paired datasets, bridging the gap between training and inference. The model’s ability to integrate preference learning strategies further enhances visual quality and aesthetic appeal, making it a powerful tool for a wide range of applications.
Versatility and Practical Applications
MLCMs are not just about speed and efficiency; they also offer remarkable versatility. The model’s compatibility with controllable generation, image style transfer, and Chinese-to-image generation opens up new possibilities for creative professionals and enthusiasts alike.
For instance, in the domain of controllable generation, MLCMs allow users to specify certain attributes or styles, tailoring the output to meet specific requirements. This capability is particularly beneficial for applications in digital content creation and entertainment, where precise control over the generated content is crucial.
In the field of image style transfer, MLCMs enable the seamless transformation of images, applying different artistic styles or visual effects with ease. This feature can be leveraged in various creative industries, including graphic design and animation, to enhance visual storytelling.
Additionally, the model’s ability to handle Chinese-to-image generation highlights its potential in diverse linguistic and cultural contexts, expanding its usability beyond Western-centric applications.
MLCMs represent a significant leap forward in the field of generative modeling, offering a scalable, efficient, and high-quality solution for text-to-image synthesis. By eliminating the need for multiple denoising steps and leveraging a progressive training strategy, MLCMs set a new standard for speed and performance. Their versatility in applications such as controllable generation, image style transfer, and multilingual image synthesis further underscores their potential to transform various creative industries. As AI continues to evolve, innovations like MLCMs pave the way for more accessible, efficient, and sustainable models, bringing us closer to a future where high-quality image generation is available at the click of a button.