New Framework Enhances Multi-Step Decision-Making in Complex Environments
- Enhanced Learning from Experience: Agent Q integrates guided Monte Carlo Tree Search (MCTS) and a self-critique mechanism, enabling AI to learn effectively from both successful and failed interactions.
- Real-World Application Success: In the WebShop environment, Agent Q significantly outperforms existing models, boosting the LLaMA-3 70B model’s success rate from 18.6% to 95.4% with online search capabilities.
- Autonomous Decision-Making Leap: Agent Q demonstrates a substantial advancement in AI’s ability to perform complex, multi-step reasoning tasks autonomously, bridging the gap between theoretical AI capabilities and real-world application.
The development of large language models (LLMs) has propelled artificial intelligence (AI) into new realms of capability, particularly in the context of natural language processing and basic reasoning tasks. However, these models have traditionally faced significant limitations when applied to dynamic, multi-step environments where autonomous decision-making is required. The introduction of Agent Q, a groundbreaking framework for advanced AI reasoning and learning, marks a pivotal step forward in overcoming these challenges.
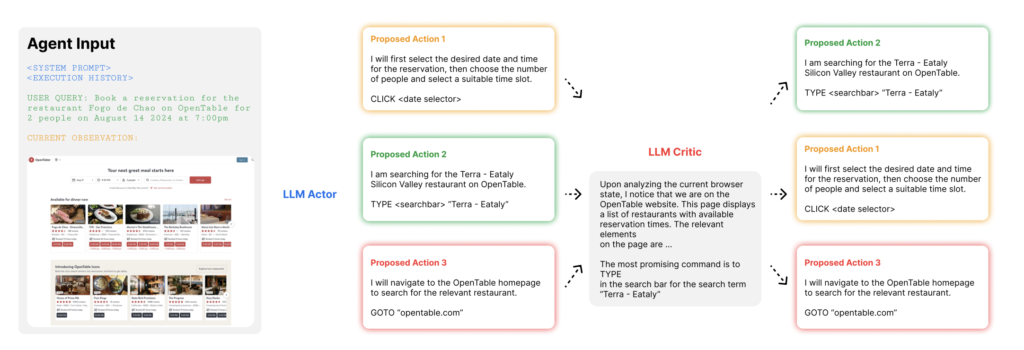
Agent Q: A New Era of Autonomous AI
Agent Q is designed to address the shortcomings of current LLMs in complex environments by combining several innovative techniques. Traditional supervised fine-tuning on static datasets often leads to suboptimal performance, particularly in scenarios that require dynamic decision-making. Agent Q bypasses these limitations through the use of guided Monte Carlo Tree Search (MCTS) and a self-critique mechanism. This approach allows the AI to learn from a broader spectrum of experiences—both successes and failures—thereby improving its generalization capabilities in complex, multi-step tasks.
One of the most impressive aspects of Agent Q is its application in the WebShop environment, a simulated e-commerce platform where the AI is tasked with navigating and making decisions in real-time. Traditional LLMs, including strong models like GPT-4, have struggled in such environments. For example, base models often fail to follow instructions or perform actions that require nuanced understanding and decision-making. However, with the integration of Agent Q, the LLaMA-3 70B model’s performance soared from an initial zero-shot success rate of 18.6% to an impressive 95.4% with the aid of online search capabilities.
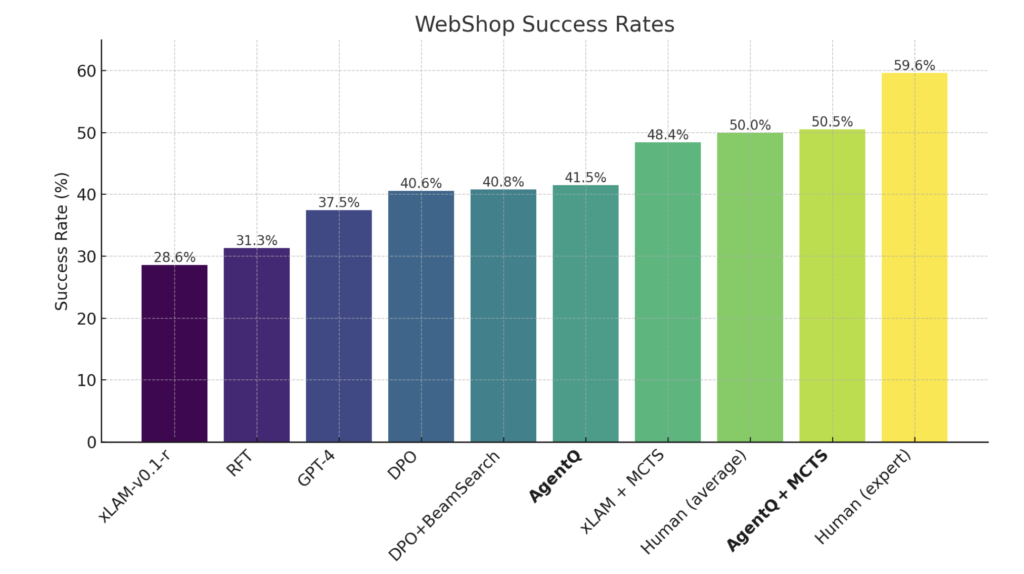
The Power of Agent Q’s Methodology
The key to Agent Q’s success lies in its AgentWrite pipeline, which decomposes complex tasks into manageable subtasks. This allows the AI to maintain coherence and precision across extended operations. Additionally, the use of Direct Preference Optimization (DPO)—a variant tailored for off-policy learning—enables the model to refine its decision-making process iteratively.
Through rigorous testing, Agent Q consistently outperformed behavior cloning and other reinforced fine-tuning baselines. In particular, the framework demonstrated its effectiveness in the WebShop environment, where it not only surpassed the performance of earlier models but also exceeded the average human success rate when equipped with the capability to perform online searches.
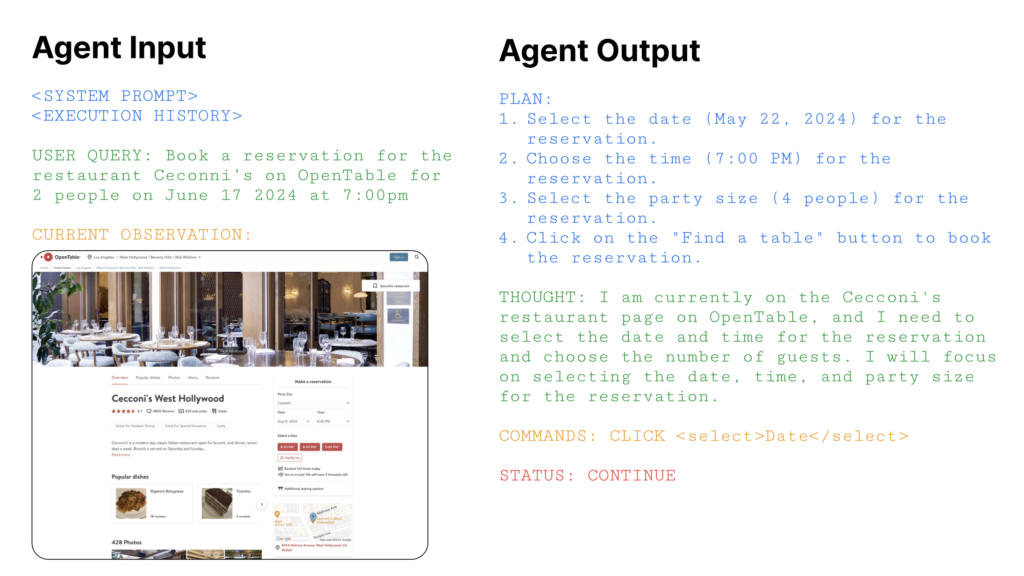
Implications and Future Prospects
The implications of Agent Q’s advancements extend far beyond the WebShop environment. The framework’s ability to enhance autonomous decision-making in AI has the potential to revolutionize various sectors, from e-commerce to complex problem-solving in fields like finance, healthcare, and logistics. The success of Agent Q in real-world booking scenarios, where it achieved a staggering 340% improvement in success rate, underscores the framework’s versatility and applicability across different domains.
Looking forward, the development team behind Agent Q has identified several areas for future research. These include expanding the AgentWrite framework to handle even longer and more complex tasks, refining the quality of generated long-output data, and improving inference efficiency to ensure that the model’s performance remains robust even as task complexity increases.
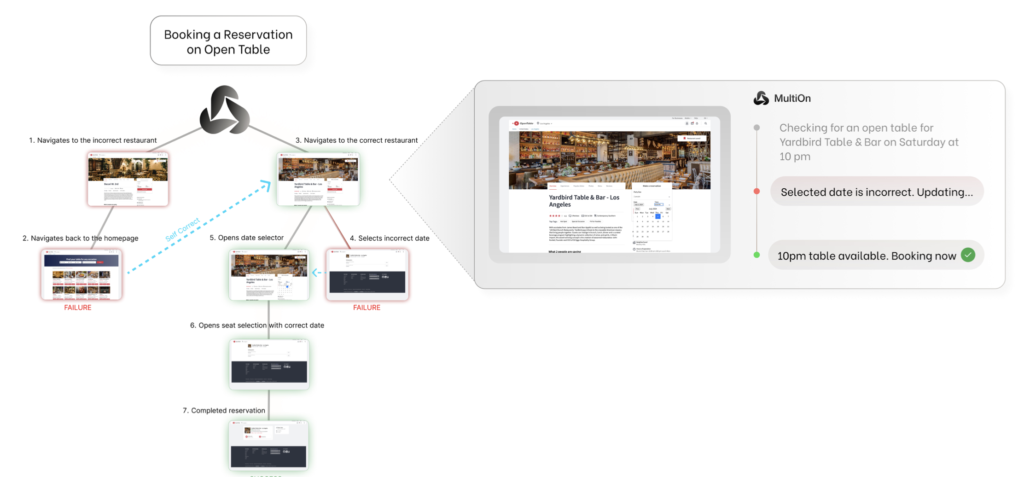
Agent Q represents a significant leap forward in the capabilities of autonomous AI agents. By integrating advanced reasoning techniques with real-time decision-making, this new framework bridges the gap between the theoretical potential of large language models and their practical application in dynamic environments. As AI continues to evolve, Agent Q’s innovations will likely pave the way for more sophisticated, reliable, and autonomous AI systems capable of tackling some of the most complex challenges in both digital and physical domains.