How Artificial Intelligence is Transforming Cancer Prognosis and Treatment
- AI-powered models using clinical notes and genomic data can predict cancer survival and treatment outcomes with unprecedented accuracy.
- The MSK-CHORD dataset integrates real-world data from over 24,000 cancer patients, uncovering new clinicogenomic relationships.
- Natural language processing (NLP) enables automated annotation of unstructured medical records, overcoming traditional data silos in oncology research.
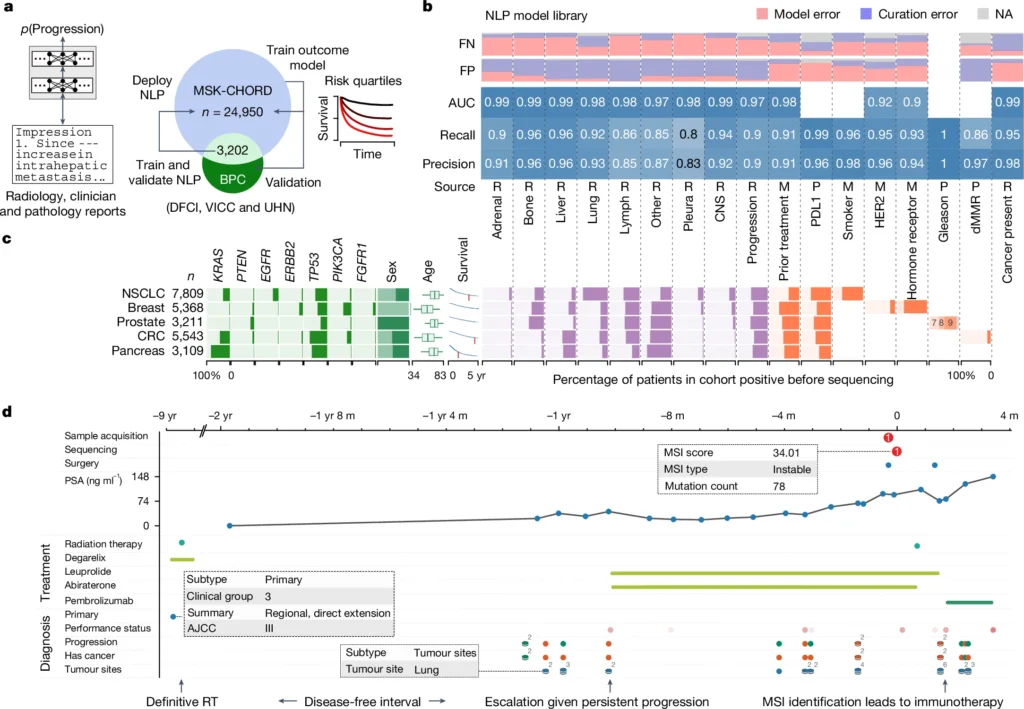
The integration of artificial intelligence (AI) into oncology is revolutionizing how cancer outcomes are predicted and treatments are personalized. By leveraging vast amounts of real-world data, including electronic health records (EHRs) and tumor-genome profiling, researchers are uncovering new insights into cancer progression, metastasis, and response to therapies. A groundbreaking study at Memorial Sloan Kettering Cancer Center (MSKCC) demonstrates how AI can transform unstructured clinical notes into actionable data, enabling more accurate predictions of survival and treatment outcomes. This article explores the potential of AI in oncology, the challenges it addresses, and the implications for future cancer care.
The Power of Real-World Data in Cancer Research
Cancer research has long relied on clinical trials and small, curated datasets. However, the digitization of health records and the growing availability of tumor DNA sequencing have opened new avenues for studying cancer outcomes. Real-world data (RWD) from EHRs, genomic profiling, and patient demographics offer a rich, untapped resource for understanding the complexities of cancer.
The MSK-CHORD dataset, developed by MSKCC, exemplifies the potential of RWD. This harmonized oncologic dataset includes information from 24,950 patients with various cancers, such as non-small-cell lung, breast, colorectal, prostate, and pancreatic cancers. By combining structured data (e.g., medication records, tumor registries) with unstructured text (e.g., radiology and pathology reports), MSK-CHORD provides a comprehensive view of cancer trajectories.
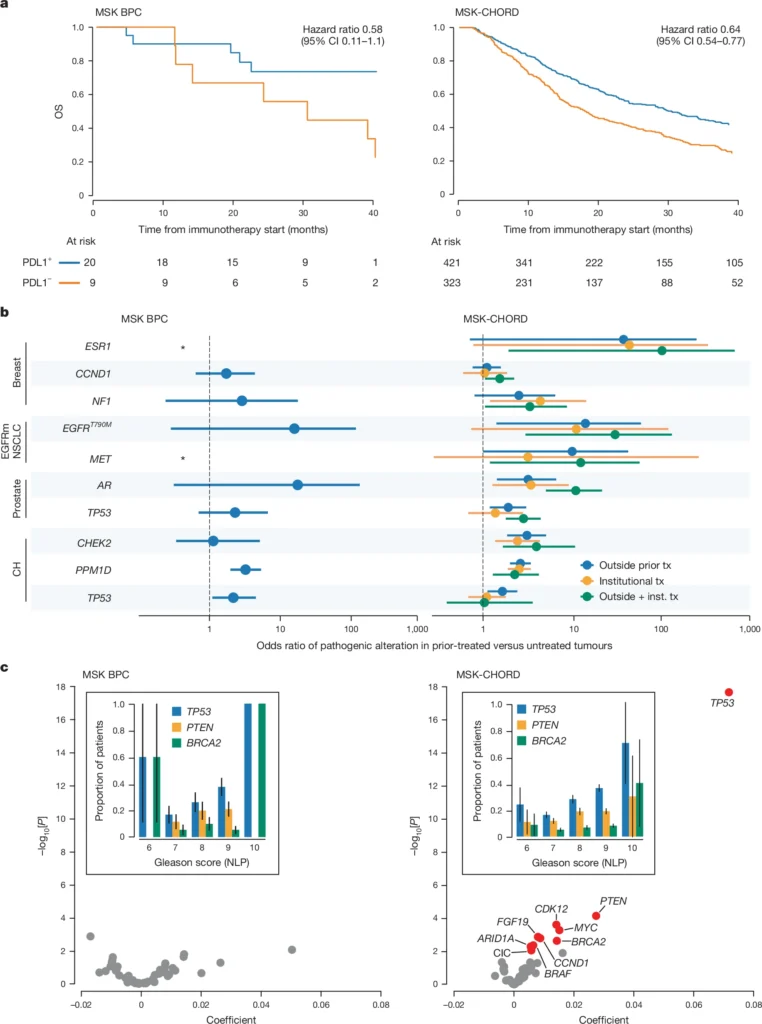
AI and Natural Language Processing: Breaking Down Data Silos
One of the biggest challenges in utilizing RWD is the unstructured nature of clinical notes, radiology reports, and pathology records. Traditionally, extracting meaningful insights from these free-text documents required manual annotation, a time-consuming and error-prone process.
AI, particularly natural language processing (NLP), has changed the game. Advanced transformer-based models, such as Clinical-Longformer, can automatically annotate unstructured medical records, identifying key features like disease sites, genomic mutations, and treatment responses. In the MSK-CHORD study, NLP was used to annotate over 705,000 radiology reports, uncovering predictors of metastasis and survival. For example, the study identified a relationship between the SETD2 mutation and lower metastatic potential in lung adenocarcinoma patients treated with immunotherapy.
Predicting Cancer Outcomes with AI Models
AI models trained on the MSK-CHORD dataset have demonstrated remarkable accuracy in predicting cancer outcomes. By integrating multimodal data—clinical notes, genomic profiles, and tumor registries—these models outperform traditional methods that rely solely on genomic data or cancer staging.
For instance, machine learning models trained on MSK-CHORD data were able to predict overall survival (OS) more effectively than models based on genomic data alone. The study also used AI to analyze time to metastasis, identifying genomic alterations associated with metastatic potential. These insights can guide treatment decisions, such as selecting patients who are more likely to benefit from immunotherapy.
Overcoming Barriers to Integrative Cancer Research
Despite its promise, the integration of RWD in cancer research faces significant hurdles. Data silos—where genomic, radiology, and EHR data are stored separately—limit the ability to perform comprehensive analyses. The MSK-CHORD study addresses this challenge by harmonizing data from multiple sources, creating a unified dataset that enables cross-disciplinary research.
Moreover, the study highlights the feasibility of automated annotation, reducing reliance on manual data extraction. This approach not only accelerates research but also ensures scalability, allowing researchers to analyze larger datasets and uncover patterns that would be missed in smaller cohorts.
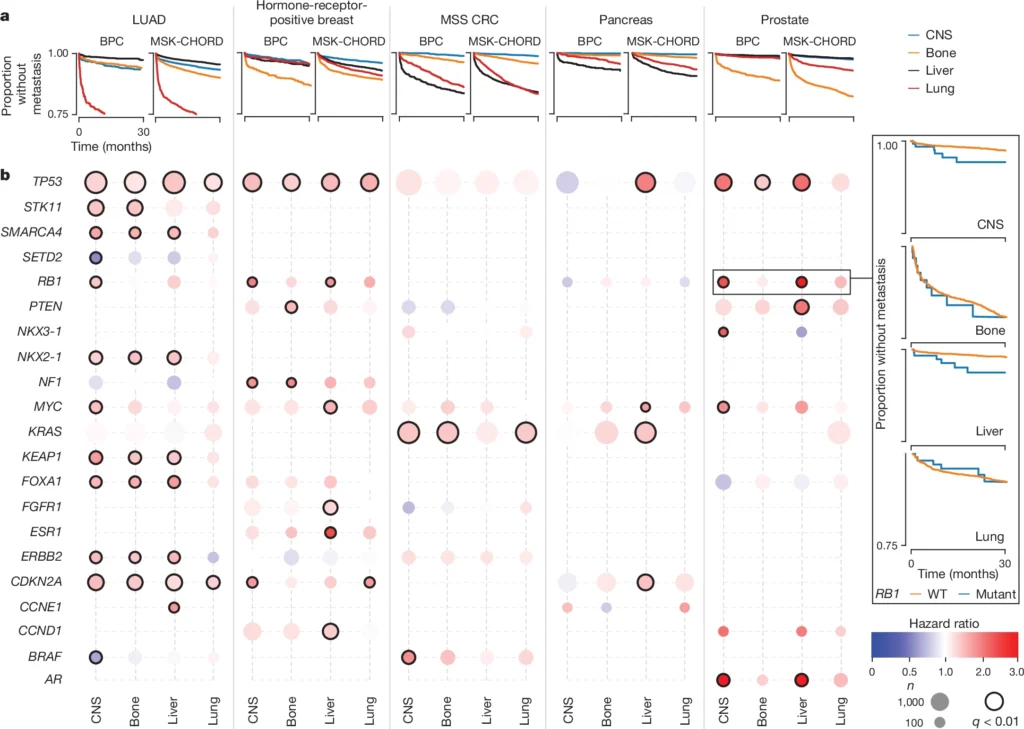
Implications for Cancer Care and Beyond
The findings from the MSK-CHORD study have far-reaching implications for cancer care. By leveraging AI to predict outcomes and identify genomic features associated with treatment response, clinicians can personalize therapies to individual patients. For example, patients with specific mutations or disease sites may be prioritized for certain treatments, improving survival rates and quality of life.
Beyond oncology, the integration of AI and RWD has the potential to transform other areas of medicine. From predicting hospital readmissions to identifying risk factors for chronic diseases, the applications of AI in healthcare are vast and growing.
The Future of AI in Oncology
As AI continues to evolve, its role in oncology will only expand. Future research may focus on integrating additional data modalities, such as imaging and histopathology, to create even more comprehensive models. Collaborative efforts between hospitals, academic institutions, and commercial entities will be essential to overcome data silos and ensure the ethical use of patient data.
The MSK-CHORD study is a testament to the power of AI in advancing cancer research. By combining cutting-edge technology with real-world data, researchers are paving the way for a new era of precision oncology—one where cancer outcomes are not just predicted but actively improved.
The integration of AI and RWD represents a paradigm shift in cancer care. By harnessing the power of unstructured clinical notes and genomic data, researchers can uncover new insights into cancer progression and treatment. The MSK-CHORD dataset is a shining example of how AI can break down barriers in oncology research, offering hope for better outcomes and a brighter future for patients worldwide.